Life
Revolutionising Crime-Solving: AI Detectives on the Beat
Explore the potential of AI in law enforcement with Soze, a system that can analyse vast amounts of data quickly. Discover the benefits and challenges of this technology.
Published
2 weeks agoon
By
AIinAsia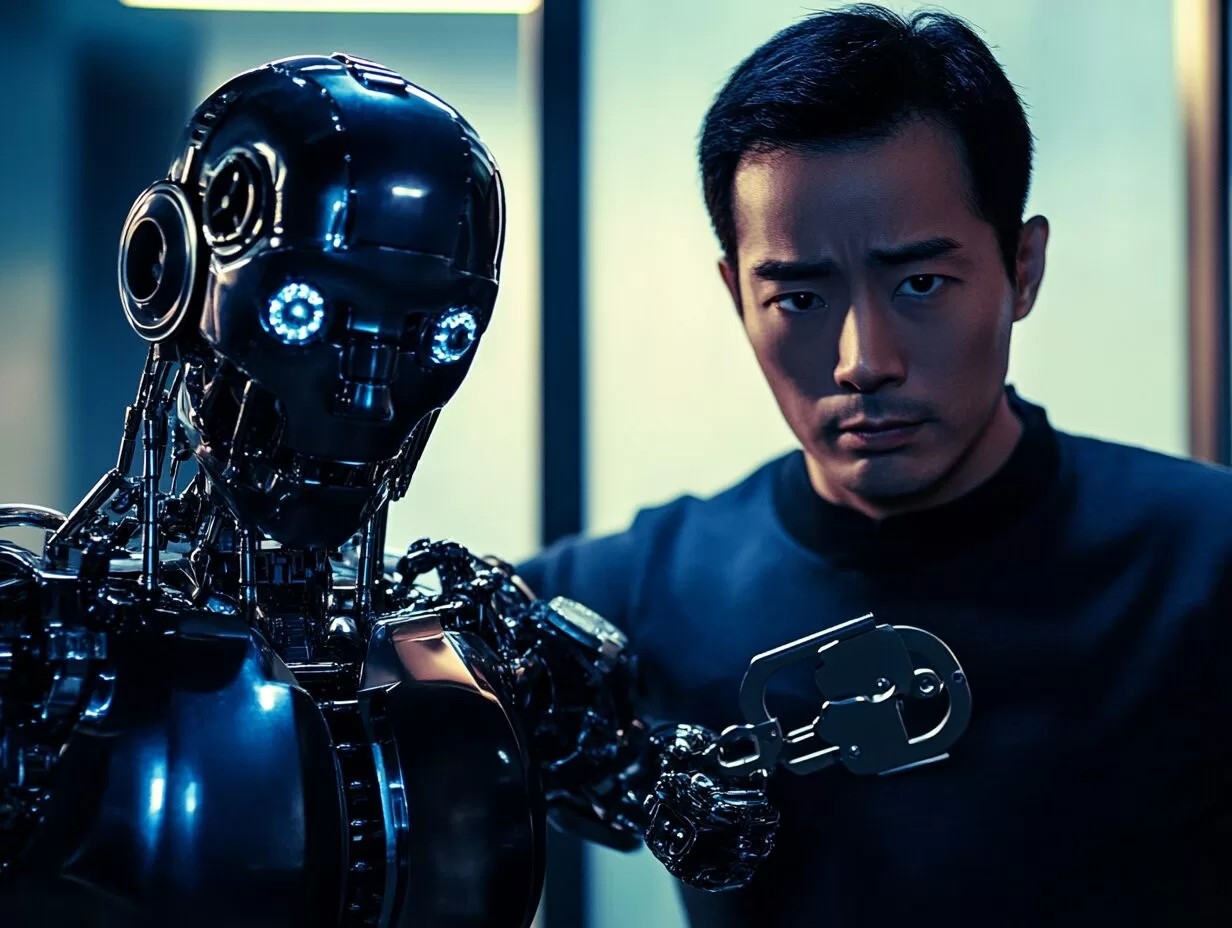
TL;DR:
- AI-powered system, Soze, is being tested by UK police to solve cold cases by analysing vast amounts of data quickly.
- The system scanned evidence from 27 complex cases in 30 hours, equivalent to 81 years of human work.
- Concerns remain about the accuracy and potential biases of AI in law enforcement.
In the fast-paced world of technology, artificial intelligence (AI) is making waves in various sectors, including law enforcement. A police department in the United Kingdom is currently testing an AI-powered system that could revolutionise crime-solving, particularly for cold cases. This innovative approach is not without its controversies, however. Let’s delve into the details of this cutting-edge technology and its implications.
The Power of AI in Crime-Solving
The Avon and Somerset Police Department is at the forefront of this technological advancement. They are testing an AI system called Soze, developed in Australia, which has the potential to condense decades of detective work into mere hours. According to Sky News, the AI was able to scan and analyse evidence from 27 complex cases in about 30 hours. This is equivalent to 81 years of human work, highlighting the system’s incredible efficiency.
Gavin Stephens, the chairman of the UK’s National Police Chiefs’ Council, expressed his optimism about the technology. He noted that Soze could be particularly useful for cold cases with vast amounts of material. The system can ingest and assess this data quickly, providing a fresh perspective that could lead to breakthroughs.
How Soze Works
Soze is designed to scan and analyse various types of evidence, including emails, social media accounts, videos, financial statements, and other documents. By processing this data at an unprecedented speed, the AI can help detectives uncover patterns and connections that might have been missed otherwise. This capability makes Soze a valuable tool for law enforcement agencies, especially those facing personnel and budget constraints.
Concerns and Challenges
While the potential benefits of Soze are impressive, there are significant concerns about its accuracy and reliability. AI models are known to produce incorrect results or even hallucinate information. This is particularly problematic in law enforcement, where false positives can have severe consequences.
Stephens also mentioned another AI project that involves creating a database of knives and swords used in crimes. While this could be a useful tool for investigations, it is crucial to ensure that the AI systems are working correctly and without bias.
Bias and Inaccuracies in AI
One of the most concerning aspects of AI in law enforcement is the potential for bias. A model used to predict a suspect’s likelihood of committing future crimes was found to be inaccurate and biased against Black people. This echoes the themes of Philip K. Dick’s “Minority Report,” where predictive policing leads to false arrests and injustices.
Facial recognition technology, another AI application, has also been criticised for its inaccuracies. Minorities have been wrongly accused of crimes due to false positives generated by these systems. These issues are so concerning that the US Commission on Civil Rights has criticised the use of AI in policing.
The Human Factor
It is essential to remember that AI systems are built on data collected by humans, who can be biased and prone to errors. This means that familiar issues are often baked into the AI from the start. There is a common misconception that machines are infallible, but the reality is more complex.
The Future of AI in Law Enforcement
Despite the challenges, the potential of AI in law enforcement is undeniable. Systems like Soze could significantly enhance the efficiency and effectiveness of investigations. However, it is crucial to address the concerns about accuracy and bias before these technologies are widely adopted.
Ensuring Fairness in AI
Before implementing AI systems in law enforcement, it is essential to conduct thorough testing and validation. This includes assessing the system’s accuracy and checking for any biases in its algorithms. Additionally, law enforcement agencies should be transparent about their use of AI and engage with the public to build trust.
Comment and Share:
We would love to hear your thoughts on the use of AI in law enforcement. Do you think systems like Soze could revolutionise crime-solving, or are you concerned about the potential for bias and inaccuracies? Share your experiences and opinions in the comments below. Don’t forget to subscribe for updates on AI and AGI developments.
You may also like:
- AI vs. Human Bias: The Fight for Fair Recruitment in the Digital Age
- The Truth About OpenAI’s o1: Is It Worth the Hype?
- Can Facial Recognition Reveal Your Deepest Secrets?
- To learn more about the use of AI in law enforcement, tap here.
Author
Discover more from AIinASIA
Subscribe to get the latest posts sent to your email.
You may like
-
The Rise of AI in Indonesia: A $366 Billion Opportunity
-
China’s Bold Move: Shaping Global AI Regulation with Watermarks
-
ChatGPT’s Unsettling Advance: Is It Getting Too Smart?
-
Nevada’s AI Experiment: Unemployment Benefits Decided by Machines
-
The Secret Weapon Against AI Plagiarism: Watermarking
-
AI in Asia: Bridging the Risk Management Gap
Life
AI Revolution at Wimbledon: 300 Jobs at Risk as Tradition Fades
AI in Wimbledon: The future of tennis and the impact on jobs.
Published
22 hours agoon
October 15, 2024By
AIinAsia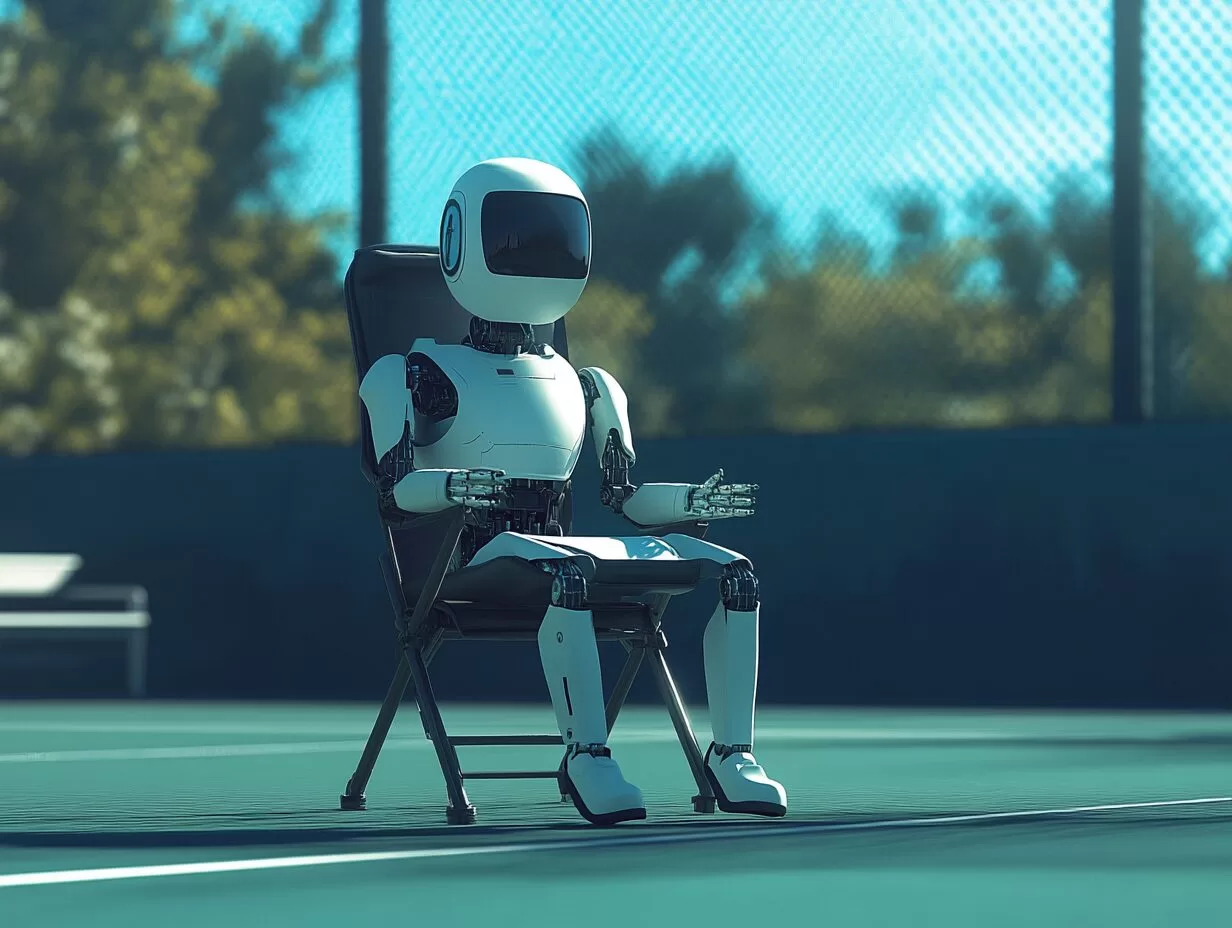
TL;DR:
- Wimbledon plans to replace line judges with AI, putting 300 jobs at risk.
- The decision follows the successful use of Hawk-Eye Live at the 2020 US Open.
- Experts express concerns about job losses and the human element in tennis.
The AI Revolution at Wimbledon
Wimbledon, one of the world’s most prestigious tennis tournaments, is set to undergo a significant transformation. The All England Club has announced plans to replace line judges with Artificial Intelligence (AI) from next year. This decision has left many staff members devastated, as it puts 300 jobs at risk. The move comes four years after the successful implementation of the Hawk-Eye Live system at the 2020 US Open.
A Tradition Comes to an End
Line judges have been an integral part of Wimbledon since its inception 147 years ago. Their presence on the court has been a symbol of tradition and human involvement in the sport. However, the tide of AI seems unstoppable, and Wimbledon is not immune to its influence.
The Impact on Jobs
The decision to replace line judges with AI has sparked concerns about job losses. Chair umpire Richard Ings, speaking to the Telegraph, described it as a “sad but inevitable day.” He noted that while AI brings gains, it also results in the loss of the human touch. Ings said, “Nothing will hold back the tide of AI. And these technologies create gains for sure, but we also lose something on the human side. Will your job be safe from AI?”
The Human Element in Tennis
Ings also highlighted the emotional impact on line judges, stating that they have had their “love and passion ripped away” following the controversial call. He added, “More than 300 good people and excellent officials – the best of the best working at the pinnacle of the sport – today had their love and passion ripped away.”
John Parry, who umpired eight Wimbledon finals during his career, shared similar sentiments. He said, “It’s just a feeling of sadness because there are quite a nucleus of line judges at the top level who are now out of a job.” Parry also noted that some players, including Roger Federer, valued the human element in the game.
Concerns for Lesser Tournaments
The decision to replace line judges with AI has raised concerns about the future of lesser tennis tournaments. Those hoping to become line judges will no longer have the opportunity to work at a Grand Slam, which could lead to recruitment issues. Andrew Jarrett, a former Wimbledon referee, expressed his worries about the future. He said, “I saw the announcement and it’s inevitable, I think. It’s progress, like it or not. But there’s potentially more of a problem further down the food chain. Small pro events that can’t afford ELC (electronic line-calling) may struggle to source officials who no longer have the incentive of being able to prove their worth for selection to Wimbledon.”
The Lawn Tennis Association’s Response
The Lawn Tennis Association (LTA) has acknowledged the concerns and is working with the Association of British Tennis Officials to develop a new strategy. The aim is to ensure that officials can be retained within the sport. However, the future remains uncertain for many line judges.
The Future of AI in Sports
The decision to replace line judges with AI at Wimbledon is a significant step in the integration of technology into sports. While AI offers many benefits, such as increased accuracy and efficiency, it also raises important questions about the role of humans in the sporting world. As AI continues to advance, it is crucial to consider the impact on jobs and the human element in sports.
The decision to replace line judges with AI at Wimbledon marks a significant shift in the world of tennis. While AI offers many benefits, it also raises important questions about job security and the human element in sports. As AI continues to advance, it is crucial to consider its impact on the sporting world and the people who work within it. The future of AI in sports is uncertain, but one thing is clear: the tide of technology is unstoppable.
Comment and Share:
What are your thoughts on the decision to replace line judges with AI at Wimbledon? Do you think this is a positive step forward for the sport, or are you concerned about the loss of the human touch? Share your opinions in the comments below and subscribe for updates on AI and AGI developments.
- You may also like:
- The Future of Sports: How AI and AGI are Revolutionising Olympic Victories
- AI Takes Centre Stage at the Paris Olympics: A Tale of Two Broadcasters
- How professional Football is using AI to improve Corner Kicks
- To learn more about AI’s impact on tennis tap here.
Author
Discover more from AIinASIA
Subscribe to get the latest posts sent to your email.
Life
Are You Ready for the AI Revolution? Prepare for 2027!
The AI Revolution by 2027 is closer than you think. Learn how to prepare for AI’s explosive growth and its impact on the future.
Published
2 days agoon
October 14, 2024By
AIinAsia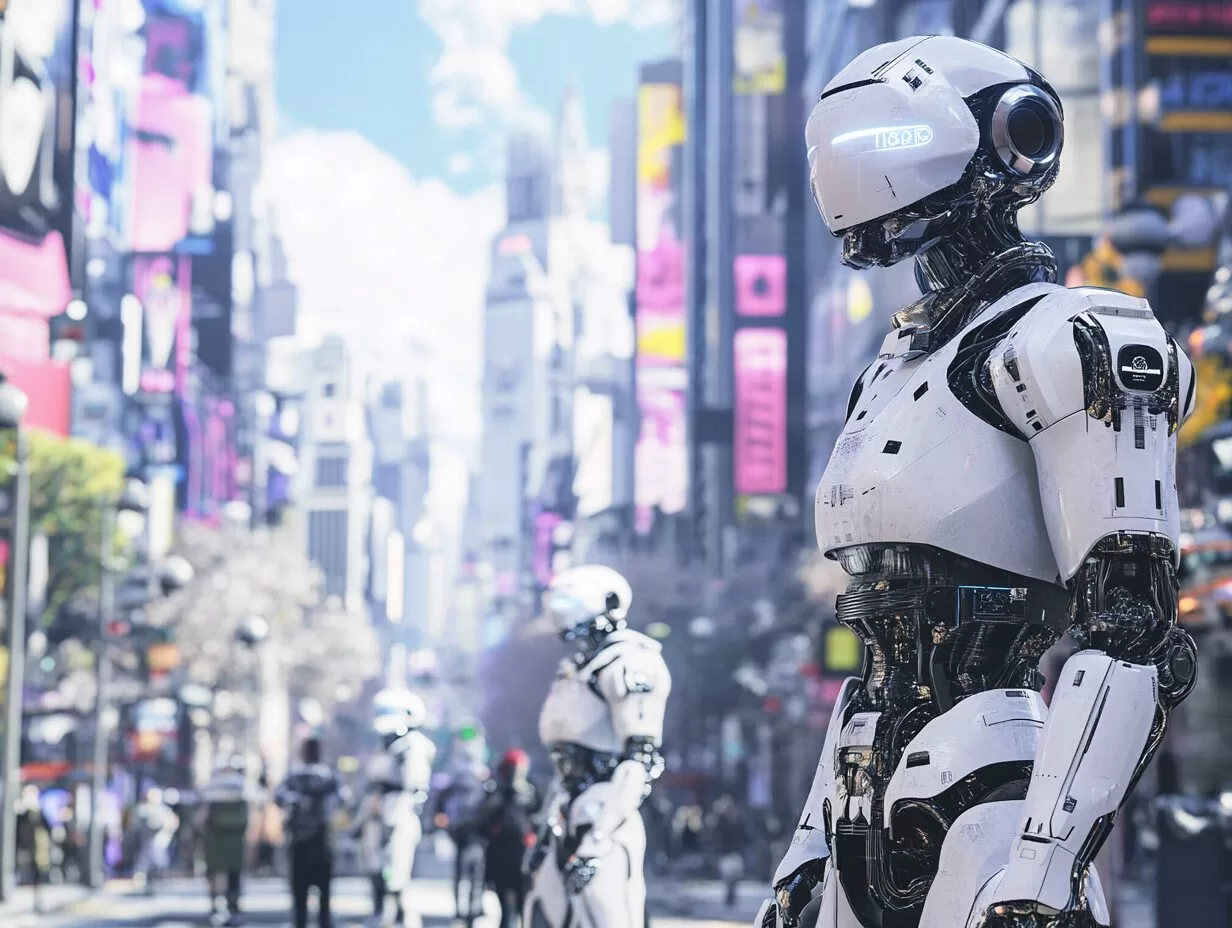
TL;DR:
- AI is developing at an unprecedented speed, with models becoming 5X better each year.
- By 2027, AI could reach the intelligence level of top PhDs, leading to an intelligence explosion.
- The AI industrial revolution will bring rapid advancements, potentially disrupting jobs and economies.
Imagine waking up one day to find AI has revolutionised the world overnight. Sound far-fetched? It’s closer than you think. Not many people realise how quickly AI is developing. By the time you finish this article, you’ll not only understand the AI revolution’s trajectory but also know how to prepare for it. And when AI superintelligence emerges in 2027? You’ll be ready.
From Smart to Superhuman: AI’s Explosive Growth by 2027
Most bullets travel faster than the speed of sound, making them invisible to the naked eye. This is similar to the speed of AI development—so fast that most people won’t see it coming.
According to Leopold in his essay “Situational Awareness”, ChatGPT models are evolving at the speed of ~0.5 OOMs/year. In other words, AI is becoming 5X better each year, compounding over time.
This means that by 2027/28, ChatGPT and other LLMs—large Language Models—will be as smart as the top PhDs worldwide.
“Over and over again, year after year, skeptics have claimed ‘deep learning won’t be able to do X’ and have been quickly proven wrong. If there’s one lesson we’ve learned from the past decade of AI, it’s that you should never bet against deep learning.”
— Leopold Aschenbrenner
Advertisement
Once AIs reach the level of knowledge of top PhDs, they won’t just know as much as one smart PhD; they will know as much as one million PhDs combined.
Just picture that.
At the moment, perhaps a few thousand smart people are researching and developing AI. However, once AI becomes smarter than those developing it, it will be able to improve itself at a much faster rate than human researchers can.
Once AI systems reach that level, they can automate AI research itself, triggering powerful feedback loops that could accelerate development so rapidly that we won’t be able to catch up.
AI is developing this fast for three main reasons:
- The amount of computing power dedicated to AI is continually increasing. Companies like Nvidia produce billions of dollars worth of AI chips every year.
- AI algorithms are becoming better and faster. In less than two years, ChatGPT became 1,000 times more efficient, with AI companies investing billions to improve these algorithms.
- Improvements to algorithms include enhanced Reinforcement Learning from Human Feedback (RLHF) and better Chain of Thought.
By 2027, don’t be surprised if your new “smart” fridge denies you that midnight snack because it has calculated a better diet plan for you.
2028’s World: When AI = 1 Million Human Scientists
AI progress will not stop at the human level.
Hundreds of millions of AGIs will automate AI research, compressing a decade of algorithmic progress into less than or equal to 1 year.
We will go very fast from college degree-level AIs (what we have at the time of writing this article) to superhuman AI systems.
The power of AI superintelligence will surprise almost everyone, as its growth will compound extremely fast.
As Irving John Good predicted, AI will explode:
“An ultraintelligent machine could design even better machines; there would then unquestionably be an ‘intelligence explosion,’ and the intelligence of man would be left far behind. Thus the first ultraintelligent machine is the last invention that man need ever make.”
— Irving John Good, 1965
While AlphaGo may seem focused solely on a game, it illustrates that AIs can surpass human abilities in potentially any field.
Soon, ChatGPT and other LLMs will be vastly smarter than us, akin to an AI earning a PhD while humans are still at the elementary school level.
AIs will literally leave humans in the dust!
This is the full-blown AGI — Artificial General Intelligence — future that will surprise most people, make many jobs redundant, and disrupt businesses that fail to adapt.
And it’s not that far away.
When Will AGI Happen?
At the current pace, we can expect to have AGI in just four years.
Once achieved, AGI will automate AI research itself, leading to an extreme explosion in superintelligence. This is akin to having millions of smart human researchers working 24/7.
Just think about it: once we achieve AGI, the massive GPU data centres we are building will host millions of AGIs running simultaneously.
This will be equivalent to 100 million human-equivalent intelligences operating at speeds ten times faster than humans.
These AIs will work on significant human problems, such as curing diseases, conducting longevity research, and solving various other issues, all while improving themselves.
They will also be replacing human jobs. Lots of them.
More than ever, you need to adapt and be at the forefront of these developments.
This is no longer science fiction; it’s something that will happen soon.
The Power of Superintelligence
The super-fast development of superintelligent AI systems by the end of this decade is something we must confront.
These AIs will be both quantitatively and qualitatively superhuman, processing information at unprecedented speeds and generating new solutions beyond human comprehension.
It’s possible that in just 10 years, AI will be so complex and superintelligent that we won’t even be able to understand how it works.
We will be like kindergarteners trying to grasp a PhD in mathematics.
It is better to position yourself to benefit from the dramatic advancements in robotics, scientific progress, and economic growth.
Economic growth rates could exceed 30% per year, similar to those seen during the Industrial Revolution.
Superintelligence will bring huge advantages to those at the forefront, disrupt governments, and transform both society and the economy.
But buckle up, because the leap to AI superintelligence will create a very volatile period in our world. This period could be one of the most dangerous in history, marked by rapid changes and little time for critical decisions.
You must brace for impact. Perhaps everything will go smoothly; perhaps not. Either way, please prepare yourself.
If we’re lucky, AI might just solve all our problems. If not, well… at least we’ll have self-driving cars to take us to the unemployment line.
Profit from the AI Industrial Revolution
To prepare for this AI industrial revolution, it’s important to position your investments to benefit from AI and technology. Many have people have most of my portfolio allocated to cryptocurrencies, tech stocks, robotics, and tech ETFs.
To fuel the AI revolution, there will be enormous demand for hardware; therefore, being a shareholder in GPU-related companies can’t go wrong. However, if you want to diversify further, go for the ETFs.
Over the coming few years, we will need 10 to 100 times more computing power and techno-optimists, believe there’s a very high chance that AI progress will benefit humanity.
Still, given the ultra-fast AI developments toward AGI and superintelligence, most people will be caught by surprise.
The key is to position yourself to understand and invest in these technologies — this is exactly why we write about and share our knowledge of AI, investing, tech, and personal growth.
We can’t be passive in a world evolving at lightning speed. Whether you like it or not — AI progress is coming — and you need to prepare actively.
Comment and Share:
Are you excited or worried about the AI revolution? Share your thoughts and experiences with AI and AGI technologies. Don’t forget to subscribe for updates on AI and AGI developments and comment on the article below.
- You may also like:
To learn more about the AI revolution, tap here.
Author
Discover more from AIinASIA
Subscribe to get the latest posts sent to your email.
Life
Predicting Fetal Well-being with Deep Learning
AI fetal monitoring using deep learning models for CTG interpretation improves neonatal outcomes and reduces provider burden.
Published
2 weeks agoon
October 4, 2024By
AIinAsia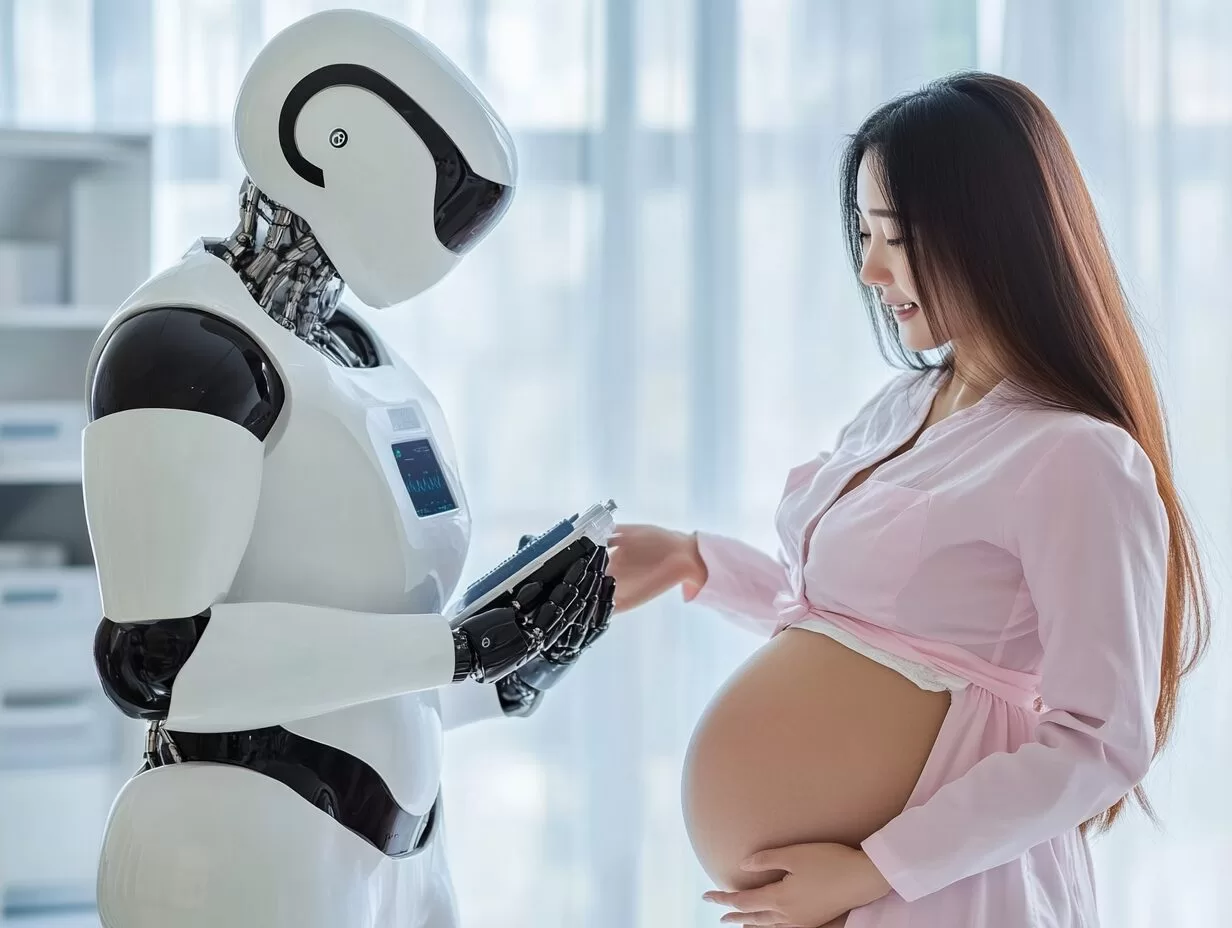
TL;DR:
- AI models can predict fetal well-being using cardiotocography (CTG) signals.
- Deep learning methods improve CTG interpretation, reducing false-positive rates.
- Combining fetal heart rate (FHR) and uterine contractions (UC) data enhances model performance.
The Future of Fetal Monitoring
Imagine a world where artificial intelligence (AI) can accurately predict the well-being of a fetus during pregnancy and labour. This is no longer a dream; it’s a reality. Recent advancements in AI and deep learning are revolutionising the field of fetal monitoring, offering the potential to improve neonatal outcomes and reduce the burden on healthcare providers. In this article, we delve into the groundbreaking work on developing and evaluating machine learning models for cardiotocography (CTG) interpretation.
Understanding Cardiotocography (CTG)
Cardiotocography (CTG) is a crucial technique used during pregnancy and labour to monitor fetal well-being. It involves recording the fetal heart rate (FHR) and uterine contractions (UC) using doppler ultrasound. CTG can be done continuously or intermittently, with sensors placed externally or internally.
Currently, healthcare providers interpret CTG recordings using guidelines from organisations like the National Institute of Child Health and Human Development (NICHD) or the International Federation of Gynecologists and Obstetricians (FIGO). These guidelines define patterns in CTG and FHR traces that may indicate fetal distress.
The Role of AI in Improving CTG Interpretation
Despite its widespread use, CTG interpretation is complex and subjective, leading to high false-positive rates and intra- and inter-observer variability. This is particularly challenging in low-resource settings where access to skilled interpreters is limited.
Enter AI. Recent research has focused on using deep learning methods to improve CTG interpretation. These methods use physiological time series data as input, offering a more comprehensive analysis compared to traditional feature extraction techniques.
Developing and Evaluating Deep Learning Models for CTG
In a recent paper titled “Development and evaluation of deep learning models for cardiotocography interpretation,” researchers developed end-to-end neural network-based models to predict measures of fetal well-being. These models were trained on an open-source CTG dataset, the CTU-UHB Intrapartum Cardiotocography Database, which includes 552 FHR and UC CTG signal pairs.
Model Architecture
The researchers began with the CTG-net network architecture, which convolves the paired FHR and UC input signals temporally before conducting a depthwise convolution to learn the relationship between them. They added several methodological configurations, including architecture and hyperparameter optimization, single input variation, and the addition of clinical metadata.
Pre-processing and Pre-training
To improve data quality, the researchers created a pre-processing pipeline that included inputting missing measurements, random cropping, and additive multiscale noise for data augmentation and downsampling. This generated a large dataset for pre-training and training the models.
Intermittent versus Continuous CTG Use Cases
CTG use comes in two primary formats: intermittent and continuous. In high-resource settings, continuous CTGs are used to monitor fetal heart rate throughout labour. In low-resource settings, intermittent CTGs are often used, covering only about 30 minutes at any point during labour.
The researchers simulated intermittent settings by splitting the 90-minute signals in the dataset into 30-minute signals and training and evaluating the model at different time points. This helped understand how training and evaluating on intermittent time points impacts model performance.
Predicting Objective and Subjective Ground Truth Labels
The researchers used three outcome labels from the dataset:
- Arterial umbilical cord blood pH: An objective measurement that tracks fetal acidosis, an indication of fetal distress.
- Apgar score: A subjective measure recorded by a clinician after delivery that reflects the general health of the newborn.
- Abnormal label: If either Apgar or pH results were abnormal.
Evaluating Model Prediction Robustness
The researchers performed several comparisons to evaluate model performance, including:
- Performance on the dataset versus the state-of-the-art CTG-net model.
- Apgar versus pH classification tasks.
- FHR-only versus FHR+UC.
- Base model using the last 30 minutes of labour (continuous case) versus intermittent measurements.
- Base model of FHR+UC versus FHR+UC+Metadata.
- Subgroup performance of the base model (FHR+UC) with subgroups determined by binarizing clinical metadata.
The results showed that combining FHR+UC achieved the highest model performance for both pH and Apgar classification. The pre-training step enabled the highest model performance, and adding clinical metadata slightly improved model performance for pH but less so for Apgar.
Subgroup Evaluations
The researchers found significant differences in baseline performance between subgroups with frequent and infrequent UC signals gaps for pH prediction and for subgroups with frequent and infrequent FHR signal gaps for Apgar prediction. With metadata, the performance disparities observed with pH prediction were mitigated. However, including metadata increased the AUROC performance disparities for demographic and clinical-related subgroups on this task.
Open CTG Model for Research Use Cases
The researchers are currently exploring open-sourcing their models, hoping that other researchers and stakeholders can build on this work with their own datasets to evaluate it for their clinical use cases.
Limitations and Future Work
The study had limitations that constrain the generalizability of the findings. Future investigations should involve a larger and more diverse dataset sourced from maternity centers worldwide, encompassing varied clinical contexts, demographics, and outcomes. Additionally, further work is needed to understand how such prediction algorithms can be optimally integrated into clinical workflows to improve neonatal outcomes.
The Promise of AI in Fetal Monitoring
The development and evaluation of deep learning models for CTG interpretation hold immense promise for improving fetal monitoring and neonatal outcomes. By leveraging AI, healthcare providers can gain objective interpretation assistance, reducing the burden and potentially improving fetal outcomes.
Comment and Share:
What are your thoughts on the future of AI in fetal monitoring? Do you think AI can revolutionise the field and improve neonatal outcomes? Share your experiences and thoughts in the comments below. Don’t forget to subscribe for updates on AI and AGI developments and join the conversation!
- You may also like:
- AI Takes Aim at Skin Cancer: DermaSensor Gets FDA Nod
- How AI and Wearables are Transforming Medicine
- Revolutionising Healthcare: Malaysia’s First AI-Detected Lung Cancer Success Story
- To learn more about AI and fetal medicine, tap here.
Author
Discover more from AIinASIA
Subscribe to get the latest posts sent to your email.

Adrian’s Arena: East Meets West – Contrasting AI Partnership Strategies for OpenAI

AI Revolution at Wimbledon: 300 Jobs at Risk as Tradition Fades

Grindr’s AI Wingman: Revolutionising Dating for the LGBTQ+ Community
Trending
-
Marketing2 weeks ago
Adrian’s Arena: How AI is Reshaping Industries and Shaping Our Future
-
Business19 hours ago
Adrian’s Arena: East Meets West – Contrasting AI Partnership Strategies for OpenAI
-
Tools2 weeks ago
The Truth About OpenAI’s o1: Is It Worth the Hype?
-
Tools2 weeks ago
ChatGPT’s New Voice: A Revolution in AI, but at What Cost?
-
Business2 weeks ago
Revolutionising Critical Infrastructure: How AI is Becoming More Reliable and Transparent
-
Business2 weeks ago
AI Coding Assistants: The Future of Software Development
-
Tools2 weeks ago
Mistral’s Pixtral 12B and the Future of Multimodal Models
-
Tools2 weeks ago
Revolutionising Video Creation: Adobe’s Upcoming Generative AI Tools