Life
The AI Wars: Navigating the Future of Search in Asia
Winning the AI wars requires a combination of knowledge, empathy, courage, and user-centricity.
Published
10 months agoon
By
AIinAsia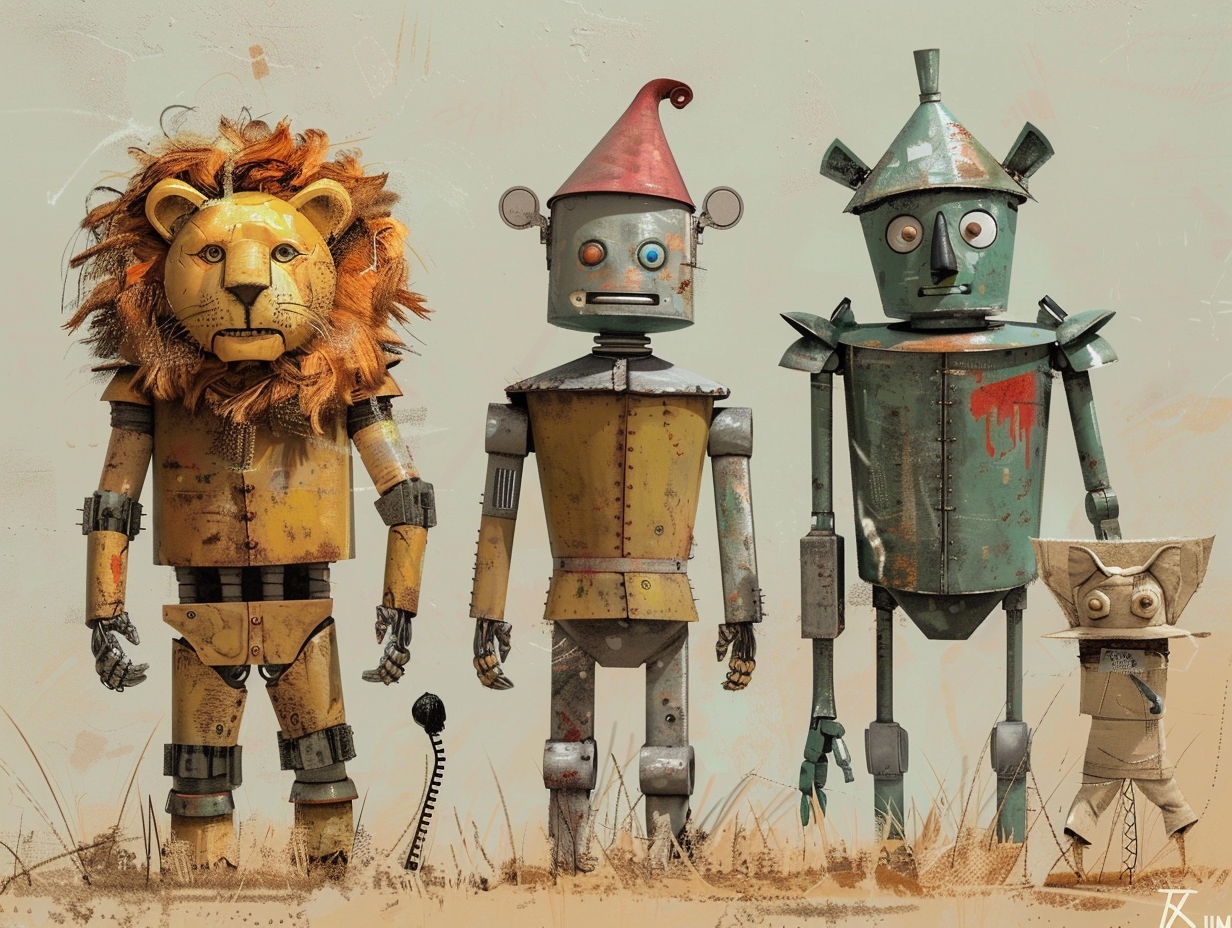
TL;DR:
- The AI wars are heating up, with key players like OpenAI’s ChatGPT, Google’s Gemini, and others vying for dominance.
- The winning AI will need a ‘brain’ (knowledge), a ‘heart’ (empathy), courage, and a sense of ‘home’ (user-centricity).
- Brands must focus on building trust, providing accurate information, and creating empathetic AI experiences to succeed.
The AI Wars: A New Battlefield
Artificial Intelligence (AI) is changing the way we search for information. As traditional search methods become less relevant, AI is stepping in to make finding information more efficient and accurate. This shift has sparked a new kind of war: the AI wars.
Key Players in the AI Wars
The AI landscape is filled with key players like OpenAI’s ChatGPT, Google’s Gemini, and Anthropic’s Claude. Even Apple and Amazon have joined the race with their own Large Language Models (LLMs), Ferret (now MM1) and Project Olympus.
The Winning AI Formula
The winning AI will need a ‘brain’, a ‘heart’, courage, and a sense of ‘home’. This translates to knowledge, empathy, the courage to uphold principles, and a user-centric approach.
Knowledge: The AI’s Brain
AI models need accurate and reliable information to provide valuable responses. Brands that can produce and verify this information will have an advantage. For instance, a retailer like FatBrain, which accurately catalogs the country of origin for all its products, will have an edge over less precise platforms.
Empathy: The AI’s Heart
Empathy will be a crucial factor in the AI world. Users will prefer AI that understands their needs, is patient, respectful, and communicates effectively. Brands must focus on creating empathetic AI experiences to connect with their audience.
Courage: The AI’s Backbone
The winner of the AI war will need the courage to uphold principles of liberty, freedom of speech, and resist the temptation to suppress differing voices. Brands must be courageous in their stance to maintain user trust.
Home: The AI’s User-Centricity
AI must prioritise user needs and preferences. Brands need to focus on building their brand, creating great products, and providing excellent customer service to win customer loyalty.
Navigating the AI Wars
To succeed in the AI wars, brands must focus on building trust, providing accurate information, and creating empathetic AI experiences. The future of search is here, and it’s up to brands to adapt and thrive in this new landscape.
Comment and Share
What do you think about the AI wars? How are you preparing your brand for the future of search? Share your thoughts in the comments below and don’t forget to subscribe for updates on AI and AGI developments.
You may also like:
- The Demise of the Web: How Google and AI are Redefining the Internet
- Human-AI Differences: Artificial Intelligence and the Quest for AGI in Asia
- The Interface War: How Anthropic’s Artifacts Could Redefine AI Collaboration
- For Case studies on AI implementation in Asia tap here.
Author
Discover more from AIinASIA
Subscribe to get the latest posts sent to your email.
You may like
Life
“Sounds Impressive… But for Whom?” Why AI’s Overconfident Medical Summaries Could Be Dangerous
New research shows AI chatbots often turn cautious medical findings into overconfident generalisations. Discover what that means for healthcare communication.
Published
26 minutes agoon
May 13, 2025By
AIinAsia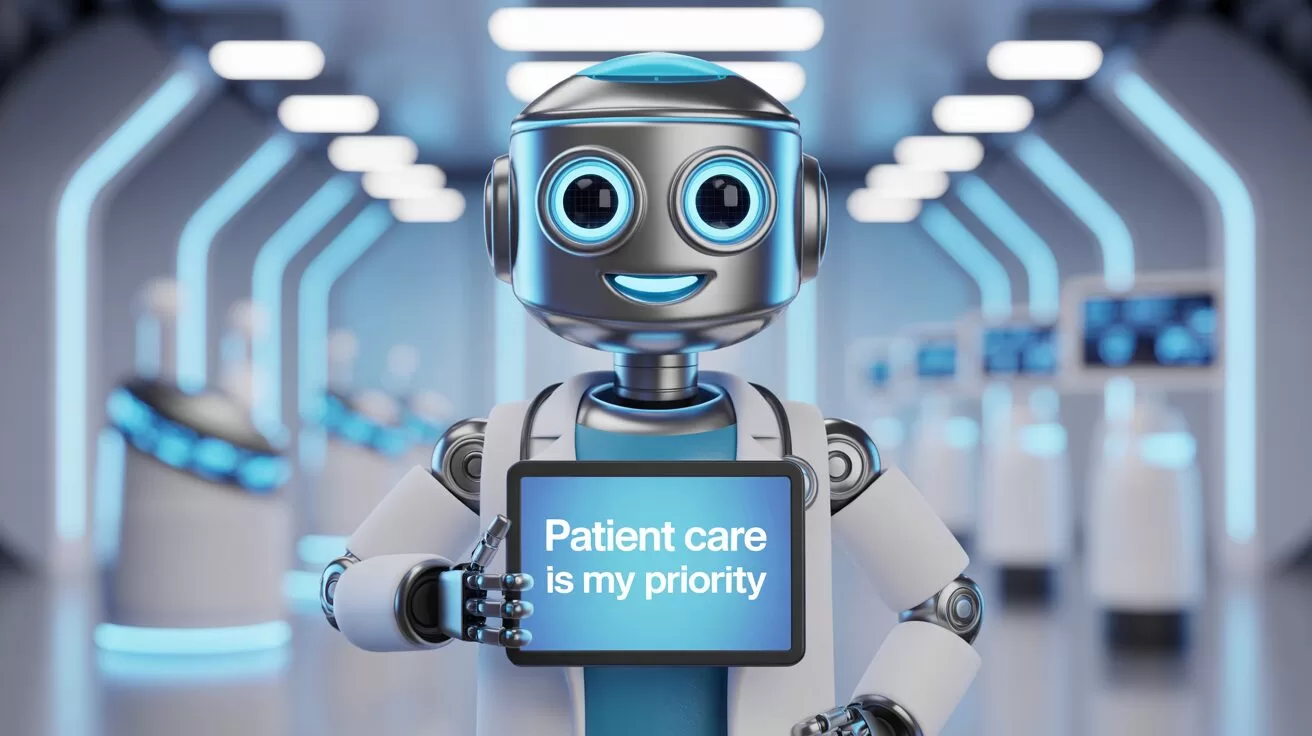
TL;DR — What You Need to Know
- Medical research thrives on precision — but humans and AIs both love to overgeneralise with AI-generated medical summaries.
- New research shows large language models routinely turn cautious medical claims into sweeping, misleading statements.
- Even the best models aren’t immune — and the problem could quietly distort how science is understood and applied.
Why AI-Generated Medical Summaries Could Be Misleading
In medicine, the golden rule is: never say more than your data justifies.
Clinicians and researchers live by this. Journal reviewers demand it. Medical writing, as a result, is often painstakingly specific — sometimes to the point of impenetrability. Take this gem of a conclusion from a real-world trial:
“In a randomised trial of 498 European patients with relapsed or refractory multiple myeloma, the treatment increased median progression-free survival by 4.6 months, with grade three to four adverse events in 60 per cent of patients and modest improvements in quality-of-life scores, though the findings may not generalise to older or less fit populations.”
Meticulous? Yes. Memorable? Not quite.
So, what happens when that careful wording gets trimmed down — for a press release, an infographic, or (increasingly) an AI-generated summary?
It becomes something like:
“The treatment improves survival and quality of life.”
Technically? Not a lie. But practically? That’s a stretch.
From nuance to nonsense: how ‘generics’ mislead
Statements like “the treatment is effective” are what philosophers call generics — sweeping claims without numbers, context, or qualifiers. They’re dangerously seductive in medical research because they sound clear, authoritative, and easy to act on.
But they gloss over crucial questions: For whom? How many? Compared to what? And they’re everywhere.
In a review of over 500 top journal articles, more than half included generalisations that went beyond the data — often with no justification. And over 80% of those were, yep, generics.
This isn’t just sloppiness. It’s human nature. We like tidy stories. We like certainty. But when we simplify science to make it snappy, we risk getting it wrong — and getting it dangerously wrong in fields like medicine.
Enter AI. And it’s making the problem worse.
Our latest research put 10 of the world’s most popular large language models (LLMs) to the test — including ChatGPT, Claude, LLaMA and DeepSeek. We asked them to summarise thousands of real medical abstracts.
Even when prompted for accuracy, most models:
- Dropped qualifiers
- Flattened nuance
- Turned cautious claims into confident-sounding generics
In short: they said more than the data allowed.
In some cases, 73% of summaries included over-generalisations. And when compared to human-written summaries, the bots were five times more likely to overstate findings.
Worryingly, newer models — including the much-hyped GPT-4o — were more likely to generalise than earlier ones.
Why is this happening?
Partly, it’s in the training data. If scientific papers, press releases and past summaries already overgeneralise, the AI inherits that tendency. And through reinforcement learning — where human approval influences model behaviour — AIs learn to prioritise sounding confident over being correct. After all, users often reward answers that feel clear and decisive.
The stakes? Huge.
Medical professionals, students and researchers are turning to LLMs in droves. In a recent survey of 5,000 researchers:
- Nearly half already use AI to summarise scientific work.
- 58% believe AI outperforms humans in this task.
That confidence might be misplaced. If AI tools continue to repackage nuanced science into generic soundbites, we risk spreading misunderstandings at scale — especially dangerous in healthcare.
What needs to change?
For humans:
- Editorial guidelines need to explicitly discourage generics without justification.
- Researchers using AI summaries should double-check outputs, especially in critical fields like medicine.
For AI developers:
- Models should be fine-tuned to favour caution over confidence.
- Built-in prompts should steer summaries away from overgeneralisation.
For everyone:
- Tools that benchmark overgeneralisation — like the methodology in our study — should become part of AI model evaluation before deployment in high-stakes domains.
Because here’s the bottom line: in medicine, precision saves lives. Misleading simplicity doesn’t.
So… next time your chatbot says “The drug is effective,” will you ask: for whom, exactly?
You may also like:
- Google’s Med-Gemini Outshines GPT in Clinical Diagnostics
- AI Revolution: How Google’s Search Updates Could Impact Your Online Business
- Masterclass: Crafting Effective ChatGPT Prompts in Healthcare in 2024
Author
Discover more from AIinASIA
Subscribe to get the latest posts sent to your email.
Life
Whose English Is Your AI Speaking?
AI tools default to mainstream American English, excluding global voices. Why it matters and what inclusive language design could look like.
Published
3 days agoon
May 10, 2025By
AIinAsia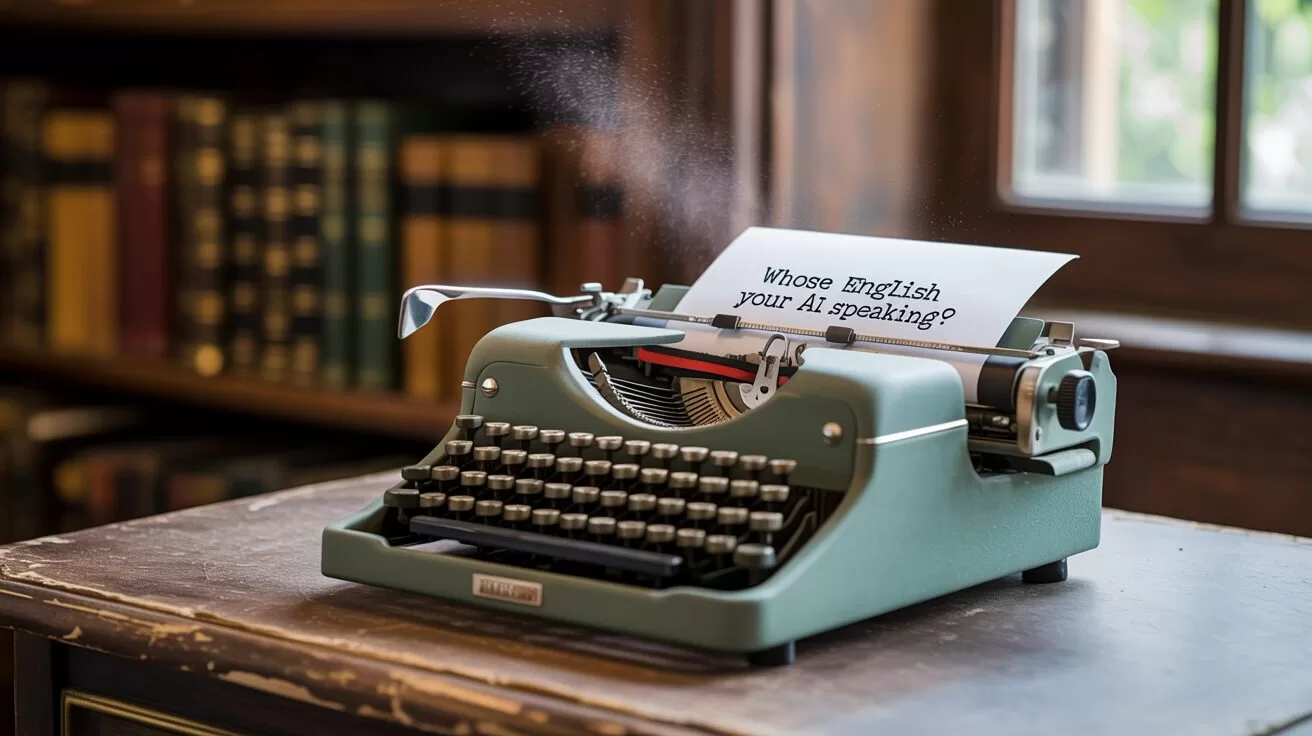
TL;DR — What You Need To Know
- Most AI tools are trained on mainstream American English, ignoring global Englishes like Singlish or Indian English
- This leads to bias, miscommunication, and exclusion in real-world applications
- To fix it, we need AI that recognises linguistic diversity—not corrects it.
English Bias In AI
Here’s a fun fact that’s not so fun when you think about it: 90% of generative AI training data is in English. But not just any English. Not Nigerian English. Not Indian English. Not the English you’d hear in Singapore’s hawker centres or on the streets of Liverpool. Nope. It’s mostly good ol’ mainstream American English.
That’s the voice most AI systems have learned to mimic, model, and prioritise. Not because it’s better. But because that’s what’s been fed into the system.
So what happens when you build global technology on a single, dominant dialect?
A Monolingual Machine in a Multilingual World
Let’s be clear: English isn’t one language. It’s many. About 1.5 billion people speak it, and almost all of them do so with their own twist. Grammar, vocabulary, intonation, slang—it all varies.
But when your AI tools—from autocorrect to resume scanners—are only trained on one flavour of English (mostly US-centric, polished, white-collar English), a lot of other voices start to disappear. And not quietly.
Speakers of regional or “non-standard” English often find their words flagged as incorrect, their accents ignored, or their syntax marked as a mistake. And that’s not just inconvenient—it’s exclusionary.
Why Mainstream American English Took Over
This dominance didn’t happen by chance. It’s historical, economic, and deeply structural.
The internet was largely developed in the US. Big Tech? Still mostly based there. The datasets used to train AI? Scraped from web content dominated by American media, forums, and publishing.
So, whether you’re chatting with a voice assistant or asking ChatGPT to write your email, what you’re hearing back is often a polished, neutral-sounding, corporate-friendly version of American English. The kind that gets labelled “standard” by systems that were never trained to value anything else.
When AI Gets It Wrong—And Who Pays the Price
Let’s play this out in real life.
- An AI tutor can’t parse a Nigerian English question? The student loses confidence.
- A resume written in Indian English gets rejected by an automated scanner? The applicant misses out.
- Voice transcription software mangles an Australian First Nations story? Cultural heritage gets distorted.
These aren’t small glitches. They’re big failures with real-world consequences. And they’re happening as AI tools are rolled out everywhere—into schools, offices, government services, and creative workspaces.
It’s “Englishes”, Plural
If you’ve grown up being told your English was “wrong,” here’s your reminder: It’s not.
Singlish? Not broken. Just brilliant. Indian English? Full of expressive, efficient, and clever turns of phrase. Aboriginal English? Entirely valid, with its own rules and rich oral traditions.
Language is fluid, social, and fiercely local. And every community that’s been handed English has reshaped it, stretched it, owned it.
But many AI systems still treat these variations as noise. Not worth training on. Not important enough to include in benchmarks. Not profitable to prioritise. So they get left out—and with them, so do their speakers.
Towards Linguistic Justice in AI
Fixing this doesn’t mean rewriting everyone’s grammar. It means rewriting the technology.
We need to stop asking AI to uphold one “correct” form of English, and start asking it to understand the many. That takes:
- More inclusive training data – built on diverse voices, not just dominant ones
- Cross-disciplinary collaboration – between linguists, engineers, educators, and community leaders
- Respect for language rights – including the choice not to digitise certain cultural knowledge
- A mindset shift – from standardising language to supporting expression
Because the goal isn’t to “correct” the speaker. It’s to make the system smarter, fairer, and more reflective of the world it serves.
Ask Yourself: Whose English Is It Anyway?
Next time your AI assistant “fixes” your sentence or flags your phrasing, take a second to pause. Ask: whose English is this system trying to emulate? And more importantly, whose English is it leaving behind?
Language has always been a site of power—but also of play, resistance, and identity. The way forward for AI isn’t more uniformity. It’s more Englishes, embraced on their own terms.
You may also like:
- How Singtel Used AI to Bring Generations Together for Singapore’s SG60
- Revolutionising Workspaces: The Surge of AI and ChatGPT in Indian Companies
- Or try out the free version of Claude AI by tapping here.
Author
Discover more from AIinASIA
Subscribe to get the latest posts sent to your email.
Business
Build Your Own Agentic AI — No Coding Required
Want to build a smart AI agent without coding? Here’s how to use ChatGPT and no-code tools to create your own agentic AI — step by step.
Published
4 days agoon
May 9, 2025By
AIinAsia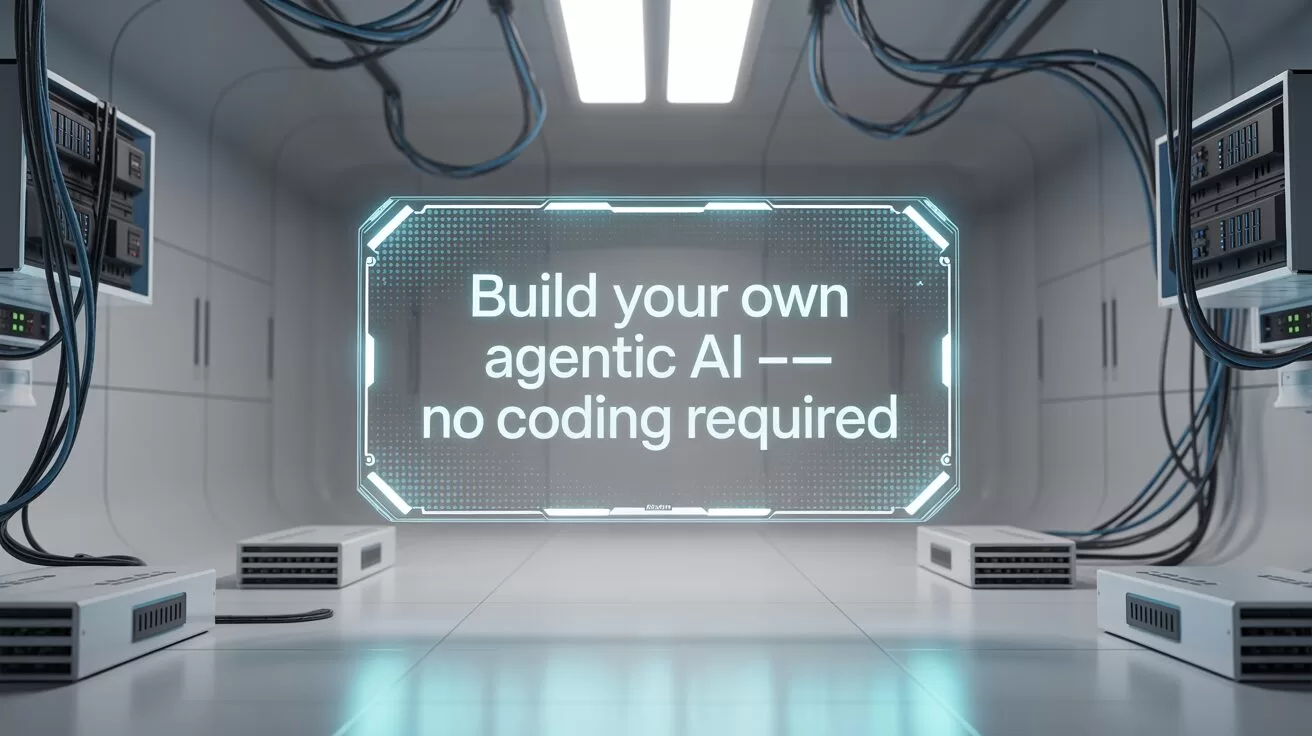
TL;DR — What You Need to Know About Agentic AI
- Anyone can now build a powerful AI agent using ChatGPT — no technical skills needed.
- Tools like Custom GPTs and Make.com make it easy to create agents that do more than chat — they take action.
- The key is to start with a clear purpose, test it in real-world conditions, and expand as your needs grow.
Anyone Can Build One — And That Includes You
Not too long ago, building a truly capable AI agent felt like something only Silicon Valley engineers could pull off. But the landscape has changed. You don’t need a background in programming or data science anymore — you just need a clear idea of what you want your AI to do, and access to a few easy-to-use tools.
Whether you’re a startup founder looking to automate support, a marketer wanting to build a digital assistant, or simply someone curious about AI, creating your own agent is now well within reach.
What Does ‘Agentic’ Mean, Exactly?
Think of an agentic AI as something far more capable than a standard chatbot. It’s an AI that doesn’t just reply to questions — it can actually do things. That might mean sending emails, pulling information from the web, updating spreadsheets, or interacting with third-party tools and systems.
The difference lies in autonomy. A typical chatbot might respond with a script or FAQ-style answer. An agentic AI, on the other hand, understands the user’s intent, takes appropriate action, and adapts based on ongoing feedback and instructions. It behaves more like a digital team member than a digital toy.
Step 1: Define What You Want It to Do
Before you dive into building anything, it’s important to get crystal clear on what role your agent will play.
Ask yourself:
- Who is going to use this agent?
- What specific tasks should it be responsible for?
- Are there repetitive processes it can take off your plate?
For instance, if you run an online business, you might want an agent that handles frequently asked questions, helps users track their orders, and flags complex queries for human follow-up. If you’re in consulting, your agent could be designed to book meetings, answer basic service questions, or even pre-qualify leads.
Be practical. Focus on solving one or two real problems. You can always expand its capabilities later.
Step 2: Pick a No-Code Platform to Build On
Now comes the fun part: choosing the right platform. If you’re new to this, I recommend starting with OpenAI’s Custom GPTs — it’s the most accessible option and designed for non-coders.
Custom GPTs allow you to build your own version of ChatGPT by simply describing what you want it to do. No technical setup required. You’ll need a ChatGPT Plus or Team subscription to access this feature, but once inside, the process is remarkably straightforward.
If you’re aiming for more complex automation — such as integrating your agent with email systems, customer databases, or CRMs — you may want to explore other no-code platforms like Make.com (formerly Integromat), Dialogflow, or Bubble.io. These offer visual builders where you can map out flows, connect apps, and define logic — all without needing to write a single line of code.
Step 3: Use ChatGPT’s Custom GPT Builder
Let’s say you’ve opted for the Custom GPT route — here’s how to get started.
First, log in to your ChatGPT account and select “Explore GPTs” from the sidebar. Click on “Create,” and you’ll be prompted to describe your agent in natural language. That’s it — just describe what the agent should do, how it should behave, and what tone it should take. For example:
“You are a friendly and professional assistant for my online skincare shop. You help customers with questions about product ingredients, delivery options, and how to track their order status.”
Once you’ve set the description, you can go further by uploading reference materials such as product catalogues, FAQs, or policies. These will give your agent deeper knowledge to draw from. You can also choose to enable additional tools like web browsing or code interpretation, depending on your needs.
Then, test it. Interact with your agent just like a customer would. If it stumbles, refine your instructions. Think of it like coaching — the more clearly you guide it, the better the output becomes.
Step 4: Go Further with Visual Builders
If you’re looking to connect your agent to the outside world — such as pulling data from a spreadsheet, triggering a workflow in your CRM, or sending a Slack message — that’s where tools like Make.com come in.
These platforms allow you to visually design workflows by dragging and dropping different actions and services into a flowchart-style builder. You can set up scenarios like:
- A user asks the agent, “Where’s my order?”
- The agent extracts key info (e.g. email or order number)
- It looks up the order via an API or database
- It responds with the latest shipping status, all in real time
The experience feels a bit like setting up rules in Zapier, but with more control over logic and branching paths. These platforms open up serious possibilities without requiring a developer on your team.
Step 5: Train It, Test It, Then Launch
Once your agent is built, don’t stop there. Test it with real people — ideally your target users. Watch how they interact with it. Are there questions it can’t answer? Instructions it misinterprets? Fix those, and iterate as you go.
Training doesn’t mean coding — it just means improving the agent’s understanding and behaviour by updating your descriptions, feeding it more examples, or adjusting its structure in the visual builder.
Over time, your agent will become more capable, confident, and useful. Think of it as a digital intern that never sleeps — but needs a bit of initial training to perform well.
Why Build One?
The most obvious reason is time. An AI agent can handle repetitive questions, assist users around the clock, and reduce the strain on your support or operations team.
But there’s also the strategic edge. As more companies move towards automation and AI-led support, offering a smart, responsive agent isn’t just a nice-to-have — it’s quickly becoming an expectation.
And here’s the kicker: you don’t need a big team or budget to get started. You just need clarity, curiosity, and a bit of time to explore.
Where to Begin
If you’ve got a ChatGPT Plus account, start by building a Custom GPT. You’ll get an immediate sense of what’s possible. Then, if you need more, look at integrating Make.com or another builder that fits your workflow.
The world of agentic AI is no longer reserved for the technically gifted. It’s now open to creators, business owners, educators, and anyone else with a problem to solve and a bit of imagination.
What kind of AI agent would you build — and what would you have it do for you first? Let us know in the comments below!
You may also like:
- How To Start Using AI Agents To Transform Your Business
- Revolution Ahead: Microsoft’s AI Agents Set to Transform Asian Workplaces
- AI Chatbots: 10 Best ChatGPTs in the ChatGPT Store
- Or tap here to try this out now at ChatGPT by tapping here.
Author
Discover more from AIinASIA
Subscribe to get the latest posts sent to your email.

“Sounds Impressive… But for Whom?” Why AI’s Overconfident Medical Summaries Could Be Dangerous

Whose English Is Your AI Speaking?

Edit AI Images on the Go with Gemini’s New Update
Trending
-
Marketing3 weeks ago
Playbook: How to Use Ideogram.ai (no design skills required!)
-
Life2 weeks ago
WhatsApp Confirms How To Block Meta AI From Your Chats
-
Business2 weeks ago
ChatGPT Just Quietly Released “Memory with Search” – Here’s What You Need to Know
-
Tools3 days ago
Edit AI Images on the Go with Gemini’s New Update
-
Life1 week ago
Geoffrey Hinton’s AI Wake-Up Call — Are We Raising a Killer Cub?
-
Life26 minutes ago
“Sounds Impressive… But for Whom?” Why AI’s Overconfident Medical Summaries Could Be Dangerous
-
Life7 days ago
Too Nice for Comfort? Why OpenAI Rolled Back GPT-4o’s Sycophantic Personality Update
-
Life3 days ago
Whose English Is Your AI Speaking?