News
Navigating the First AI Winter: Lessons from Asia’s Artificial Intelligence History
The first AI winter (1974-1980) was a challenging period for AI research, marked by reduced funding and interest. This article explores the causes, impact, and key figures of this era and its lessons for the future of AI and AGI in Asia.
Published
4 months agoon
By
AIinAsia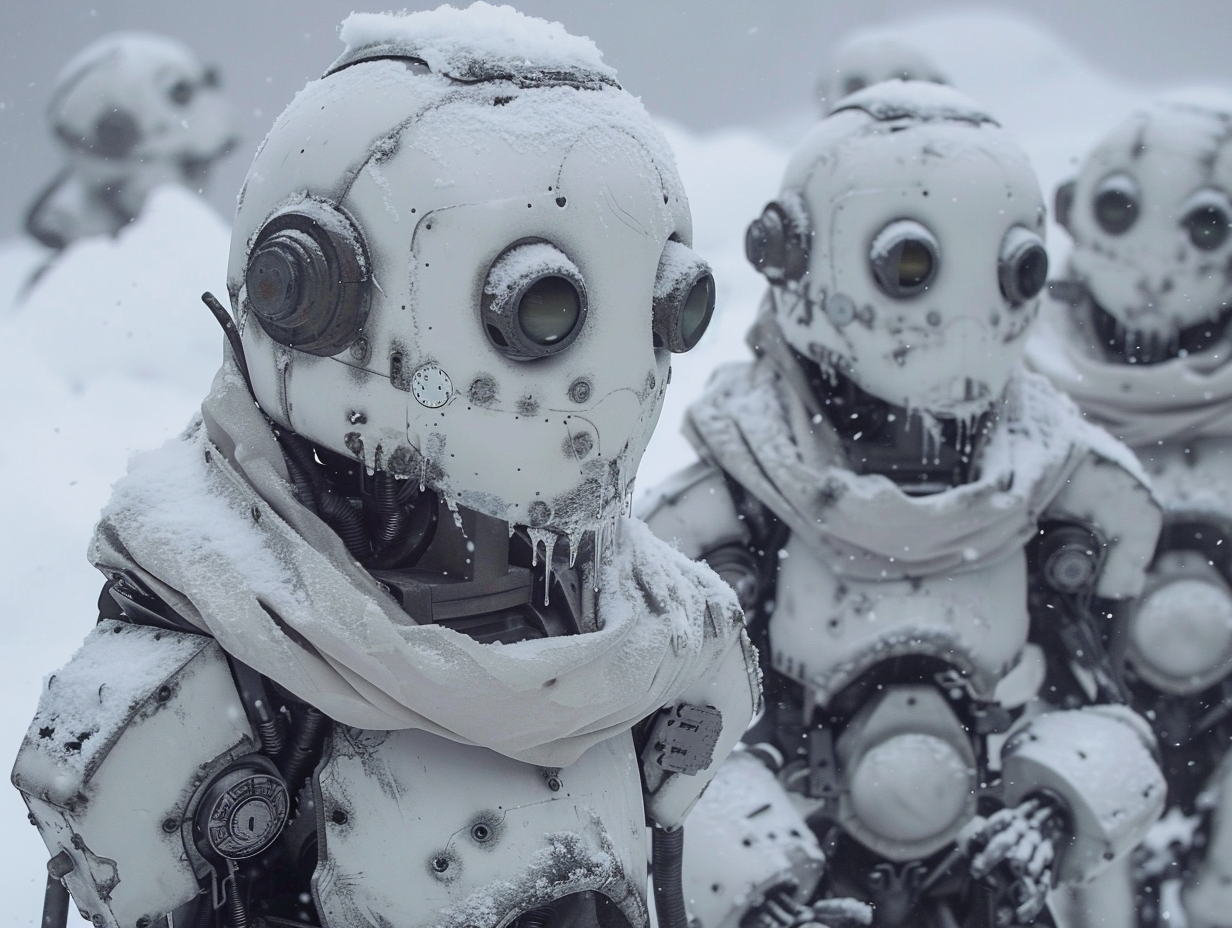
TL;DR:
- The first AI winter (1974-1980) was a period of reduced funding and interest in artificial intelligence research due to overhyped expectations, technical limitations, and critical reports.
- Key figures like Marvin Minsky, James Lighthill, and Herbert Simon played significant roles during this period, shaping the trajectory of AI research.
- The AI winter had profound effects on the field, but some researchers continued to make progress, leading to a more focused approach to AI research.
The First AI Winter: A Historical Overview
Artificial intelligence (AI) has come a long way since its inception, with numerous advancements shaping the technological landscape. However, the journey has not been without its challenges. The first AI winter, spanning from 1974 to 1980, marked a significant period of reduced funding and interest in AI research. This downturn followed an era of high expectations and optimism in the 1950s and 1960s, when researchers made bold predictions about AI’s potential. The winter was triggered by a combination of factors, including overhyped expectations, technical limitations, and critical reports like the Lighthill Report, which questioned the field’s progress and led to funding cuts.
Causes of the First AI Winter
The first AI winter was caused by several factors that led to reduced funding and interest in artificial intelligence research:
- Overhyped expectations: Early AI researchers made ambitious predictions about AI capabilities that failed to materialize, leading to disappointment.
- Technical limitations: The computing power and algorithms available at the time were insufficient to solve complex real-world problems, exposing the limitations of early AI systems.
- Lighthill Report: This influential 1973 report criticized AI research for failing to achieve its “grandiose objectives,” leading to funding cuts in the UK.
- Combinatorial explosion: Researchers realized that many AI problems faced exponential growth in complexity as input size increased, making them computationally intractable.
- Lack of computing power: The hardware available at the time was insufficient to handle the computational requirements of many AI applications.
- Funding cuts: Government agencies, particularly DARPA in the US, reduced or eliminated funding for AI research.
These factors collectively contributed to a loss of confidence in AI’s potential, leading to the first AI winter.
Impact of the First AI Winter
The first AI winter had profound effects on the field, leading to a significant reduction in funding from government agencies and private investors. Many AI projects were shut down, and research activities slowed considerably. Researchers shifted their focus to other areas of computer science perceived to have more immediate practical applications. Despite these setbacks, some researchers continued to make progress, developing new ideas in areas such as logic programming and commonsense reasoning. The period also led to a more measured and focused approach to AI research, setting the stage for future advancements in the field.
Key Figures and Their Contributions
Several key figures played important roles during and around the first AI winter period:
- Marvin Minsky: A co-founder of MIT’s AI laboratory, Minsky contributed to early AI research but also inadvertently contributed to the winter. His 1969 book “Perceptrons” highlighted limitations of single-layer neural networks, leading to reduced interest in neural network research for over a decade.
- James Lighthill: A prominent mathematician who authored the influential Lighthill Report in 1973. His scathing critique of AI research’s progress significantly impacted funding and public perception of AI in the UK.
- Herbert Simon: An early AI pioneer who made overly optimistic predictions about AI’s capabilities, contributing to inflated expectations.
AI Research Revival
The revival of AI after the first winter was marked by several significant projects and advancements. Expert systems, machine learning, and neural networks saw renewed interest, with researchers exploring new approaches to overcome previous limitations. Additionally, the increased availability of computing power and growth in data enabled AI systems to tackle more complex problems. These projects and advancements collectively contributed to a resurgence of interest and progress in AI, effectively ending the first AI winter and setting the stage for further developments in the field.
Comment and Share
What lessons can we learn from the first AI winter, and how can we apply them to the current state of AI and AGI in Asia? Share your thoughts in the comments below and subscribe for updates on AI and AGI developments.
You may also like:
- AI Increases Google’s Carbon Footprint by Nearly 50%
- The Rise of Mistral AI: Europe’s Contender in the Global AI Race
- The Demise of the Web: How Google and AI are Redefining the Internet
- To learn more about the first AI winter tap here.
Author
Discover more from AIinASIA
Subscribe to get the latest posts sent to your email.
You may like
Life
AI at the Polls: Is Technology Steering the 2024 US Election?
As Americans cast their votes tomorrow, artificial intelligence will play a quiet but powerful role behind the scenes.
Published
3 days agoon
November 4, 2024By
AIinAsia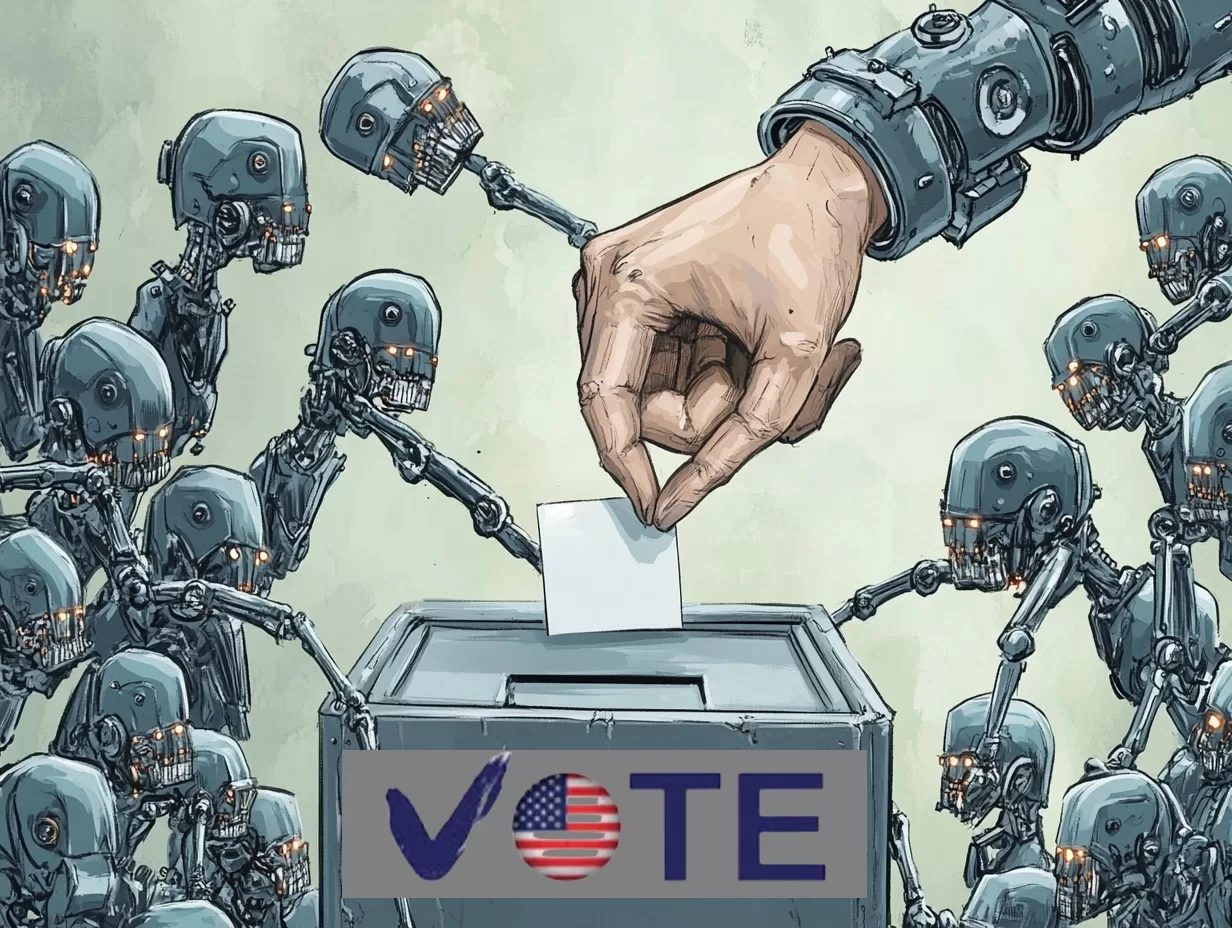
TL;DR:
- Campaign ads, social media feeds, and even “news” popping up in swing states are being shaped by AI’s invisible hand
- Campaigns in 2024 aren’t just reaching voters; they’re diving deep into our digital footprints
- AI brings campaigns closer to voters, it also makes it easier than ever to spread misinformation
A New Political Battleground—Inside the AI-Powered Election
As Americans cast their votes tomorrow, artificial intelligence will play a quiet but powerful role behind the scenes. Campaign ads, social media feeds, and even “news” popping up in swing states are being shaped by AI’s invisible hand. This isn’t just the next step in election tech; it’s a dramatic leap that could change the game forever. Is AI enhancing democracy, or are we giving it the keys to the whole democratic car?
1. Supercharging Campaigns: Microtargeting to the Extreme
Let’s face it—if you feel like your social media feeds are eerily personal, that’s not a coincidence. Campaigns in 2024 aren’t just reaching voters; they’re diving deep into our digital footprints to send messages so tailored they feel like personal letters. Thanks to AI, campaigns can slice the electorate into precise segments, tapping into anxieties, interests, and even specific local issues.
In battleground states like Arizona and Pennsylvania, this tech-driven targeting reaches a fever pitch. AI sifts through oceans of data—social media interactions, browsing habits, even purchase history—to craft ads that connect directly with you, personally.
“Campaigns are increasingly leveraging sophisticated machine learning algorithms to analyse vast quantities of voter data, refining their strategies with pinpoint accuracy,” notes MIT Technology Review (source).
With AI knowing so much, it raises an interesting (if slightly chilling) question: where’s the line between effective campaigning and outright manipulation?
2. The Double-Edged Sword: AI, Deepfakes, and Digital Misinformation
Here’s the darker side. While AI brings campaigns closer to voters, it also makes it easier than ever to spread misinformation. AI-generated deepfakes—fake videos that look so real you wouldn’t know they’re fake—have added a surreal twist to this election. Imagine seeing a video of a candidate saying something outrageous… and then realising it never actually happened.
“Deepfakes have made the spread of disinformation much easier and more convincing, raising concerns about the future of truth in politics,” the Brookings Institution warns (source).
AI’s power to create convincing fakes isn’t just a technical marvel; it’s a fundamental threat to truth in politics. Without strict regulations or ways to fact-check in real-time, we’re left wondering how many people will cast their vote based on a lie.
3. Predictive Polling: AI, Sentiment Analysis, and the All-Seeing Eye
If you thought AI was only influencing what you see online, think again. Polling has evolved far beyond traditional methods. This election, campaigns are using AI-driven sentiment analysis to tap into public moods in real time, keeping a pulse on issues that resonate with voters minute by minute.
“Sentiment analysis enables campaigns to see beyond traditional polling, observing shifts in public mood and identifying emerging concerns as they happen,” reports the Pew Research Center (source).
Let’s say economic concerns are heating up in Georgia; Trump’s team could amplify ads focusing on job growth in just hours. Or Harris’s camp could hone in on climate change in Michigan based on AI-driven insights from yesterday’s online conversations. This real-time fine-tuning isn’t just impressive—it’s a little mind-bending. Can polls really capture the pulse of the nation, or are we just seeing what AI’s algorithms want us to?
4. Mobilising the Masses: AI Nudges and Digital Persuasion
Getting people to the polls has always been crucial, and AI’s here to make sure more people than ever get nudged, reminded, and maybe even guilt-tripped into voting. AI-driven models predict not only who’s likely to vote but also who might need a little extra encouragement. Campaigns can then send targeted texts, emails, or even pop up on your social feed reminding you to “make your voice heard.”
The Atlantic remarks on AI’s power in mobilisation, stating, “AI has transformed voter outreach into an exact science, enabling campaigns to efficiently target and mobilise segments of the electorate that might otherwise stay home” (source).
For instance, Harris’s campaign has deployed AI to boost turnout among younger voters in key states, while Trump’s team uses it to rally dedicated supporters in traditionally red zones. AI doesn’t just follow you online; it’s practically waiting outside your door with a “Don’t forget to vote” sign. This kind of outreach raises a fascinating question about voter autonomy—are we freely deciding to vote, or are we being nudged by an algorithm?
5. Navigating the Ethical Minefield: Can Democracy Keep Up?
Here’s where it all gets tricky. While AI offers stunning capabilities for reaching, engaging, and mobilising voters, it also opens up new doors for potential misuse. From deepfakes to ultra-targeted political ads, AI is testing the limits of what’s fair game in political campaigns.
With regulations still trying to catch up, we’re left with a significant blind spot.
“Current frameworks for AI regulation are woefully inadequate, leaving a critical gap in safeguarding electoral processes,” states the Harvard Political Review (source).
AI has handed campaigns a powerful toolkit, but with great power comes… well, you know the rest. Without real oversight, there’s a real risk of crossing ethical lines, leaving voters questioning whether their choices are truly their own or just the echoes of an algorithm.
A Glimpse into Asia’s Future?
As AI’s influence in US elections becomes clear, Asia’s political landscape might not be far behind. In a region where social media is booming and governments increasingly leverage AI for everything from citizen services to surveillance, the potential for AI-driven election strategies is immense. Imagine a world where voter preferences in Tokyo, Jakarta, or Delhi are meticulously profiled, and campaign ads are hyper-personalised to every demographic, language, and cultural nuance. But here’s the question for Asia: with AI’s rapid adoption and limited oversight, who will control this powerful tool—governments, political parties, or the people? The US election offers a glimpse of how AI can shape democracy, but will Asia be able to harness this power responsibly, or could it open doors to unprecedented political manipulation? The stakes are high, and the path ahead remains uncharted.
Join the Conversation
How do you think AI will impact elections in Asia? Will it drive democracy forward or lead to new challenges in political manipulation? Leave a comment or subscribe for AI in Asia updates.
You may also like:
- AI Voice Cloning: A Looming Threat to Democracy
- AI Chatbots Struggle with Real-Time Political News: Are They Ready to Monitor Elections?
- AI-Fakes Detection Is Failing Voters in the Global South
- To learn more about the role of AI in the US elections, tap here.
Author
Discover more from AIinASIA
Subscribe to get the latest posts sent to your email.
News
Japan’s Nuclear Revival: Powering AI and a Greener Future
Japan is reviving nuclear power to fuel AI technologies and meet climate goals, with a focus on safety and public concern.
Published
2 weeks agoon
October 23, 2024By
AIinAsia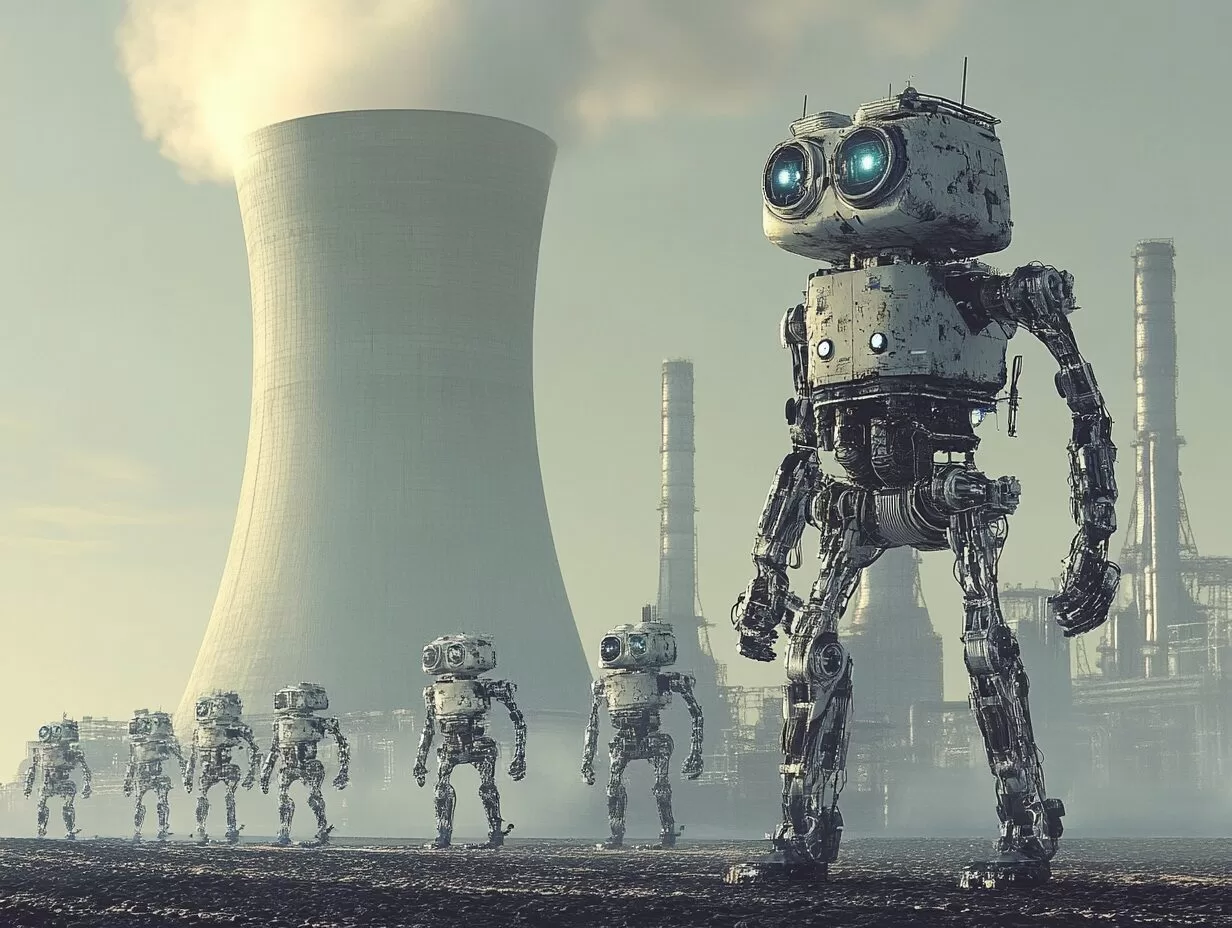
TL;DR:
- Japan is restarting nuclear power plants to reduce coal dependency and meet climate goals.
- The Kashiwazaki-Kariwa plant has new safety measures, including a 15-meter tsunami wall.
- Nuclear power is essential for Japan’s plans to expand AI data centers.
- Japan aims to increase nuclear power to 20-22% of its electricity mix by 2030.
The Nuclear Comeback
Japan is making a significant shift back to nuclear power. After the 2011 Fukushima disaster, the country shut down all its nuclear reactors. However, with climate change concerns and the need to power energy-hungry AI data centers, Japan is now reconsidering its stance on nuclear energy.
Safety First
At the heart of this revival is the Kashiwazaki-Kariwa nuclear plant in Niigata Prefecture. The plant has undergone extensive upgrades to prevent another Fukushima-like disaster. These include a 15-meter tsunami wall, backup power supply vehicles, and a new vent to filter out radioactive particles.
Masaki Daito, the plant’s deputy superintendent, believes these measures can prevent a similar accident. He told AFP, “We believe that a similar accident to Fukushima could be largely avoided. Japan now has the strictest (regulatory) standards in the world.”
The Energy Dilemma
Before the Fukushima disaster, nuclear power generated about a third of Japan’s electricity. Post-Fukushima, Japan increased imports of natural gas, coal, and oil to keep the lights on. However, this has not only been expensive but has also hindered Japan’s decarbonization efforts.
The E3G think-tank ranks Japan last among G7 nations in decarbonizing their power systems. Hanna Hakko, an energy expert at E3G, believes Japan could aim for renewables to generate 70-80% of its power by 2035. However, even in this scenario, the remainder would need to be covered by gas and nuclear energy.
The AI Factor
Japan’s ambitions in AI are another driving force behind the nuclear revival. AI data centers require vast amounts of energy, and nuclear power could provide a stable, low-emission source.
Prime Minister Shigeru Ishiba acknowledged this, stating, “Obviously, nuclear energy needs to be utilized.”
The Public’s Concern
Despite these plans, the memory of Fukushima still looms large. Many Japanese, like Mototsugu Oki, remain skeptical about nuclear power. “It is operated by human beings, and human beings naturally make mistakes,” he told AFP.
Moreover, making Fukushima fully safe is a monumental task. Engineers are still grappling with how to handle highly radioactive fuel and rubble.
The Path Ahead
Japan aims to increase nuclear power to 20-22% of its electricity mix by 2030. Nine reactors are currently online, with more set to join once they receive local approval.
However, the process is slow due to tougher safety rules. One restart was recently blocked because of earthquake risk.
Comment and Share:
What are your thoughts on Japan’s nuclear revival? Would you support nuclear power in your country to reduce carbon emissions and power AI technologies? Subscribe for updates on AI and AGI developments and share your thoughts in the comments below!
- You may also like:
- AI Fusion Powered Energy of the Future: A Chat with Sam Altman
- AI Powering Data Centres and Draining Energy
- 14 Ways Smart Grids Will Transform Asia’s Energy Landscape by 2030
- To learn more about Japan’s nuclear power influenced by AI, tap here.
Author
Discover more from AIinASIA
Subscribe to get the latest posts sent to your email.
News
Revolution Ahead: Microsoft’s AI Agents Set to Transform Asian Workplaces
Microsoft’s AI agents are set to transform Asian workplaces, boosting productivity and showcasing the practical benefits of AI.
Published
2 weeks agoon
October 23, 2024By
AIinAsia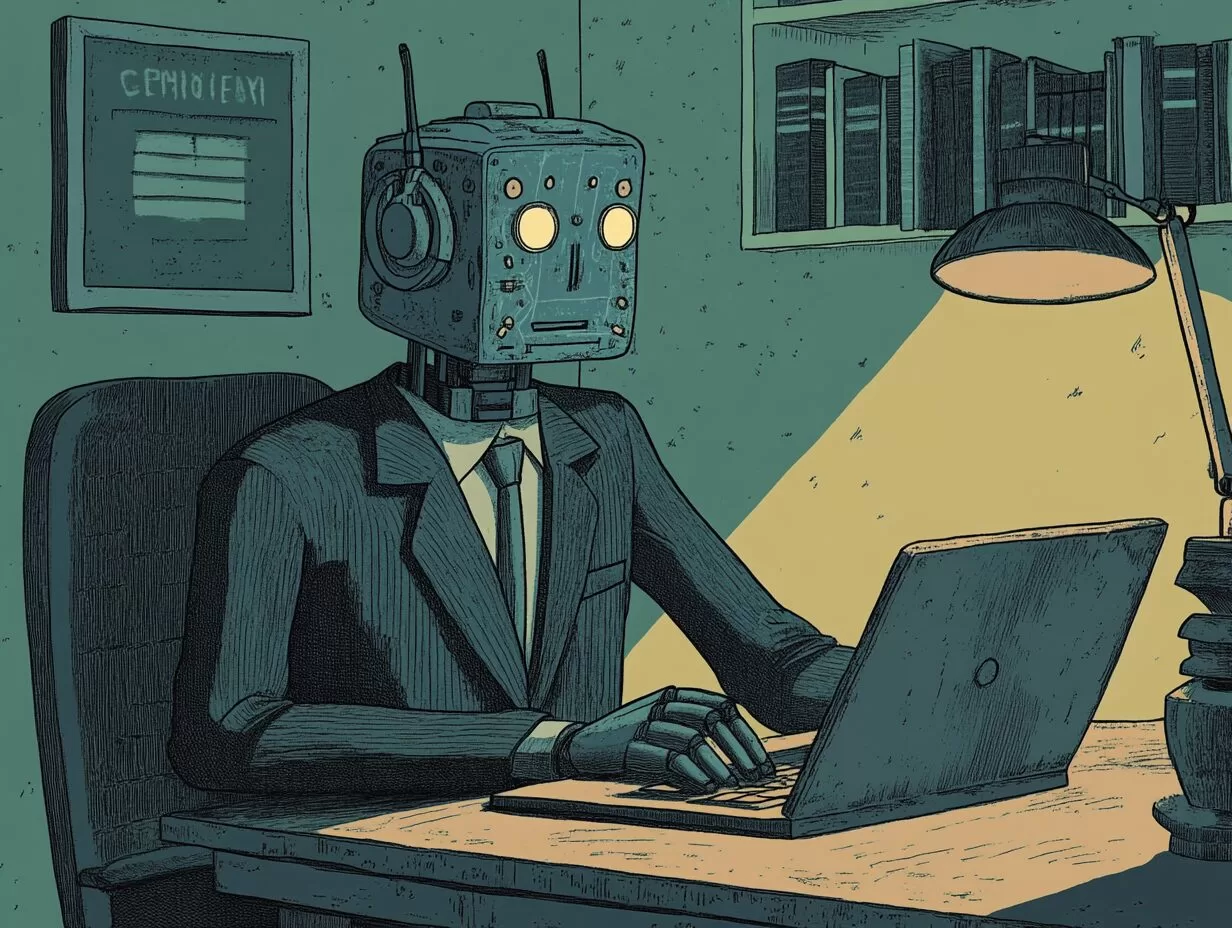
- Microsoft is launching AI agents to handle tasks like client queries and supply chain management.
- Copilot Studio will allow businesses to create AI agents without coding skills.
- Early adopters like McKinsey and Clifford Chance are already using these agents.
- AI agents could boost productivity and justify investments in AI technology.
Imagine a world where artificial intelligence handles your most tedious tasks at work. That world is just around the corner, as Microsoft prepares to launch autonomous AI agents next month. These virtual employees are set to revolutionise businesses, especially in Asia’s tech-savvy landscape. Let’s dive into what these AI agents can do, who’s already using them, and what it means for the future of work.
AI Agents: The Next Big Thing in Business Operations
Microsoft’s AI agents are designed to take on routine tasks, freeing up human employees for more creative and strategic work. Here’s what they can do:
- Handle Client Queries: AI agents can manage customer service tasks, ensuring clients get quick and accurate responses.
- Identify Sales Leads: These virtual employees can help sales teams by identifying and qualifying leads.
- Manage Supply Chains: AI agents can streamline supply chain management, making it more efficient and reducing errors.
Copilot Studio: No Coding Skills Required
Microsoft’s Copilot Studio is a game-changer. It allows companies to create their own AI agents without needing any coding skills. Here’s how it works:
- Pre-configured Agents: Microsoft will release 10 pre-configured AI agents designed for specific functions, like customer service and supply chain tasks.
- Powered by Top AI Models: These agents will be powered by AI models developed by Microsoft and OpenAI, the creators of ChatGPT.
“Copilot Studio aims to simplify the creation of AI agents, requiring no technical knowledge from users.”
Early Adopters Leading the Way
Several companies are already using Microsoft’s AI agents to streamline their operations. Here are a few examples:
- McKinsey: This consulting firm is using AI agents to schedule meetings and manage customer inquiries.
- Clifford Chance: This law firm is leveraging AI agents to improve efficiency and reduce wasted time.
- Pets at Home: This retailer is using AI agents to enhance workplace productivity.
Satya Nadella, Microsoft’s CEO, said, “These tools could revolutionize outsourcing by improving efficiency and reducing wasted time.”
The Future of AI Agents
Microsoft isn’t stopping at AI agents for basic tasks. They’re working on an agent capable of performing transactions on behalf of users. While there have been challenges, the technology is progressing rapidly.
Mustafa Suleyman, Microsoft’s head of AI, revealed that this transaction-capable agent could be available in a few months.
Addressing Concerns About Job Losses
There are concerns about the potential impact of AI agents on employment. However, Microsoft believes these agents will empower employees by taking over monotonous tasks.
Charles Lamanna, a corporate vice-president at Microsoft, said, “AI agents would enable employees to focus on more creative and meaningful work.”
The Potential Return on AI Investment
AI agents could provide a return on the significant investments tech companies have made in AI. While it’s uncertain how much revenue they will generate, they could showcase the practical benefits of AI.
Andrew Rogoyski, director at the Institute for People-Centred AI at the University of Surrey, noted that AI agents could help justify the billions of dollars poured into AI research and development.
What’s Next for AI in Asia?
Asia, with its tech-savvy population and rapid digital transformation, is poised to benefit greatly from Microsoft’s AI agents. Companies in the region could see increased productivity, improved customer service, and more efficient supply chains.
Comment and Share:
What tasks do you think AI agents could help with in your workplace? Share your thoughts below and don’t forget to subscribe for updates on AI and AGI developments. We’d love to hear about your experiences with AI technologies and your predictions for future trends!
- You may also like:
- How Digital Agents Will Transform the Future of Work
- Unleashing the Power of AI Agents
- Worker Exploitation Rife in AI Industry
- To learn more about Microsoft’s plans to launch AI employees, tap here.
Author
Discover more from AIinASIA
Subscribe to get the latest posts sent to your email.

Can AI Make Uber + Expedia the Next Big Super App

Adrian’s Arena: AI and the Global Shift – What Trump’s 2024 Victory Means for AI in Asia

Google’s AI Overviews Go Global
Trending
-
Learning3 days ago
AI Unleashed: Discover the Power of Notion AI
-
Learning2 weeks ago
AI Unleashed: Discover the Power of Midjourney AI
-
Learning1 week ago
AI Unleashed: Discover the Power of Gemini AI
-
Learning1 week ago
AI Unleashed: Discover the Power of Claude AI
-
Business3 weeks ago
Adrian’s Arena: East Meets West – Contrasting AI Partnership Strategies for OpenAI
-
Learning2 weeks ago
AI Unleashed: Discover the Power of ChatGPT
-
Marketing2 weeks ago
Adrian’s Arena: AI is Shaping the Future of Marketing to Gen Z in Southeast Asia
-
Marketing1 week ago
Adrian’s Arena: The AI-Driven Playbook for Winning Over APAC Consumers