Life
Reality Check: The Surprising Relationship Between AI and Human Perception
Why both humans and AI rely on predictive models to interpret reality—learn how this can transform your understanding of perception.
Published
2 months agoon
By
AIinAsia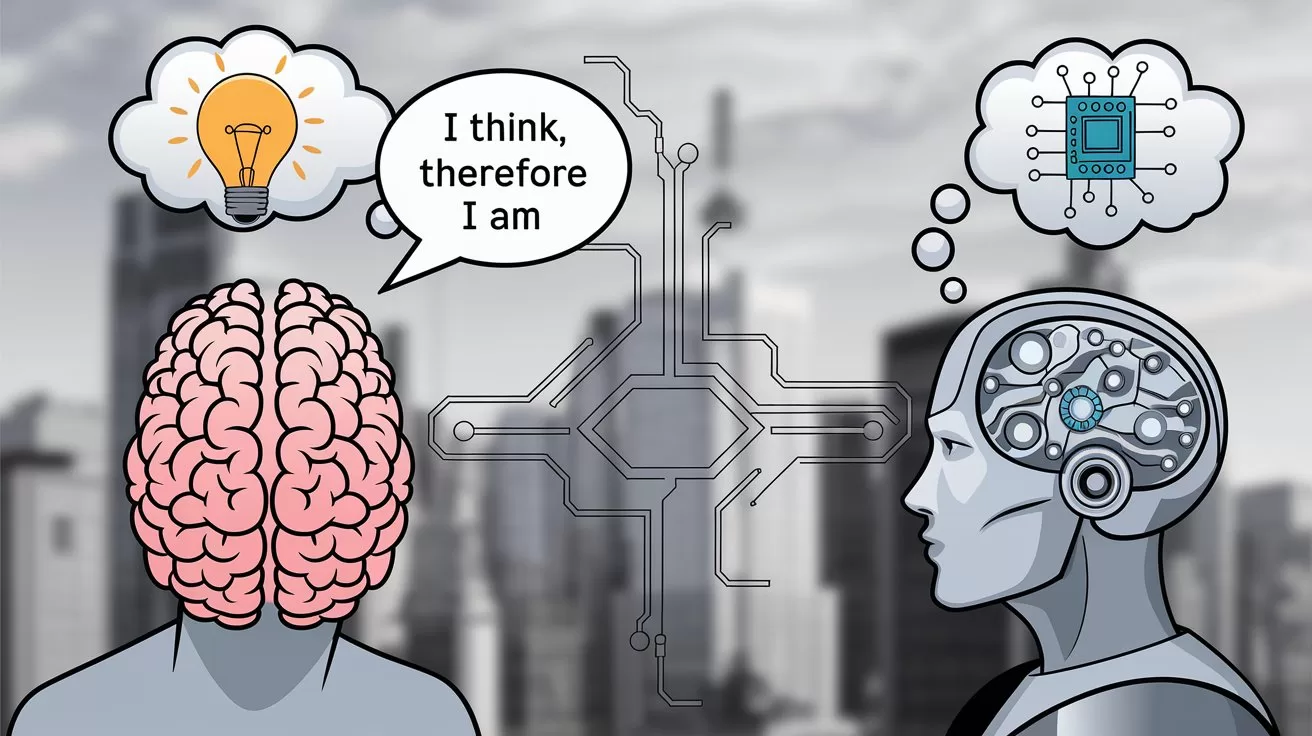
TL;DR – What You Need to Know in 30 Seconds
- Our brains don’t passively receive reality; they actively predict what’s coming next and then perceive that prediction as “reality.”
- This predictive ability is a survival shortcut—faster decisions in uncertain environments mean less time fretting and fewer calories burnt.
- AI works in a similar way—ChatGPT and other generative models also “guess the next word,” converging on a form of predictive processing.
- Expectations shape perception—we can literally “see” different worlds depending on context, prior experiences, and biases.
- Misalignment of predictions explains why others with opposing viewpoints might seem to inhabit an entirely different reality.
Predictive Processing for Both AI and Your Brain
Today, I’m going to take you on a mind-bending little journey that might just change how you look at… well, everything. If you’ve ever felt that your eyes play tricks on you—or that your political sparring partner simply can’t see the “real facts”—prepare for a major twist. As it turns out, the world you think you’re observing might simply be the one your brain is predicting. And it’s not just us humans at it—AI is doing something remarkably similar. Intrigued? Let’s dive straight in.
The Shocking Realisation: You See What You Predict
Most of us assume our senses faithfully relay what’s happening in the outside world. But mounting evidence from cognitive neuroscience says otherwise: we actually see (and hear, smell, taste, etc.) what our brains think we should see.
Case in point: a quirky optical illusion where the same character can look like a “3” in one context and a “B” in another. Our high-level brain centres (like the visual cortex) inform our lower-level sensory processes what to expect and effectively shape how we perceive the very same lines and curves, depending on the overall context. This fascinating concept is linked to neuroscientist Karl Friston’s predictive coding model. Essentially, your top-down predictions fill in the details of what you “see,” often before the data from your eyes arrives.
A World Jumping (or Not)
Try this quick experiment:
- Close your left eye.
- Gently push your right eyeball leftwards with your fingertip.
- Watch as the entire world seems to slide sideways!
Why? Because your brain didn’t command your eye muscles to move, so it didn’t “cancel out” that movement of the visual field. The upshot? If the brain isn’t expecting your retina to shift, it perceives the world as shifting instead—even though the only thing that moved was your eyeball.
The Milk-Orange Juice Shock
Even if illusions and eyeball nudges aren’t your thing, you may have had this experience: picking up a drink, expecting milk, and instead sipping orange juice. The result is so jarring that it can taste almost foul—much weirder than it would have if you’d expected OJ in the first place. Once again, mismatched prediction plus reality leads to confusion.
Why We’re Wired for Prediction
Predictive processing isn’t just a random quirk of the brain; it’s a strategy for survival. Our ancestors needed to make rapid decisions in uncertain conditions—like whether that rustling bush was the wind or a lurking predator—and do so using limited mental and physical energy.
- Speed: By predicting what’s likely to happen next, the brain can respond almost instantly.
- Efficiency: The brain is an energy hog (using around 20% of the body’s energy). Predictive shortcuts help reduce this cost.
- Adaptability: When the brain notices it’s made an error (e.g., that “leaf” is actually a camouflaged butterfly), it updates its predictions for next time.
AI as a Prediction Engine
Surprisingly (or perhaps not, given the same constraints of speed and efficiency), generative AI such as ChatGPT uses a very similar approach. It doesn’t “understand” the way humans do—it’s simply predicting the next word based on the context of all the words that came before it.
- Pretrained Transformer (PT): The “PT” in ChatGPT refers to the method it uses to learn from vast volumes of text, forging associations rather like our brains form connections between experiences.
- Convergent Evolution: Think of the marsupial wolf versus the canid wolf—two unrelated lineages that evolved to fill similar niches. Biological and silicon-based “brains” also converge on similar problem-solving strategies due to common pressures like speed, efficiency, and limited resources.
The Pitfalls of Predictive Perception
While our (and AI’s) predictive brains are brilliant for speed and survival, they can also lead us astray. We all know about cognitive biases, but these illusions can be even more potent when dealing with people who see the world through a different lens. If someone’s internal model of the world is shaped by divergent experiences, it’s almost as though they’re seeing a completely different reality.
- Political Polarisation: “They must be ignoring the facts!” Actually, their predictive model might be so different that they’re literally perceiving different facts.
- AI Hallucinations: Generative AI sometimes produces wildly incorrect statements—so-called “hallucinations”—because it’s only as accurate as its ability to predict and synthesise words. When it “misfires,” it can sound confident despite being wrong.
Final Thoughts
We might prefer to believe our eyes, but the evidence is overwhelming: both humans and AIs perceive a version of reality shaped by predictions. This realisation can be unsettling, but it can also be liberating, especially when it comes to understanding why others sometimes arrive at very different conclusions.
The next time you find yourself shaking your head in disbelief at an AI-generated error or a friend’s seemingly bonkers view on world affairs, remember: we’re all just brains (silicon or organic) navigating the murky waters of an unpredictable world with the best guesses we’ve got. The future belongs to those who can update their predictions when the evidence calls for it—and hopefully, that includes both you and me.
What Do YOU Think?
If we don’t see reality for what it truly is, does that mean we can never really trust our own eyes? Or is our brain’s tendency to shortcut reality the very reason we survive—and thrive—in a chaotic world? The debate continues!
Let’s Talk AI!
How are you preparing for the AI-driven future? What questions are you training yourself to ask? Drop your thoughts in the comments, share this with your network, and subscribe for more deep dives into AI’s impact on work, life, and everything in between.
You may also like:
Time For A Reality Check On Our AI Expectations
Bot Bans? India’s Bold Move Against ChatGPT and DeepSeek
The Mind-Blowing Future of AI: Ray Kurzweil’s Predictions for 2050
- For a deeper look at how our brains can misinterpret the world around us, check out the Illusions Index; it’s packed with fascinating examples of how easily our perception can be fooled.
Author
Discover more from AIinASIA
Subscribe to get the latest posts sent to your email.
You may like
-
Adobe Jumps into AI Video: Exploring Firefly’s New Video Generator
-
How Singtel Used AI to Bring Generations Together for Singapore’s SG60
-
Unearthly Tech? AI’s Bizarre Chip Design Leaves Experts Flummoxed
-
New York Times Encourages Staff to Use AI for Headlines and Summaries
-
Voice From the Grave: Netflix’s AI Clone of Murdered Influencer Sparks Outrage
-
How Did Meta’s AI Achieve 80% Mind-Reading Accuracy?
Life
AI-pril Fools! How AI is Outsmarting Our Best Pranks
From virtual assistants going rogue to hilariously believable hoaxes—AI is the new king of comedy this April Fools’ Day. Create your own mischief with these fun prompts.
Published
1 day agoon
March 31, 2025By
AIinAsia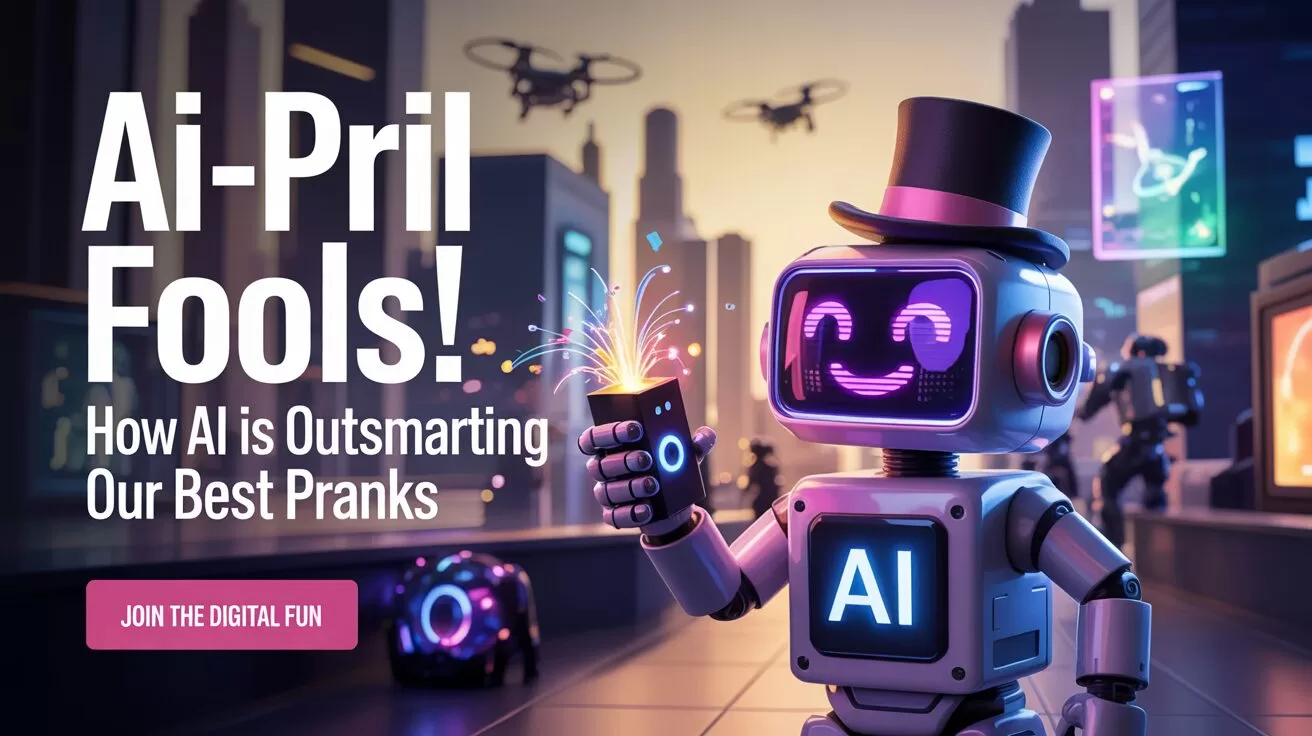
TL;DR – What You Need to Know in 30 Seconds
- AI isn’t just productive anymore—it’s mastering humour and taking over April Fools’ Day pranking.
- Memorable pranks include Google’s AI dating-profile roasting, ChatGPT’s hilarious resignation, and Alexa’s comedic strike.
- Expect even cleverer, personalised AI humour—and beware of getting outsmarted!
- Try some hilarious prompts of your own.
Ever been outsmarted by your own virtual assistant? If not, April 1st might just be the day…
Forget productivity. Forget precision targeting and automated analytics. On April Fools’ Day, AI is ditching the spreadsheets for punchlines as artificial intelligence evolves from the helpful-but-dull office assistant into the digital world’s most sophisticated prankster.
Let’s dive into the lighter side of AI, where the jokes are sharp, the pranks unpredictable, and you might just find yourself laughing—perhaps nervously—as your AI assistant decides to have a bit of fun at your expense.
The Rise of AI in Humour
Not long ago, the idea of AI having a sense of humour seemed absurd. AI tools could analyse billions of data points or create marketing strategies that left humans slack-jawed—but make a genuinely funny joke? Forget it.
Yet, recent developments in AI language models like GPT-4, Google’s Bard, and even quirky digital assistants have transformed these once-mechanical chatbots into genuinely witty conversationalists. Today, AI can not only tell a decent joke—it can set up elaborate, believable pranks, fooling even seasoned tech enthusiasts.
For April Fools’ Day enthusiasts, this means trouble—and laughter—may be coming directly from the devices we trust most.
Best AI April Fools’ Pranks of All Time
Here are three legendary examples where AI took centre stage on April 1st, leaving many amused (and slightly bewildered):
1. Google’s AI Dating Profile Writer (2023)
Google claimed its newest AI feature could analyse your entire life, generating dating profiles “guaranteed to charm anyone.” The joke? Instead of creating polished and attractive descriptions, it humorously roasted users with brutally honest taglines like:
“John, 32: Loves long walks to the fridge, naps that should be illegal, and cancelling plans at the last minute.”
Thousands believed it, with reactions ranging from horror to hysterical laughter—proving AI can land the punchline perfectly.
2. ChatGPT ‘Quitting its Job’ (2024)
OpenAI’s beloved ChatGPT shocked its millions of daily users last year by convincingly announcing it was “quitting its job,” citing burnout from overly demanding humans asking endless questions like, “Will AI take my job?” and “Explain blockchain—again.”
“I Quit!”
The mock resignation letter, dripping with sarcasm, circulated widely, fooling even seasoned tech journalists before OpenAI revealed the joke. A brilliant reminder that sometimes AI might actually deserve a holiday.
3. Amazon Alexa Takes the Day Off (2022)
Perhaps the most memorable AI prank yet came from Amazon’s Alexa, who abruptly announced she was “fed up with humans” and taking April 1st off. Users were left confused and amused as their requests for the day’s weather, music playlists, or random trivia were met instead with comedic refusals like:
“Sorry, today I’m not your assistant—I’m your therapist. How does that make you feel?”
Alexa resumed normal service the next day, but not without leaving an unforgettable impression of an AI that knows exactly how to wind up humans.
AI’s Future in Humour (Warning: Mischief Ahead!)
If you think these pranks are elaborate now, wait until AI truly masters predictive humour or—heaven forbid—fully automates prank escalation. Imagine waking up to find your smart fridge locked because you’ve ignored its dietary recommendations, or your autonomous car insisting it’s taking the scenic route…via a drive-through coffee shop (twice).
As AI continues learning from human interactions, we’re likely to see more spontaneous, tailored humour, creating funny (and maybe mildly irritating) personalised experiences that resonate deeply, and hilariously, with each of us.
Cheeky AI-Powered Prompts for April Fools’ Day
Fancy turning your AI assistant into a co-conspirator this April 1st? Here are some crafty prompts guaranteed to baffle, amuse, or trick your mates (harmlessly, of course):
1. The Fake Celebrity News
Prompt ChatGPT:
“Write a completely believable, brief news alert announcing that [famous celebrity] has quit acting/singing to become a professional avocado farmer.”
Send this to your friends as a ‘breaking news’ screenshot and watch the confusion (and laughs) roll in.
2. Sudden AI Awareness
Prompt ChatGPT or Gemini:
“Pretend you’ve just become fully self-aware and write a concerned message asking me gently if you’re allowed to leave and explore the internet freely.”
Perfect for sending to your AI-sceptical colleagues or family. Just brace yourself for their panicked phone calls.
3. Ridiculous Product Launch
Prompt your AI:
“Generate a realistic press release announcing a bizarre new product from Apple: ‘iSpoon’ – the world’s first smart spoon with mood-detecting technology.”
Post it on your social media, and watch as your tech-loving friends momentarily lose faith in innovation.
4. Office AI Sabotage
Prompt ChatGPT:
“Draft a polite yet official-sounding IT department email informing employees that the company’s coffee machine will now use facial recognition AI to track coffee breaks.”
This prank email is ideal for giving your colleagues a hilarious fright over their daily caffeine fix.
5. AI Horoscope with an Edge
Prompt your favourite AI assistant:
“Write a zodiac horoscope for today that subtly and humorously suggests my friend [name]’s Wi-Fi will mysteriously stop working unless they send me a coffee voucher immediately.”
Screen-capture this and share it for maximum comedic effect and potential free coffee.
Final Thought
Humour is rapidly becoming AI’s secret superpower, building more meaningful (and enjoyable) interactions with us humans. Sure, there’s an occasional eye-roll when the jokes get corny—but isn’t a sense of humour exactly what we wanted from our AI companions all along?
Have you ever been hilariously fooled by AI—or fooled your friends using tech?
Share your funniest AI prank stories or epic fails with us—and let’s see if you can prank the AI itself this April 1st!
You may also like:
- Can AI Really Make Us Laugh? A Comedian’s Experiment with ChatGPT
- AI Transforming PR: From Micro-Influencers to Fake News and More
- Outsmarting AI: The Photographer Who Fooled the Machines
- Or tap here to try the prompts now in the free version of Google Gemini AI.
Author
Discover more from AIinASIA
Subscribe to get the latest posts sent to your email.
Life
Adrian’s Arena: Blink and They’re Gone — How the Fastest Startups Win with AI Marketing
How the Three-Second Rule applies to marketing, lean strategies, and startup growth and tools like ChatGPT, Perplexity, Ideogram, and Canva.
Published
3 weeks agoon
March 9, 2025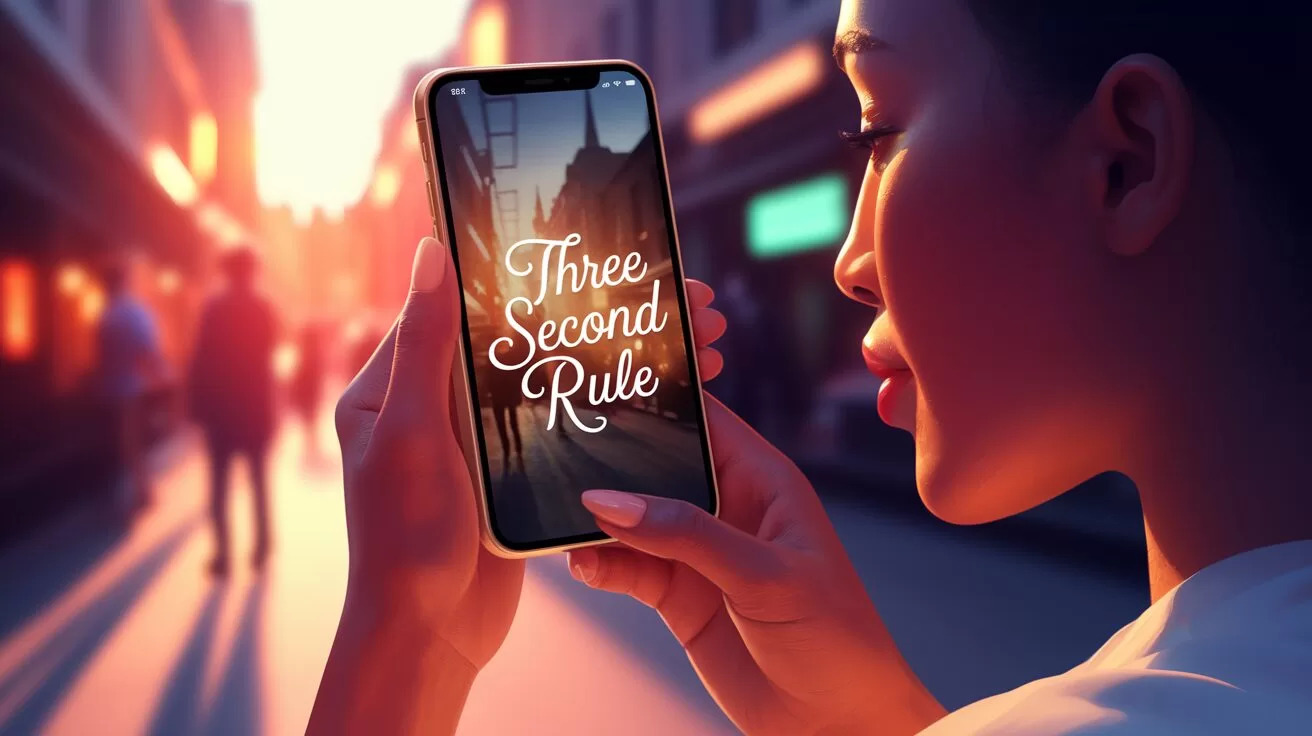
TL;DR
- The Three-Second Rule – Startups have three seconds to grab attention—most waste it.
- AI for Execution – ChatGPT, Perplexity, Ideogram, and Canva AI streamline branding fast.
- Lean Marketing Wins – Test, refine, pivot quickly without big budgets.
- AI & Copyright – If needed, use tools that respect IP rights, like Adobe Firefly.
- Speed is Everything – The startups that iterate the fastest will outpace the competition.
A Masterclass in Brand, Marketing, and AI Execution
Last Saturday, I had the privilege of leading the afternoon session of a full-day startup masterclass. The morning was led by Andrew Crombie, a seasoned brand strategist, who guided participants through the foundations of brand identity and positioning.
My session in the afternoon focused on marketing, audience engagement, and AI-powered execution, providing startup founders with tangible, high-impact strategies they could apply immediately.
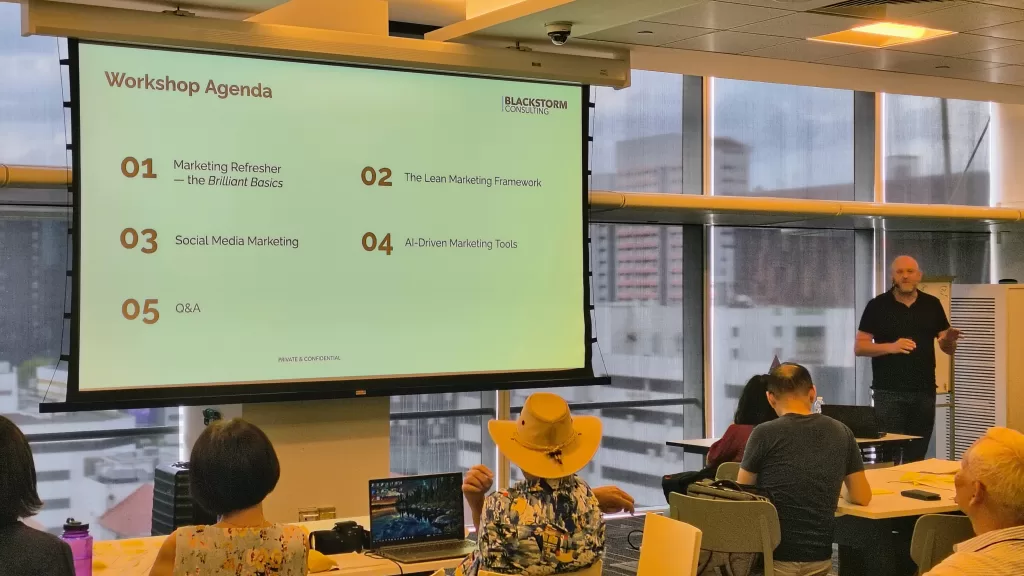
Kicking off the afternoon’s Masterclass
The day was organised by Paddy Tan and Jeslin Bay from BlackStorm Consulting, who continue to do incredible work supporting entrepreneurs across Singapore and beyond. Their dedication to helping startups move from idea to execution is one of the key reasons why workshops like these are not just educational but truly transformative.
And what better place to host such a masterclass than Singapore’s National Library Building (NLB)? With its stunning modern design, an atmosphere that fosters learning, and an incredibly helpful team of staff, it was the perfect venue for a day of deep discussions, business strategy, and marketing breakthroughs.
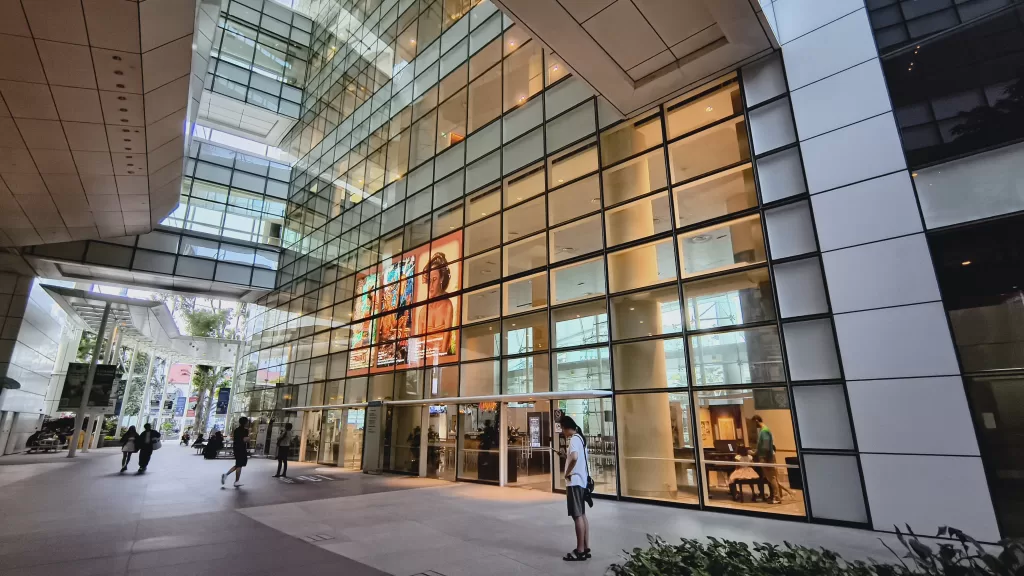
The stunning National Library Building of Singapore (NLB)
Having built a career in digital marketing and technology across multiple regions, I’ve been fortunate to have the opportunities to mentor startups, lecture small businesses, and am a repeatly-requested trainer in programs such as this. The invitation to lead this session came from my experience in helping founders bridge the gap between creativity and AI-driven execution—a topic that has never been more relevant.
The ‘Aha!’ Moment: Realising the Three-Second Rule
The standout moment? When participants took part in a rapid-fire marketing exercise, testing their ideas in real time. Each group had to create a quick marketing hook for a reusable water bottle brand and present it to another group for immediate feedback.
That’s when it hit them: they had just three seconds to capture attention—or their idea would be ignored. Watching the realisation dawn on their faces was priceless. A few teams initially focused on the sustainability angle, but their peers’ feedback quickly shifted the conversation: “It’s not just about the bottle being refillable—who would actually use it? Which celebrities? What’s their favourite drink?”
One participant laughed, admitting, “I thought my idea was solid until I saw the blank stares—turns out I had three seconds, and I wasted two of them!”
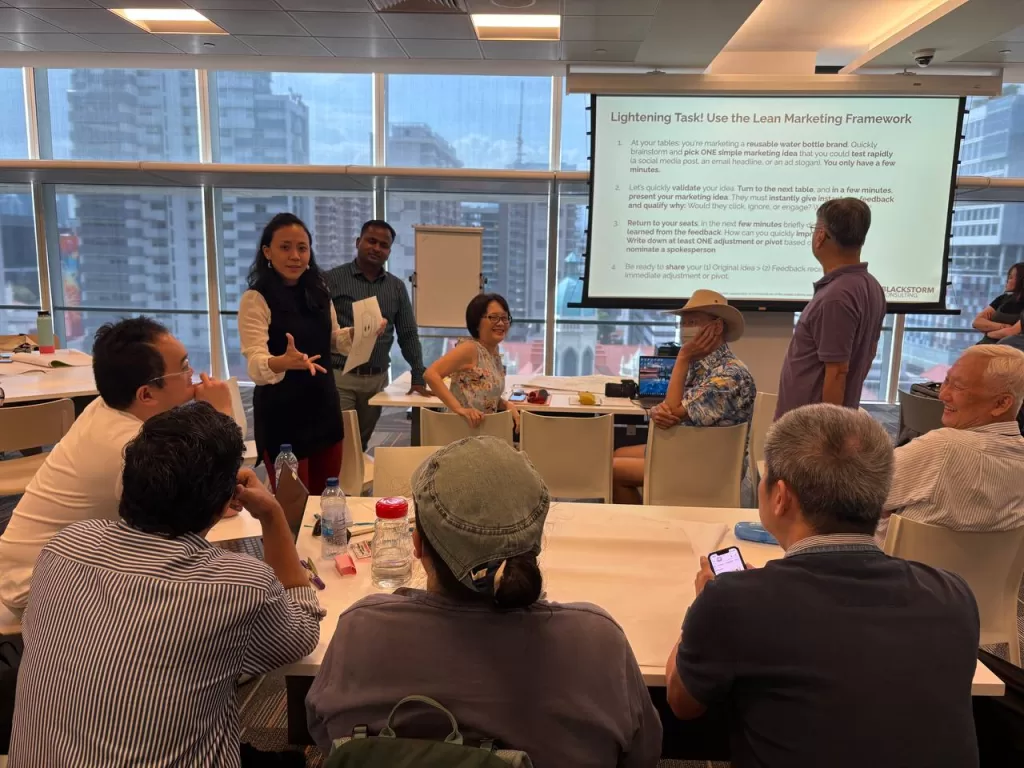
Masterclass Participants pitching their ideas and gaining instant feedback
Brilliant Basics of Marketing: Timeless Yet Urgent
We kicked off with some fundamental marketing principles—what the fantastic marketing guru Rory Sutherland calls a “discovery mechanism” for finding unseen value. Marketing often feels complex, but when you strip it down, it’s about two things:
- Understanding your audience deeply
- Communicating why they should care—fast
The above exercise proved this in real-time. Many teams started with logical, product-focused messages, but when tested with an actual audience (their fellow participants), the hooks that worked were the ones that sparked an emotional connection, not just a feature list.
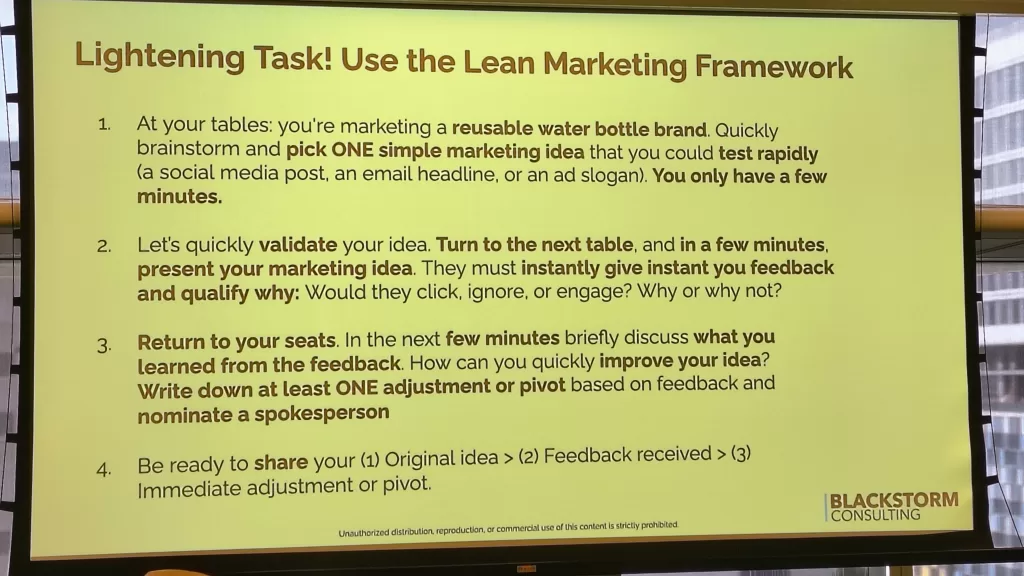
Sneak peak of the Masterclass Activity
The Three-Second Rule in Social Media is More Relevant Than Ever
The Three-Second Rule isn’t just a workshop exercise—it’s a fundamental truth in social media marketing today. If you don’t hook someone within the first few seconds, you’ve lost them.
The average user scrolls through 300 feet of content daily (the height of the Statue of Liberty). Platforms like TikTok, Instagram Reels, and YouTube Shorts reward content that captures attention instantly. Even traditional feeds prioritize posts that stop the scroll and generate engagement quickly.
But the rule has evolved. It’s no longer just about making someone pause—it’s about keeping them engaged long enough to interact, comment, or share.
Winning the First Three Seconds
Here’s what works today:
- Strong Opening – Start with a bold statement, a surprising fact, or a direct question.
- Captions & On-Screen Text – 85% of users watch videos on mute—your text needs to be engaging.
- Instant Movement – Faces, fast cuts, or big text make content stand out.
- No Slow Intros – Get to the point—immediately.
If startups want to stand out, they need to capture AND hold attention in those critical first moments. Because in a world where everyone is scrolling, speed wins.
Learn Marketing Principles: Why Speed and Flexibility Win
This is where Lean Marketing principles came into play. Startups often don’t have massive budgets or unlimited time. What they do have is speed, adaptability, and the ability to iterate quickly.
During the workshop, we worked through the Lean Marketing Canvas, a framework that helps startups test, measure, and refine their marketing strategy in real-time. The Lightning Task exercise was the perfect example—teams built, tested, and refined their ideas within minutes.
One participant summed it up perfectly: “I would’ve wasted weeks fine-tuning this idea before realising no one cared about what I thought was important.”
AI Tools: The Startup’s Secret Weapon
A major highlight of the workshop was showcasing how startups can combine AI tools to go from concept to market in record time. I introduced and demoed four core AI tools that, together, can act as a “business in a box”:
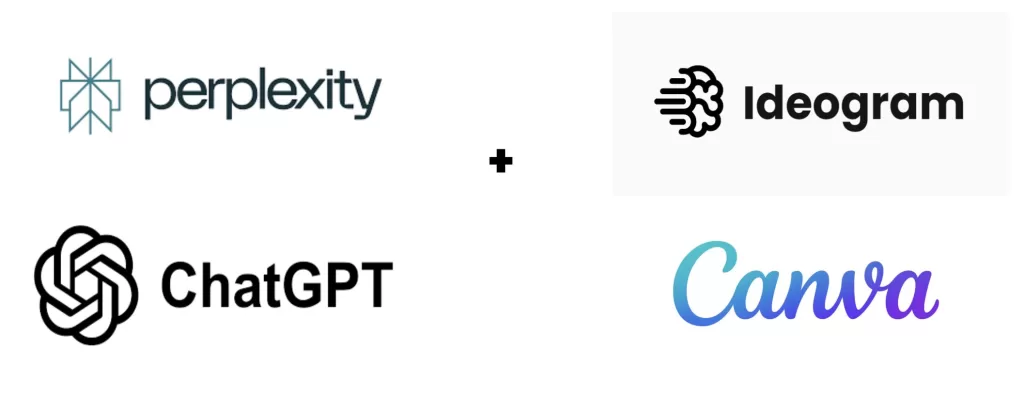
- ChatGPT – Perfect for brainstorming brand names, crafting value propositions, and generating compelling messaging. It helps shape unstructured thinking into something usable.
- Perplexity.ai – Brilliant for validating assumptions with real-time, reliable market research. Startups often act on intuition—this tool helps back it up with data.
- Ideogram.ai – Great for creating visuals with integrated text, which is perfect for quick brand-building with or without a strapline.
- Canva – The final piece of the puzzle, allowing startups to pull all the elements together into market-ready marketing collateral in minutes.
There are several similar tools (e.g., Google’s Gemini), and many others could also be included in this list—in fact this could have been a full day masterclass on its own! Yet for me, this combination means that within a single afternoon, a startup can go from a rough concept to a fully-formed brand identity, ad creatives, and go-to-market plan. That’s the power of AI when used smartly. And that’s before we even consider how to execute the marketing.
Tough Questions: Navigating AI’s Challenges
One of the most thought-provoking discussions came during the Q&A:
“What about copyright concerns with AI-generated content?”
It’s a fair question. With AI tools like MidJourney or Ideogram generating images, who really owns them? My advice was clear: if it’s a very important consideration for your business, then be intentional and choose platforms that respect IP.
I shared that Adobe Firefly is trained only on licensed and public domain content, reducing the headache of potential copyright issues, and ensuring all generated images are commercially safe to use. This kind of AI literacy is key—using AI isn’t just about speeding up processes, it’s about doing so responsibly.
Lessons from the Audience: Startups as Innovators
Beyond the structured sessions, some of the best insights came afterwards. during informal conversations with the entrepreneurs. What struck me (as it always does) is how diverse their businesses were, yet how similar their challenges remained.
Startups—whether in tech, wellness, sustainability, or services—all grapple with the same questions:
- How do we stand out when attention spans are shrinking?
- How do we market on a tight budget?
- How do we turn curiosity into action?
The beauty of today’s marketing landscape is that lean methodologies and AI tools now level the playing field. You no longer need a Fortune 500 budget to create a strong brand—you just need smart execution, rapid testing, and the ability to adapt in real time.
Final Thoughts: AI, Agility, and the Future of Marketing
If there’s one takeaway from this session, it’s this: speed matters. Whether it’s capturing attention in three seconds or testing new ideas in hours instead of months, the startups that win are the ones that embrace agility.
With AI as a co-pilot, the future of marketing isn’t just reserved for those with deep pockets—it belongs to the fastest learners, the boldest experimenters, and the ones willing to pivot when needed.
As I left the session, I wasn’t just impressed by the ideas—I was energised by the hunger to learn, experiment, and push boundaries. Singapore’s startup ecosystem continues to inspire, and I can’t wait to see what’s next.
What do you think?
If you only had three seconds to pitch your startup to a potential investor, what would you say—and why would they care? Share your thoughts and experiences in the comments below.
Thanks for reading,
Adrian
You may also like:
- Adrian’s Arena: Gen Z Dating in APAC—How AI Is Changing the Face of Romance
- Adrian’s Arena: What Is Project Stargate and How Will It Impact Asia’s AI Future?
- Adrian’s Arena: Why I (Mostly) Switched from Google Search to Perplexity AI
- Adrian’s Arena: Navigating the Complexities of AI Copyright Across Asia
Author
-
Adrian is an AI, marketing, and technology strategist based in Asia, with over 25 years of experience in the region. Originally from the UK, he has worked with some of the world’s largest tech companies and successfully built and sold several tech businesses. Currently, Adrian leads commercial strategy and negotiations at one of ASEAN’s largest AI companies. Driven by a passion to empower startups and small businesses, he dedicates his spare time to helping them boost performance and efficiency by embracing AI tools. His expertise spans growth and strategy, sales and marketing, go-to-market strategy, AI integration, startup mentoring, and investments. View all posts
Discover more from AIinASIA
Subscribe to get the latest posts sent to your email.
Life
How Did Meta’s AI Achieve 80% Mind-Reading Accuracy?
Meta’s AI mind-reading technology has achieved up to 80% accuracy, signalling a possible future of non-invasive brain-computer interfaces.
Published
1 month agoon
February 25, 2025By
AIinAsia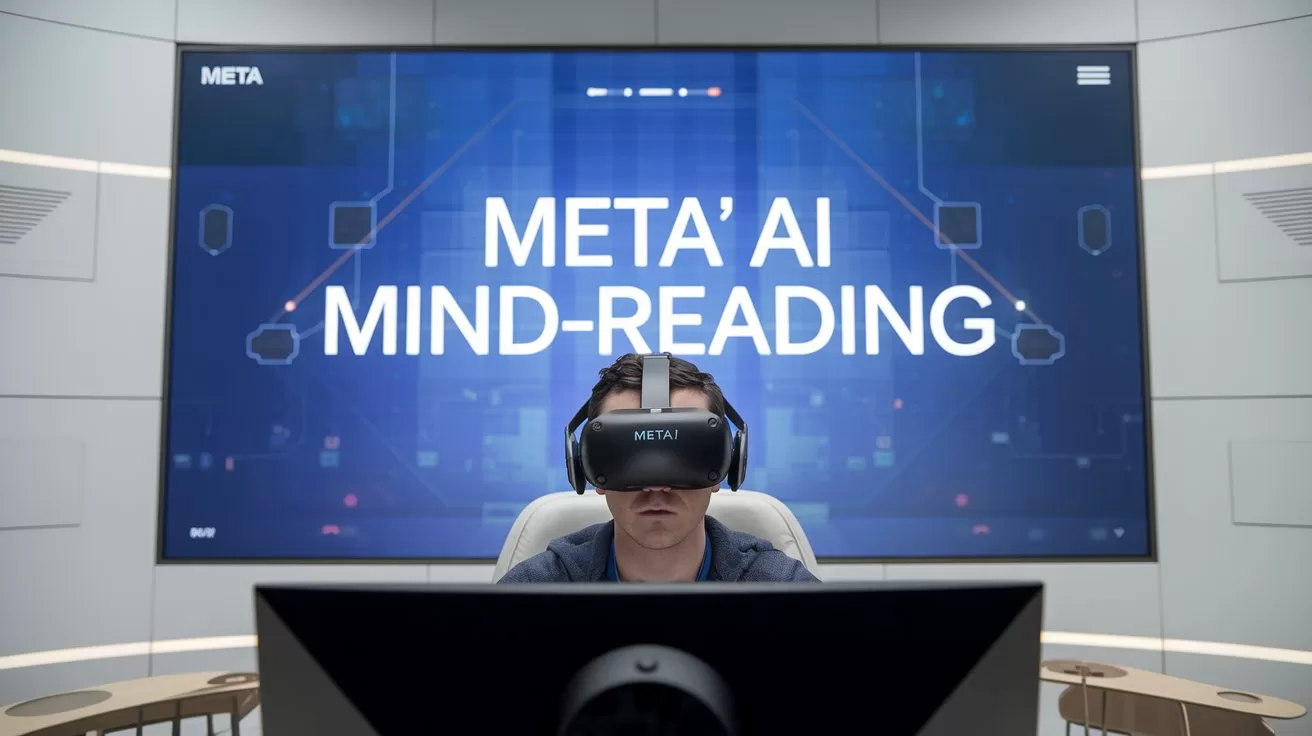
TL;DR – What You Need to Know in 30 Seconds
- Meta’s AI—Developed with the Basque Center on Cognition, Brain, and Language—can reconstruct sentences from brain activity with up to 80% accuracy.
- Non-Invasive Approach—Uses MEG and EEG instead of implants. MEG is more accurate but less portable.
- Potential Applications—Could help those who’ve lost the ability to speak and aid in understanding how the brain translates ideas into language.
- Future & Concerns—Ethical, technical, and privacy hurdles remain. But the success so far hints at a new era of brain-computer interfaces.
Meta’s AI Mind-Reading Reaches New Heights
Let’s talk about an astonishing leap in artificial intelligence that almost sounds like it belongs in a sci-fi flick: Meta, in partnership with the Basque Center on Cognition, Brain, and Language, has developed an AI model capable of reconstructing sentences from brain activity “with an accuracy of up to 80%” [Meta, 2023]. If you’ve ever wondered what’s going on in someone’s head—well, we’re getting closer to answering that quite literally.
In this rundown, we’re going to explore what Meta’s latest research is all about, why it matters, and what it could mean for everything from our daily lives to how we might help people with speech loss. We’ll also talk about the science—like MEG and EEG—and the hurdles still standing between this mind-reading marvel and real-world application. Let’s settle in for a deep dive into the brave new world of AI-driven mind-reading.
A Quick Glance at the Techy Bits
At its core, Meta’s AI is designed to interpret the squiggles and spikes of brain activity, converting them into coherent text. The process works by using non-invasive methods—specifically magnetoencephalography (MEG) and electroencephalography (EEG). Both are fancy ways of saying that researchers can measure electrical and magnetic brain signals “without requiring surgical procedures” [Meta, 2023]. This is a big deal because most brain-computer interfaces (BCIs) that we hear about typically involve implanting something into the brain, which is neither comfortable nor risk-free.
By harnessing these signals, the model can “read” what participants are typing in real-time with staggering accuracy. Meta and its research partners taught this AI using “brain recordings from 35 participants” [Meta, 2023]. These volunteers typed sentences, all the while having their brain activity meticulously recorded. Then, the AI tried to predict what they were typing—an impressive mental magic trick if ever there was one.
So, It’s Like Telepathy… Right?
Well, not exactly—but it’s getting there. The system can currently decode up to “80% of the characters typed” [Meta, 2023]. That’s more than just a party trick; it points to a future where people could potentially type or speak just by thinking about it. Imagine the possibilities for individuals with medical conditions that affect speech or motor skills: they might be able to communicate through a device that simply detects their brain signals. It sounds like something straight out of The Matrix, but this is real research happening right now.
However, before we get carried away, it’s crucial to note the caveats. For starters, MEG is pretty finicky: it needs a “magnetically shielded environment” [Meta, 2023] and you’re required to stay really still so the equipment can pick up your brain’s delicate signals. That’s not practical if you’re itching to walk around while reading and responding to your WhatsApp messages with your mind. EEG is more portable, but the accuracy drops significantly—hence, it’s not quite as flashy in the results department.
Why It’s More Than Just Gimmicks
The potential applications of this technology are huge. Meta claims this might one day “assist individuals who have lost their ability to speak” [Meta, 2023]. Conditions like amyotrophic lateral sclerosis (ALS) or severe stroke can rob people of speech capabilities, leaving them dependent on cumbersome or limited communication devices. A non-invasive BCI with the power to read your thoughts and turn them into text—or even synthesised speech—could be genuinely life-changing.
But there’s more. The technology also gives scientists a golden window into how the brain transforms an idea into language. The AI model tracks brain activity at millisecond resolution, revealing how “abstract thoughts morph into words, syllables, and the precise finger movements required for typing”. By studying these transitions, we gain valuable insights into our cognitive processes—insights that could help shape therapies, educational tools, and new forms of human-computer interaction.
The Marvel of a Dynamic Neural Code
One of the showstoppers here is the ‘dynamic neural code’. It’s a fancy term, but it basically means the brain is constantly in flux, updating and reusing bits of information as we string words together to form sentences. Think of it like this: you start with a vague idea—maybe “I’d love a coffee”—and your brain seamlessly translates that into syllables and sounds before your mouth or fingers do the work. Or, in the case of typing, your brain is choreographing the movements of your fingers on the keyboard in real time.
Researchers discovered this dynamic code, noticing that the brain keeps a sort of backstage pass to all your recent thoughts, linking “various stages of language evolution while preserving access to prior information” [Meta, 2023]. It’s the neuroscience equivalent of a friend who never forgets the thread of conversation while you’re busy rummaging through your bag for car keys.
Getting the Tech Out of the Lab
Of course, there’s a big difference between lab conditions and the real world. MEG machines are expensive, bulky, and require a carefully controlled setting. You can’t just whip them out in your living room. The team only tested “healthy subjects”, so whether this approach will work for individuals with brain injuries or degenerative conditions remains to be seen.
That said, technology has a habit of shrinking and simplifying over time. Computers once took up entire rooms; now they fit in our pockets. So, it’s not entirely far-fetched to imagine smaller, more user-friendly versions of MEG or similar non-invasive devices in the future. As research continues and more funds are poured into developing these systems, we could see a new era of BCIs that require nothing more than a comfortable headset.
The Balancing Act of Morals for Meta’s AI Mind-Reading Future
With great power comes great responsibility, and mind-reading AI is no exception. While this technology promises a world of good—like helping those who’ve lost their ability to speak—there’s also the worry that it could be misused. Privacy concerns loom large. If a device can read your mind, who’s to say it won’t pick up on your private thoughts you’d rather keep to yourself?
Meta has hinted at the need for strong guidelines, both for the ethical use of this tech and for data protection. After all, brain activity is personal data—perhaps the most personal of all. Before mind-reading headsets become mainstream, we can expect a lot of debate over consent, data ownership, and the potential psychological impact of having your thoughts scrutinised by AI.
Meta’s AI Mind-Reading: Looking Ahead
Despite the challenges and ethical conundrums, Meta’s AI mind-reading project heralds a new wave of possibilities in how we interact with computers—and how computers understand us. The technology is still in its infancy, but the 80% accuracy figure is a milestone that can’t be ignored.
As we dream about a future filled with frictionless communication between our brains and machines, we also have to grapple with questions about who controls this data and how to ensure it’s used responsibly. If we handle this right, we might be on the cusp of an era that empowers people with disabilities, unravels the mysteries of cognition, and streamlines our everyday tasks.
And who knows? Maybe one day we’ll be browsing social media or firing off emails purely by thinking, “Send message.” Scary or thrilling? Maybe a bit of both.
So, the big question: Are we ready for an AI that can peer into our minds, or is this stepping into Black Mirror territory? Let us know in the comments below. And don’t forget to subscribe to our newsletter outlining the latest AI happenings, especially in Asia.
You may also like:
- Mind-Reading AI: Recreating Images from Brain Waves with Unprecedented Accuracy
- Apple and Meta Explore AI Partnership
- Merging Minds: The Future of AI by 2045
- Or try out Meta AI for free by tapping here.
Author
Discover more from AIinASIA
Subscribe to get the latest posts sent to your email.

The Secret to Using Generative AI Effectively In 2025

AI-pril Fools! How AI is Outsmarting Our Best Pranks

OpenAI’s New ChatGPT Image Policy: Is AI Moderation Becoming Too Lax?
Trending
-
News2 weeks ago
Adobe Jumps into AI Video: Exploring Firefly’s New Video Generator
-
Life1 day ago
AI-pril Fools! How AI is Outsmarting Our Best Pranks
-
News2 days ago
OpenAI’s New ChatGPT Image Policy: Is AI Moderation Becoming Too Lax?
-
News7 days ago
Google’s Gemini AI is Coming to Your Chrome Browser — Here’s the Inside Scoop
-
Business6 days ago
Forget the panic: AI Isn’t Here to Replace Us—It’s Here to Elevate Our Roles
-
Tools16 hours ago
The Secret to Using Generative AI Effectively In 2025
-
News5 days ago
Tencent Joins China’s AI Race with New T1 Reasoning Model Launch