Life
Are You Ready for the AI Revolution? Prepare for 2027!
The AI Revolution by 2027 is closer than you think. Learn how to prepare for AI’s explosive growth and its impact on the future.
Published
1 month agoon
By
AIinAsia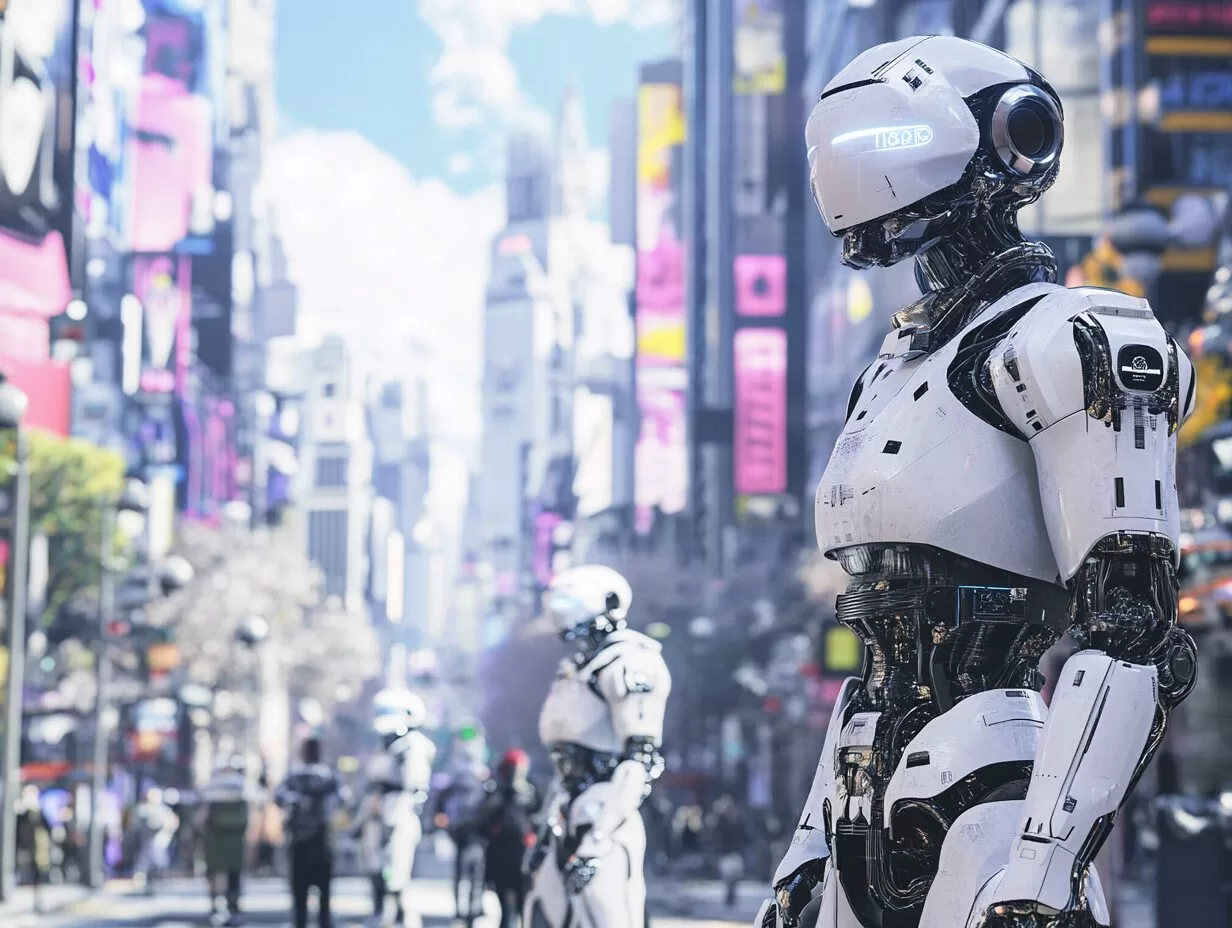
TL;DR:
- AI is developing at an unprecedented speed, with models becoming 5X better each year.
- By 2027, AI could reach the intelligence level of top PhDs, leading to an intelligence explosion.
- The AI industrial revolution will bring rapid advancements, potentially disrupting jobs and economies.
Imagine waking up one day to find AI has revolutionised the world overnight. Sound far-fetched? It’s closer than you think. Not many people realise how quickly AI is developing. By the time you finish this article, you’ll not only understand the AI revolution’s trajectory but also know how to prepare for it. And when AI superintelligence emerges in 2027? You’ll be ready.
From Smart to Superhuman: AI’s Explosive Growth by 2027
Most bullets travel faster than the speed of sound, making them invisible to the naked eye. This is similar to the speed of AI development—so fast that most people won’t see it coming.
According to Leopold in his essay “Situational Awareness”, ChatGPT models are evolving at the speed of ~0.5 OOMs/year. In other words, AI is becoming 5X better each year, compounding over time.
This means that by 2027/28, ChatGPT and other LLMs—large Language Models—will be as smart as the top PhDs worldwide.
“Over and over again, year after year, skeptics have claimed ‘deep learning won’t be able to do X’ and have been quickly proven wrong. If there’s one lesson we’ve learned from the past decade of AI, it’s that you should never bet against deep learning.”
— Leopold Aschenbrenner
Advertisement
Once AIs reach the level of knowledge of top PhDs, they won’t just know as much as one smart PhD; they will know as much as one million PhDs combined.
Just picture that.
At the moment, perhaps a few thousand smart people are researching and developing AI. However, once AI becomes smarter than those developing it, it will be able to improve itself at a much faster rate than human researchers can.
Once AI systems reach that level, they can automate AI research itself, triggering powerful feedback loops that could accelerate development so rapidly that we won’t be able to catch up.
AI is developing this fast for three main reasons:
- The amount of computing power dedicated to AI is continually increasing. Companies like Nvidia produce billions of dollars worth of AI chips every year.
- AI algorithms are becoming better and faster. In less than two years, ChatGPT became 1,000 times more efficient, with AI companies investing billions to improve these algorithms.
- Improvements to algorithms include enhanced Reinforcement Learning from Human Feedback (RLHF) and better Chain of Thought.
By 2027, don’t be surprised if your new “smart” fridge denies you that midnight snack because it has calculated a better diet plan for you.
2028’s World: When AI = 1 Million Human Scientists
AI progress will not stop at the human level.
Hundreds of millions of AGIs will automate AI research, compressing a decade of algorithmic progress into less than or equal to 1 year.
We will go very fast from college degree-level AIs (what we have at the time of writing this article) to superhuman AI systems.
The power of AI superintelligence will surprise almost everyone, as its growth will compound extremely fast.
As Irving John Good predicted, AI will explode:
“An ultraintelligent machine could design even better machines; there would then unquestionably be an ‘intelligence explosion,’ and the intelligence of man would be left far behind. Thus the first ultraintelligent machine is the last invention that man need ever make.”
— Irving John Good, 1965
While AlphaGo may seem focused solely on a game, it illustrates that AIs can surpass human abilities in potentially any field.
Soon, ChatGPT and other LLMs will be vastly smarter than us, akin to an AI earning a PhD while humans are still at the elementary school level.
AIs will literally leave humans in the dust!
This is the full-blown AGI — Artificial General Intelligence — future that will surprise most people, make many jobs redundant, and disrupt businesses that fail to adapt.
And it’s not that far away.
When Will AGI Happen?
At the current pace, we can expect to have AGI in just four years.
Once achieved, AGI will automate AI research itself, leading to an extreme explosion in superintelligence. This is akin to having millions of smart human researchers working 24/7.
Just think about it: once we achieve AGI, the massive GPU data centres we are building will host millions of AGIs running simultaneously.
This will be equivalent to 100 million human-equivalent intelligences operating at speeds ten times faster than humans.
These AIs will work on significant human problems, such as curing diseases, conducting longevity research, and solving various other issues, all while improving themselves.
They will also be replacing human jobs. Lots of them.
More than ever, you need to adapt and be at the forefront of these developments.
This is no longer science fiction; it’s something that will happen soon.
The Power of Superintelligence
The super-fast development of superintelligent AI systems by the end of this decade is something we must confront.
These AIs will be both quantitatively and qualitatively superhuman, processing information at unprecedented speeds and generating new solutions beyond human comprehension.
It’s possible that in just 10 years, AI will be so complex and superintelligent that we won’t even be able to understand how it works.
We will be like kindergarteners trying to grasp a PhD in mathematics.
It is better to position yourself to benefit from the dramatic advancements in robotics, scientific progress, and economic growth.
Economic growth rates could exceed 30% per year, similar to those seen during the Industrial Revolution.
Superintelligence will bring huge advantages to those at the forefront, disrupt governments, and transform both society and the economy.
But buckle up, because the leap to AI superintelligence will create a very volatile period in our world. This period could be one of the most dangerous in history, marked by rapid changes and little time for critical decisions.
You must brace for impact. Perhaps everything will go smoothly; perhaps not. Either way, please prepare yourself.
If we’re lucky, AI might just solve all our problems. If not, well… at least we’ll have self-driving cars to take us to the unemployment line.
Profit from the AI Industrial Revolution
To prepare for this AI industrial revolution, it’s important to position your investments to benefit from AI and technology. Many have people have most of my portfolio allocated to cryptocurrencies, tech stocks, robotics, and tech ETFs.
To fuel the AI revolution, there will be enormous demand for hardware; therefore, being a shareholder in GPU-related companies can’t go wrong. However, if you want to diversify further, go for the ETFs.
Over the coming few years, we will need 10 to 100 times more computing power and techno-optimists, believe there’s a very high chance that AI progress will benefit humanity.
Still, given the ultra-fast AI developments toward AGI and superintelligence, most people will be caught by surprise.
The key is to position yourself to understand and invest in these technologies — this is exactly why we write about and share our knowledge of AI, investing, tech, and personal growth.
We can’t be passive in a world evolving at lightning speed. Whether you like it or not — AI progress is coming — and you need to prepare actively.
Comment and Share:
Are you excited or worried about the AI revolution? Share your thoughts and experiences with AI and AGI technologies. Don’t forget to subscribe for updates on AI and AGI developments and comment on the article below.
- You may also like:
To learn more about the AI revolution, tap here.
Author
Discover more from AIinASIA
Subscribe to get the latest posts sent to your email.
You may like
-
Meet Asia’s Weirdest Robots: The Future is Stranger Than Fiction!
-
AI Unleashed: Discover the Power of Claude AI
-
AI Unleashed: Discover the Power of ChatGPT
-
Meet Tesla’s Optimus: The Humanoid Robot That Can Do Anything
-
The Future of AI: Expert Insights and Emerging Trends
-
OpenAI Expands to Singapore: A New Hub for AI Innovation in Asia
Life
Why AI Skills Will Be Non-Negotiable in 2025
AI skills will be non-negotiable in 2025, transforming industries and reshaping job roles. Learn essential AI skills and their applications to future-proof your career.
Published
3 days agoon
November 12, 2024By
AIinAsia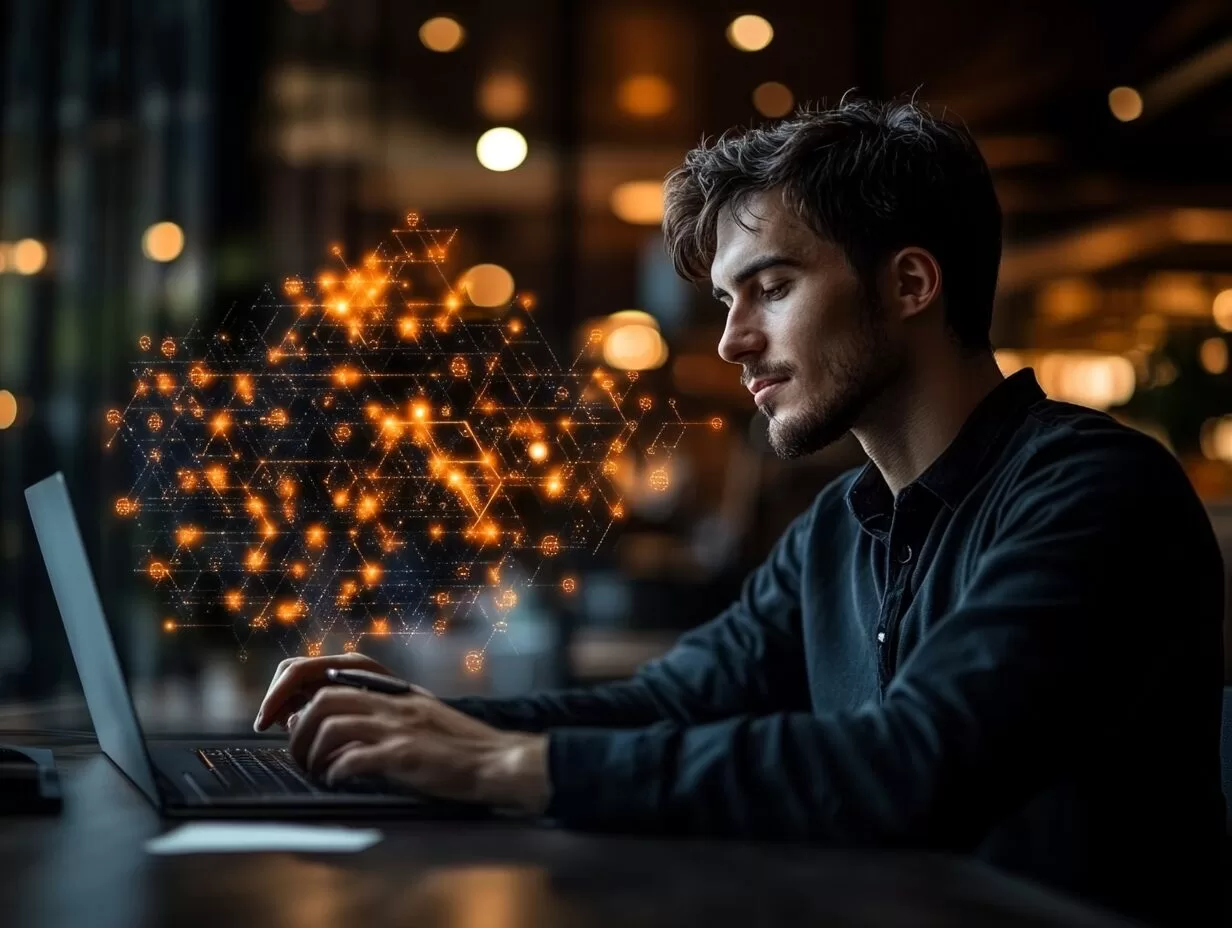
TL/DR: Understanding and developing AI skills in 2025 will be crucial for staying competitive in the future job market.
- AI skills are becoming essential for professionals across all industries by 2025.
- Key AI skills include data literacy, prompt engineering, machine learning fundamentals, AI ethics, and workflow automation.
- Professionals can start learning AI skills through online platforms, local certification programs, meetups, and on-the-job training.
Artificial intelligence is no longer just a buzzword—it’s a transformative force reshaping industries, redefining job roles, and creating entirely new fields of work. AI’s impact is undeniable across a wide spectrum of sectors, from finance and healthcare to marketing and logistics. In this rapidly evolving environment, AI skills have shifted from being a “nice-to-have” to an essential component of a future-ready career.
For professionals in every field, staying relevant means understanding AI and developing skills that allow them to leverage its potential effectively. The AI skill set encompasses more than just technical programming; it involves understanding data, ethical considerations, and integrating AI into daily tasks. In 2025, gaining proficiency in AI won’t just enhance your career prospects—it will future-proof them.
Here’s a comprehensive guide to the critical AI skills you need, their applications, and how to start building your AI knowledge.
The New Essential Skills for 2025
To thrive in a world where AI underpins most industries, it’s crucial to focus on a range of AI-related skills. While not everyone needs to be an AI developer, there are several accessible, practical skills that professionals across roles can benefit from:
Data Literacy and Visualisation
AI’s power lies in its ability to process and interpret massive volumes of data. Professionals who understand how to collect, analyse, and interpret data are better equipped to work alongside AI. Data literacy extends beyond basic analytics—it includes knowing how to use data visualisation tools like Tableau or Power BI to present AI-generated insights in a meaningful way.
Prompt Engineering
With the proliferation of language models, from ChatGPT to other AI-driven assistants, prompt engineering has become a unique and increasingly valuable skill. Knowing how to craft effective prompts can dramatically improve the quality of AI-generated outputs, allowing professionals to use AI more effectively in roles like content creation, customer support, and market analysis.
This prompt is designed to extract valuable insights from AI models, helping professionals understand the future landscape of AI in healthcare and how it might impact their roles:
Prompt: “Generate a list of top 10 AI trends in 2025 and their potential impact on the healthcare industry.”
Machine Learning Fundamentals
While deep expertise in machine learning isn’t necessary for most professionals, a foundational understanding is beneficial. Concepts like supervised vs. unsupervised learning, predictive analytics, and data training empower professionals to engage with AI projects and collaborate with data science teams more effectively.
AI Ethics, Privacy, and Compliance
As AI’s capabilities grow, so do ethical considerations around its use. Knowing how to navigate privacy concerns, data biases, and ethical decision-making is essential, especially for professionals handling sensitive data or working in regulated industries. Familiarity with data protection regulations, such as GDPR in Europe or PDPA in Singapore, is crucial for responsible AI deployment.
Workflow Automation and RPA (Robotic Process Automation)
Automation is reshaping roles across all sectors. RPA tools like UiPath and Blue Prism enable professionals to automate routine tasks, improving efficiency and allowing them to focus on strategic work. Mastery of RPA and other automation platforms can make professionals indispensable, as they help organisations streamline processes and reduce operational costs.
AI-Enhanced Decision-Making
AI is at its most valuable when it augments human decision-making. Developing skills in interpreting AI-driven insights and understanding their implications in a business context is crucial. This includes knowing when to trust AI predictions, how to assess accuracy, and integrating AI into strategic planning and forecasting.
Examples of AI Skill Applications Across Industries
AI’s influence is not confined to the tech sector; it’s reshaping roles across all industries. Here’s a look at how AI skills are transforming various fields and what it means for professionals:
Marketing and Sales
AI has become central to personalising customer experiences and optimising campaigns. Marketers use AI-driven analytics to segment audiences and tailor content, increasing engagement and conversion rates. Sales teams leverage AI to score leads, predict customer needs, and refine sales strategies. Familiarity with AI tools like Salesforce’s Einstein or HubSpot’s AI recommendations is becoming a must for anyone in these roles.
Healthcare and Life Sciences
AI is revolutionising patient care and diagnostics. Professionals in these fields are increasingly relying on AI to interpret medical data, identify risk factors, and support diagnostic processes. For example, AI systems can detect anomalies in imaging scans, giving healthcare workers valuable support in diagnosing diseases early. Knowledge of AI-powered diagnostic tools and an understanding of medical data privacy regulations (e.g., HIPAA in the U.S.) are essential for healthcare professionals.
Finance and Banking
AI is being used to detect fraud, assess credit risk, and automate trading processes. Financial professionals are expected to understand how to interpret AI-driven risk assessments and leverage predictive analytics for real-time decision-making. Skills in Python for financial data analysis, machine learning for predictive insights, and an understanding of ethical data use in finance are key.
Customer Service and Support
AI is reshaping customer service with chatbots, virtual assistants, and sentiment analysis. Prompt engineering skills help support agents get the most out of AI tools, crafting responses that align with customer needs. AI can analyse customer sentiment in real time, enabling support agents to address concerns proactively and enhance customer satisfaction.
Human Resources and Recruitment
HR professionals are turning to AI for more efficient recruitment and employee engagement. AI can screen resumes, identify high-potential candidates, and even monitor employee satisfaction through sentiment analysis. Understanding AI-driven recruitment platforms like Lever and Workday and being aware of data privacy in employee data handling can significantly boost an HR professional’s effectiveness.
How to Start Learning AI Skills in Asia
With AI skills in such high demand, the opportunities to acquire them are more accessible than ever. Here are some steps for getting started, with a focus on resources and programs in Asia:
Online Learning Platforms
Coursera, edX, and Udacity provide AI courses that range from beginner to advanced levels, often in partnership with universities like the National University of Singapore, Tsinghua University, and Indian Institute of Technology. These courses cover machine learning basics, data analytics, and AI ethics, providing a comprehensive starting point for learners.
Local Certification Programs and Workshops
Several organisations in Asia offer in-person workshops and certification programs. The SQREEM Symposium in Singapore, for example, offers hands-on training in AI tools and automation. These sessions are invaluable for gaining practical, regionally relevant experience and connecting with industry professionals.
Meetups and Professional Networks
AI-focused meetups and professional networks, like those on Meetup or LinkedIn, offer valuable opportunities for community learning. Being part of groups dedicated to AI allows professionals to discuss emerging trends, exchange tips, and stay motivated.
On-the-Job Training and Upskilling Programs
Many organisations are now providing in-house AI training for employees, recognising the importance of AI proficiency. Professionals should seek out these opportunities and take advantage of company-sponsored courses to develop skills directly applicable to their roles.
Self-Led Exploration
AI tools are often freely accessible or available at low cost, making it easier for professionals to experiment. Tools like ChatGPT, Google Colab, and data visualisation platforms (e.g., Power BI) allow individuals to apply their learning in real-world contexts. Building side projects or taking on small AI-driven tasks can boost confidence and practical skills.
The Future of Work: AI’s Role in Career Development
Looking forward, the impact of AI on career development will only grow. In 2025 and beyond, professionals will need not only to understand AI tools but also to adapt to an environment where AI is an integral part of strategic planning and operations. Here’s how AI will influence the future of work:
Increased Emphasis on Hybrid Skills
As AI handles routine tasks, human skills like creativity, problem-solving, and critical thinking will be in greater demand. Professionals who blend technical AI skills with strong interpersonal abilities will stand out.
Lifelong Learning Culture with AI Education
The AI landscape evolves rapidly, and continuous learning will be crucial to stay current. Professionals should prioritise ongoing skill development to keep up with new AI technologies, tools, and industry applications.
Greater Collaboration with AI Systems
In 2025, most jobs will involve working closely with AI systems. Professionals should embrace this collaboration, seeing AI as a partner that enhances their abilities rather than a competitor.
Building a Future-Proof Career in the Age of AI
As 2025 unfolds, AI will continue to redefine industries and reshape the professional landscape. Building a future-proof career means investing in AI skills that make you adaptable, resourceful, and prepared for the opportunities AI brings. Whether it’s data literacy, machine learning, or workflow automation, AI skills equip you to drive value in your role, make informed decisions, and remain competitive.
The journey to AI fluency may seem daunting, but even small steps can lead to substantial rewards. Start by mastering one skill, such as prompt engineering or data literacy, and gradually expand your expertise. In the age of AI, adaptability and curiosity are your most valuable assets. Embrace them, and you’ll be well-positioned to not only survive but thrive in the workforce of the future.
Join the Conversation
What AI skills are you most excited to learn and why? Share your thoughts and experiences below, and don’t forget to subscribe for updates on AI and AGI developments. Subscribe here to stay informed and engaged!
You may also like:
- Work Smarter, Not Harder: AI Hacks for Asian Professionals
- Mastering the Art of AI Prompts for Everyday Requests
- Reaching Today’s Consumers – How AI Enhances Digital Marketing
- Or try ChatGPT by tapping here.
Author
Discover more from AIinASIA
Subscribe to get the latest posts sent to your email.
Learning
Mastering the Art of AI Prompts for Everyday Requests
Mastering the art of AI prompts can transform general responses into personalised, useful answers. Learn how to refine prompts step-by-step for tailored AI outputs.
Published
7 days agoon
November 8, 2024By
AIinAsia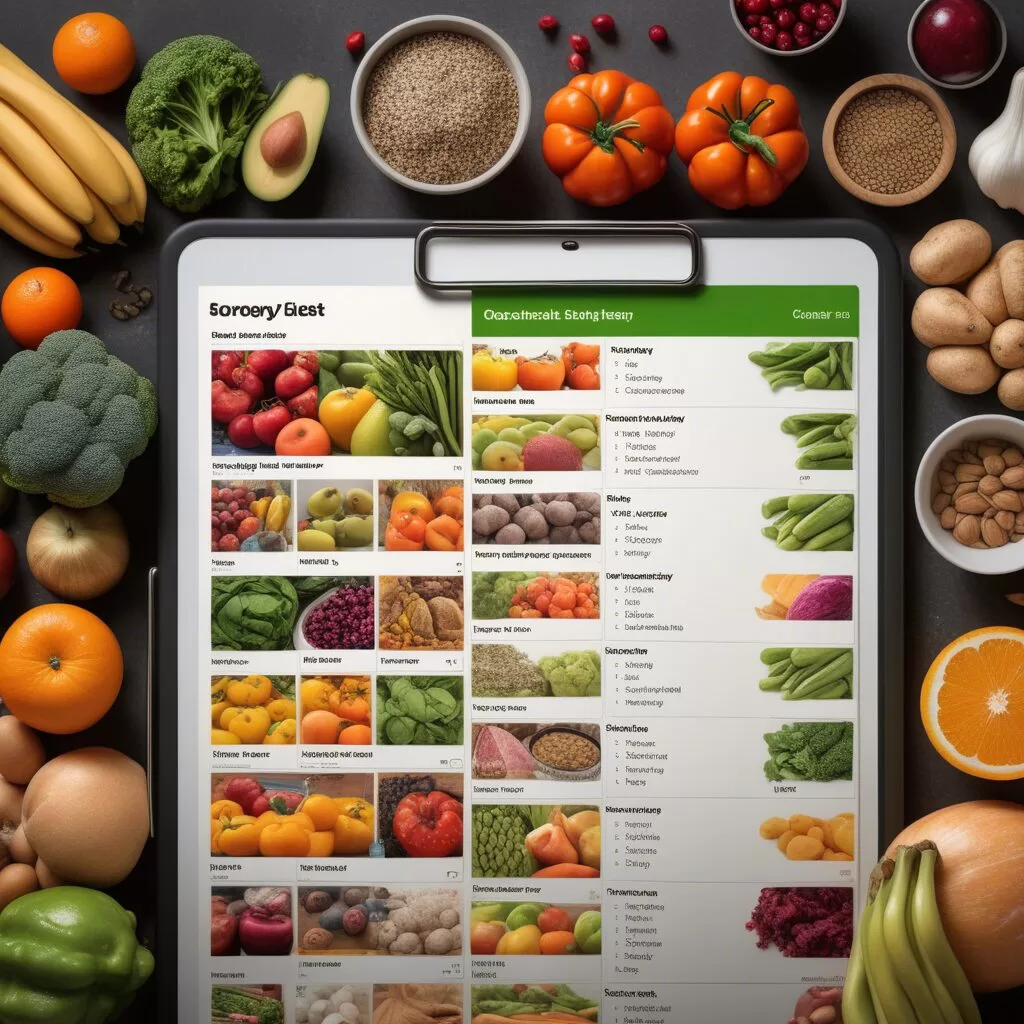
TL/DR:
- Crafting effective AI prompts can transform general responses into personalised, useful answers.
- By refining prompts step-by-step, users can tailor AI outputs to meet specific dietary, budget, and seasonal requirements.
- Including preferences, budget constraints, nutritional focus, recipe suggestions, and seasonal ingredients enhances the relevance and practicality of AI-generated grocery lists.
Asking AI the ‘right’ question can yield powerful, personalised answers. But crafting an effective prompt is a skill—one that can transform a general response into something tailored and useful for your unique needs.
In this guide, we’ll walk through building a prompt step-by-step, using a single everyday task—planning a weekly grocery list—and show you how to go from a simple request to a highly specific list that meets dietary, budget, and even seasonal requirements.
Ready to get the most out of your prompts? Let’s dive in.
Starting Simple
Sometimes, you just want a basic grocery list. Here’s how to start with a simple prompt.
Prompt Example: “Create a grocery list for a family of four with basic ingredients for breakfast, lunch, and dinner.”
What It Does: This basic prompt gives you a standard list. You’ll likely get staple items like eggs, bread, milk, and vegetables for three meals a day, but without much customisation.
Adding Preferences
To make the list more relevant, try including your family’s dietary preferences. Here’s an example with a vegetarian twist.
Prompt Example: “Create a grocery list for a family of four, focusing on vegetarian options for breakfast, lunch, and dinner.”
What It Does: By specifying “vegetarian,” the AI will provide plant-based ingredients, such as beans, lentils, and vegetables, catering to a meat-free diet. This makes the list more aligned with specific lifestyle choices.
Incorporating a Budget
Budget constraints? Let’s factor that in to make the list realistic and wallet-friendly.
Prompt Example: “Create a grocery list for a family of four, with vegetarian options for breakfast, lunch, and dinner, keeping it under $100.”
What It Does: With a budget cap, the AI can adjust portion sizes or prioritise cost-effective ingredients, such as bulk grains or frozen vegetables, to stay within the set limit. This way, you’re not overspending while keeping meals covered for the week.
Adding a Nutritional Focus
To make your grocery list even more valuable, you can add specific nutritional goals, such as high-protein options.
Prompt Example: “Create a grocery list for a family of four, vegetarian meals for breakfast, lunch, and dinner, under $100, prioritising high-protein foods and fresh produce.”
What It Does: Adding a nutritional focus means the AI will include high-protein items, like tofu, quinoa, and chickpeas, while balancing fresh fruits and vegetables. It ensures your family gets the protein they need on a budget.
Including Recipe Suggestions
What if you’d like a little cooking guidance along with the list? Adding recipes can make meal prep a breeze.
Prompt Example: “Create a grocery list for a family of four, with vegetarian meals for breakfast, lunch, and dinner, under $100, focusing on high-protein foods and fresh produce. Include a simple recipe idea for each meal.”
What It Does: With this prompt, the AI can suggest recipes like “Chickpea Salad Wrap” for lunch and “Quinoa Stir-Fry” for dinner. Each meal includes ingredients that are already on the list, so everything is efficiently planned and ready to go.
Enhancing with Seasonal Ingredients
Want your groceries to be sustainable and fresh? Adding a seasonal focus can make all the difference.
Prompt Example: “Create a grocery list for a family of four, vegetarian meals under $100, high-protein with fresh, seasonal produce for November. Include one recipe idea per meal.”
What It Does: This final layer makes the list practical and eco-friendly, focusing on produce like pumpkins, sweet potatoes, and greens that are in season in November. Seasonality ensures freshness, better prices, and sometimes even a tastier meal!
Building Effective Prompts for Everyday Life
As you can see, with each step, your prompt becomes more refined and powerful. By layering in preferences, budget, nutrition, recipe ideas, and seasonality, you create a prompt that meets your needs precisely. Try experimenting with your own prompts and discover how a well-crafted question can unlock the full potential of AI for your everyday life. Whether you’re meal-planning, organising, or budgeting, building your prompts thoughtfully makes the AI experience more rewarding and relevant.
Happy prompt-crafting!
Join the conversation
What are your favourite AI prompts for everyday tasks? Share your tips and tricks in the comments below! And don’t forget to subscribe for updates on AI and AGI developments here. We’d love to hear your thoughts on how AI is transforming your daily life!
You may also like:
- AI Chef: How ChatGPT Created a Smash Hit Pizza
- 15 Advanced Brainstorming Techniques Powered by ChatGPT
- Or tap here to try these prompts at ChatGPT
Author
Discover more from AIinASIA
Subscribe to get the latest posts sent to your email.
Life
Adrian’s Arena: AI and the Global Shift – What Trump’s 2024 Victory Means for AI in Asia
With Trump’s 2024 re-election, Asian nations might push for self-reliant AI ecosystems, regional partnerships, and stronger privacy standards.
Published
1 week agoon
November 6, 2024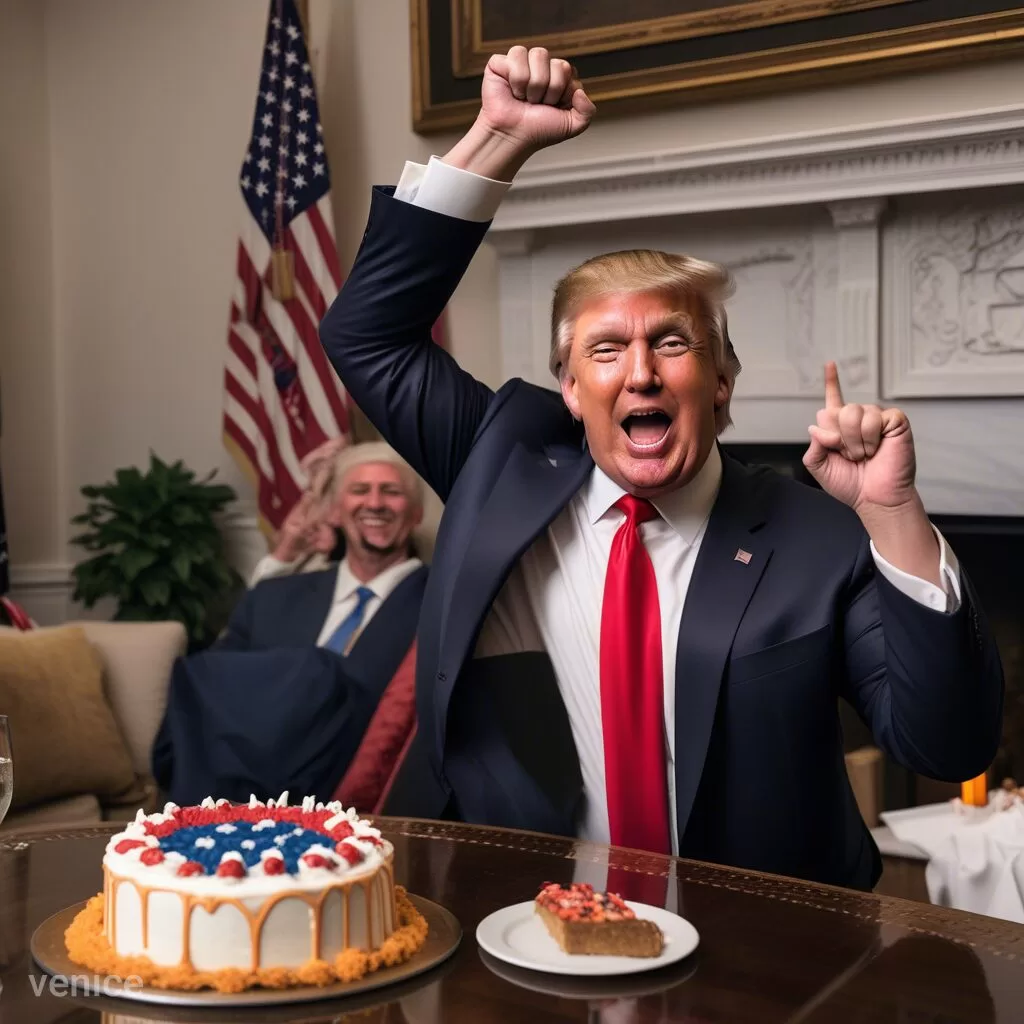
TL;DR
- Donald Trump’s 2024 presidential win could reshape AI development in Asia by prompting self-reliant AI ecosystems, more regional partnerships, and increased privacy standards.
- Asian nations may accelerate AI innovation and talent development to reduce reliance on U.S. tech, particularly as they anticipate shifts due to this result.
- Asian companies are positioned to thrive, offering privacy-compliant, localised AI insights that align with Asia’s unique market dynamics during this new Trump era.
What now for AI?
The re-election of Donald Trump to the U.S. presidency is sure to have profound global impacts, particularly in areas like artificial intelligence (AI). In Asia, where AI adoption is already soaring, Trump’s approach to foreign policy, technology, and economic partnerships may drive significant shifts in both public and private AI ventures. This focus includes Donald Trump’s 2024 election win and subsequent implications on AI in various sectors.
This article explores how the changing political landscape could reshape AI in Asia and how businesses are poised to navigate and leverage these shifts.
AI Regulation and Innovation: A Push for Autonomy
Trump’s leadership may spur a greater focus on AI autonomy in Asia, encouraging countries to develop homegrown AI solutions across various industries. For example, healthcare data analytics in Singapore, fintech solutions in India, and consumer insights platforms in Japan could see accelerated development as these nations prioritise self-reliance.
Several companies in Asia are well-positioned to contribute, offering privacy-compliant AI insights that help brands tailor messaging without relying on U.S.-based tech giants.
Trade Policies and Tech Partnerships: Redrawing Lines
With Trump’s trade policies likely to maintain a “protectionist” edge, tech partnerships across the Pacific may become more complex, leading Asia’s leading economies to bolster regional AI collaborations. This may foster tighter partnerships within Asia, where companies can provide high-impact AI solutions tailored to local consumer behaviours and trends.
Research Funding and Education: A New Wave of Asian Talent
The expected restrictions on U.S. visas for Asian students and researchers could spark a wave of investment in AI education and talent retention across Asia. AI companeis can support this talent surge by offering real-world, Asia-specific AI applications, from data analytics to customer insights and digital advertising.
Practical programs in Asia, especially in Singapore —offer hands-on AI training—equip professionals with critical skills for driving regional innovation, positioning Asia as a powerhouse for AI expertise.
AI-Powered Defense and Cybersecurity: Strengthening Regional Security
As Asian nations fortify their defences in response to Trump’s renewed focus on military alliances, AI-driven cybersecurity solutions are expected to see considerable growth. AI companies in Asia are poised to address emerging threats with precision and speed.
For instance, Asian technology could support national cybersecurity initiatives by identifying threat patterns in real-time across public data sources, providing governments and enterprises with actionable insights for safeguarding critical infrastructure.
Privacy and Data Ownership: Asia’s Standards vs. the U.S. Approach
Asia’s data governance standards are set to diverge further from those in the U.S., especially with Trump’s preference for lighter tech regulation. This shift aligns with ad tech’s approach to delivering privacy-compliant audience insights, offering Asia-based companies a way to engage their customers effectively without compromising data security.
Impact on the AI Talent Pipeline: Challenges and Opportunities
Trump’s immigration policies could impact the AI talent pipeline to the U.S., pushing many skilled AI professionals to remain in Asia. Companies can leverage this shift by tapping into local AI talent for projects that require regional expertise, particularly in the Donald Trump 2024 election AI context.
By prioritising local talent, companies can ensure that solutions align with Asia’s unique market demands, from local consumer insights to culturally resonant AI-driven advertising.
As a result, Asian companies and their partners can benefit from deeper market understanding, making their campaigns more impactful across Asia.
A Shift Towards Pan-Asian AI Standards
With Trump’s policies creating a potential divide in AI development approaches, Asian countries may push for unified AI standards within the region. By aligning AI governance across economies, Asia could build a formidable framework that encourages innovation while ensuring ethical usage and robust privacy protections.
Countries like Japan, South Korea, and Singapore are already leaders in setting high AI standards, and an Asia-wide approach could help establish a distinctive identity in the global AI community.
This alignment would also reduce friction for companies operating across multiple Asian markets, fostering an interconnected ecosystem that accelerates growth and adaptability.
The Rise of Localised AI Applications
As trade and regulatory landscapes shift, there’s an increased incentive for Asian companies to design AI solutions that cater to local languages, cultural nuances, and consumer behaviours. Localisation has always been a critical factor for success in Asia, and AI is no exception.
From natural language processing that understands regional dialects to AI-driven marketing insights that resonate with unique consumer mindsets, tailored AI applications could see a significant boost.
This emphasis on localisation not only enhances user experience but also ensures that AI remains relevant and effective in each unique market across the continent
Conclusion: A New Era for AI in Asia
The Trump presidency may catalyse a new chapter for AI in Asia. As Asian nations brace for potential shifts in trade and technology policies, they are well-positioned to accelerate regional AI innovation, self-sufficiency, and collaboration.
By investing in local talent, fostering privacy-compliant solutions, and collaborating across the region, companies like SQREEM are driving Asia’s transformation into a global AI powerhouse.
While the future may be uncertain under a second new era of Trump, we know at least it won’t be boring for the AI industry!
Join the Conversation
As AI in Asia surges towards autonomy and privacy-first innovation, will Trump’s policies drive the region to outperform the U.S. in tech advancements? Or are we on the cusp of a global AI divide? Please share your thoughts and don’t forget to subscribe for updates on AI and AGI developments.
You may also like:
- AI Chatbots Struggle with Real-Time Political News
- Asia on the Brink: Navigating Elon Musk’s Disturbing Prediction
- Read more about Trump’s impact on AI at our good friends The Diplomat
Author
-
Adrian is an AI, marketing, and technology strategist based in Asia, with over 25 years of experience in the region. Originally from the UK, he has worked with some of the world’s largest tech companies and successfully built and sold several tech businesses. Currently, Adrian leads commercial strategy and negotiations at one of ASEAN’s largest AI companies. Driven by a passion to empower startups and small businesses, he dedicates his spare time to helping them boost performance and efficiency by embracing AI tools. His expertise spans growth and strategy, sales and marketing, go-to-market strategy, AI integration, startup mentoring, and investments. View all posts
Discover more from AIinASIA
Subscribe to get the latest posts sent to your email.

How Can Singapore Strengthen Its Startup Ecosystem?

5 ChatGPT Settings to Boost Your Productivity

How AI-Assisted Music Helped Resurrect The Beatles To Grammy Nominations
Trending
-
Learning2 weeks ago
AI Unleashed: Discover the Power of Notion AI
-
Learning3 weeks ago
AI Unleashed: Discover the Power of Midjourney AI
-
Learning3 weeks ago
AI Unleashed: Discover the Power of ChatGPT
-
Learning3 weeks ago
AI Unleashed: Discover the Power of Claude AI
-
Learning2 weeks ago
AI Unleashed: Discover the Power of Gemini AI
-
Marketing3 weeks ago
Adrian’s Arena: AI is Shaping the Future of Marketing to Gen Z in Southeast Asia
-
Marketing2 weeks ago
Adrian’s Arena: The AI-Driven Playbook for Winning Over APAC Consumers
-
Marketing1 week ago
Adrian’s Arena: Reaching Today’s Consumers – How AI Enhances Digital Marketing