Business
Go Deeper – Green AI: Navigating Asia’s Journey Towards Sustainable Artificial Intelligence
A comprehensive look at both the advancements and the challenges in integrating AI with environmental goals in the region.
Published
1 year agoon
By
AIinAsia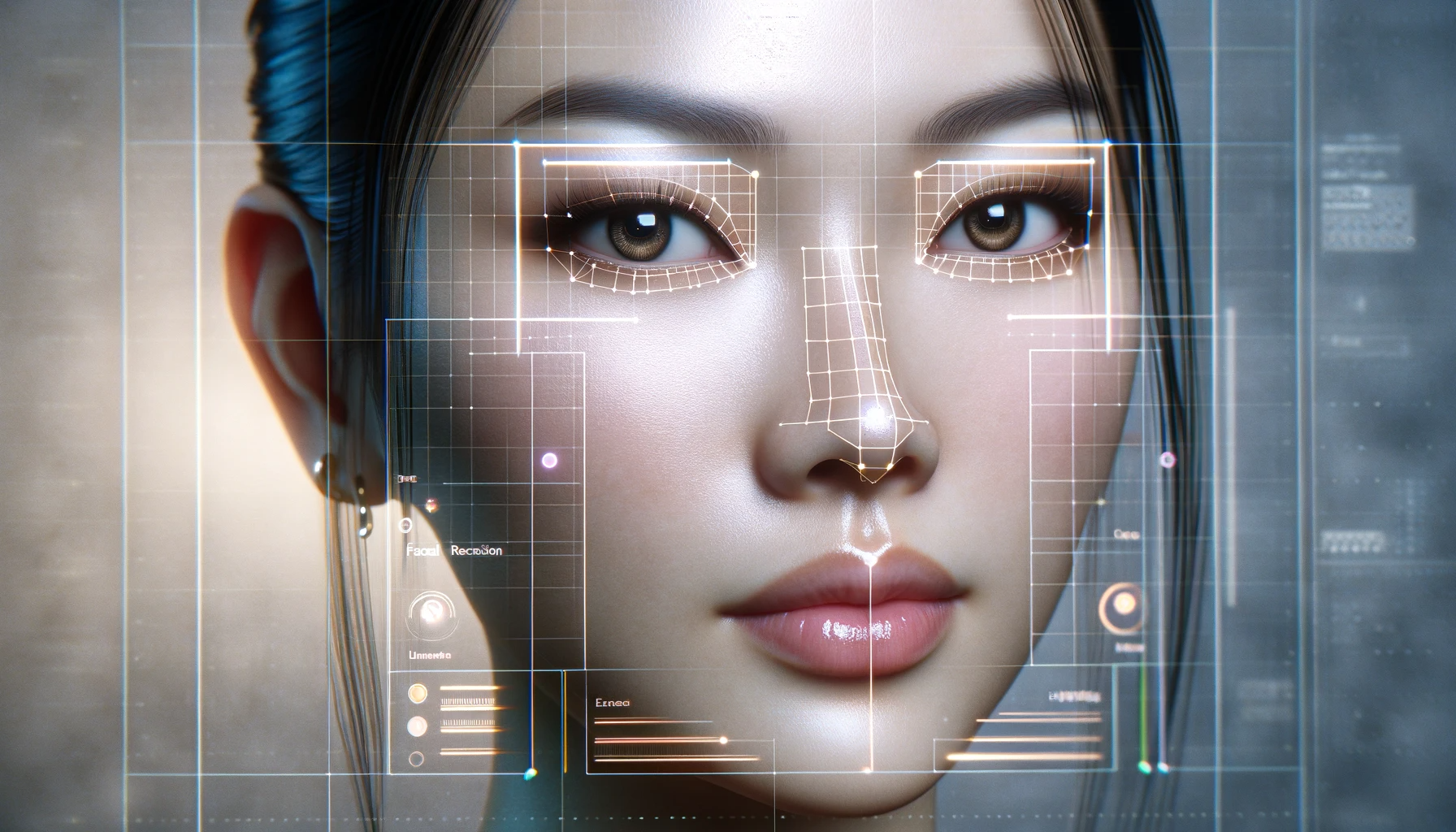
TL/DR:
- AI’s rapid growth in Asia brings environmental concerns due to its high energy consumption.
- Green AI in Asia innovative solutions like energy-efficient hardware and renewable energy sources are being developed in the region.
- Governments and communities play a crucial role in ensuring a sustainable, equitable, and ethical AI future.
Introduction
Artificial Intelligence (AI) is revolutionising industries across Asia, from smart cities to agriculture. However, its environmental footprint raises concerns about the region’s green aspirations. This article delves into the unique challenges and potential of AI’s environmental impact in Asia, while exploring innovative solutions and the role of governments and communities in shaping a sustainable AI future.
Asian Footprints, Western Precedents: The Data Revealed
The scale of AI’s energy consumption is staggering. Training a single large language model like Google’s PaLM, with its 540 billion parameters, can emit over 626,000 pounds of CO2, equaling five cars’ lifetime emissions.
Inference, the process of using these models for predictions, adds another layer, with daily estimates reaching 50 pounds of CO2 for an LLM, or a hefty 8.4 tons per year.
In Asia, Baidu’s Ernie-3.0 Titan language model, boasting 176 billion parameters, is no slouch in this energy race, highlighting the need for regional considerations (data is courtesy of arxiv.org)
Asian AI and the Carbon Conundrum

Asia’s rapid AI adoption intensifies the carbon issue. China, accounting for 27% of global AI investments, and India, with its projected $8 billion AI market by 2025, illustrate the region’s rapid embrace of this technology (statista.com, analyticsindiamag.com). From facial recognition systems in bustling metropolises to autonomous vehicles navigating crowded streets, these applications demand close examination of their energy footprint within the context of each nation’s energy mix and emission goals.
Beyond the Cloud: Asian Initiatives for Greener AI
Asia is not only facing the problem but also leading in finding solutions. Innovators across the region are developing cutting-edge technologies to reduce AI’s environmental impact.
- Energy-Efficient Hardware: India’s Centre for Development of Advanced Computing (CDAC) is pioneering energy-efficient hardware solutions tailored for AI workloads. These innovations aim to decouple AI advancements from unsustainable energy practices (cdac.in).
- Green Data Centres: China’s Alibaba Cloud boasts its “Sustainable Computing Initiative,” utilising renewable energy sources and cutting-edge chip technologies to green its data centres (alibabacloud.com).
- Cooling Algorithms: Japan’s NEC Laboratories developed a machine learning algorithm that reduces data centre cooling energy consumption by up to 50%, a crucial innovation considering data centres in China alone consume 2.7% of the nation’s total energy (nec.com, China Academy of Information and Communications Technology).
Case Studies: Balancing Benefits and Challenges
1. Smart Agriculture: Balancing Efficiency with Energy Demand

Across Asia, the rise of smart agriculture promises both environmental benefits and challenges. AI-powered drones in Thailand, equipped with imaging technology, helped farmers reduce chemical pesticide use by 30% (World Resources Institute), a win for sustainability. However, these technologies necessitate energy for charging, data transmission, and cloud computing, potentially negating their ecological advantages. Finding ways to optimise energy consumption through AI itself, like NEC’s cooling innovation, is crucial for ensuring smart agriculture truly delivers on its green promise.
2. Facial Recognition: Security vs. Transparency and Sustainability

In China, vast networks of facial recognition cameras enhance public safety while raising concerns about energy consumption and data privacy. A single camera can consume up to 1,500 kWh per year, equivalent to a typical household fridge (South China Morning Post). Implementing facial recognition systems that leverage energy-efficient hardware and prioritise responsible data management, alongside exploring alternative security solutions, is crucial for mitigating the footprint and ensuring public trust.
3. Renewable Energy Integration: Powering AI with Clean Sources

The growing appetite of AI for energy necessitates a shift towards renewable resources. India’s National AI Strategy aims to power data centers with solar and wind energy, potentially reducing their carbon footprint by up to 80% (NITI Aayog). This not only reduces AI’s own emissions but also contributes to national clean energy goals. Japan’s NEC Laboratories have developed AI algorithms that optimise data center cooling, saving up to 50% in energy consumption (NEC). Such innovations pave the way for a more sustainable and efficient future for AI infrastructure.
Policy Catalysts: Steering AI Towards Sustainability
Governments across Asia are implementing initiatives to promote energy-efficient AI and address the environmental concerns associated with AI growth.
- Green Data Centres: Singapore’s Green Data Centre initiative incentivises energy-efficient data centre operations, promoting the adoption of best practices in design, build, and operation of data centres to reduce energy consumption and environmental impact.
- Ethical AI Guidelines: South Korea’s Ministry of Science and ICT has established ethical AI guidelines, emphasising the importance of transparency, accountability, and fairness in AI development and deployment, which can indirectly contribute to more sustainable AI practices.
Eco-friendly AI: Where Will the Green Path Lead For AI in Asia

Imagine a future where AI isn’t just a power-hungry consumer, but an environmental guardian. Imagine AI-powered drones planting trees at a rate exceeding deforestation, their movements optimised by algorithms trained on satellite imagery. Envision city-wide energy grids, seamlessly integrating renewable sources with the help of AI algorithms predicting demand and fluctuations (World Economic Forum). These scenarios, once science fiction, become increasingly plausible with rapid advancements in green AI research.
The Role of Green AI in Asian Startups and Innovation Hubs
Asia’s thriving startup ecosystem is playing a significant role in driving sustainable AI innovation. Entrepreneurs are developing creative solutions to address AI’s environmental impact, from AI-powered energy management systems to algorithms that optimise resource allocation. For example, Hong Kong-based startup, Green Earth Energy, uses AI to optimise solar panel performance, maximising clean energy generation.
Doing The Right Thing: Navigating Bias and Data Justice
The promise of a greener future through AI cannot be separated from ensuring ethical development and deployment. Biases embedded in training data can perpetuate environmental injustices, favoring urban centers with resource-intensive AI applications while neglecting rural communities grappling with climate change impacts. Studies show facial recognition algorithms struggle with darker skin tones, raising concerns about discriminatory surveillance practices in vulnerable communities (MIT Technology Review). Addressing these ethical issues through diverse data sets, transparent algorithms, and community inclusion is crucial for a truly green and equitable AI future.
The cost of greening AI technologies can be substantial, yet the long-term economic benefits, such as energy savings and increased efficiency, can offset initial investments. A study by the Asian Development Bank (ADB) highlights that sustainable AI practices could boost Asia’s economy by enhancing productivity while preserving environmental integrity.
Ethical dimension of AI deployment, encompassing issues like data privacy, equitable access, and social impact, is gaining prominence. Initiatives like India’s AI ethics guidelines underscore the need for a balanced approach that considers both human and environmental welfare.
Embracing Cross-Cultural Perspectives to Achieve an AI Environmental Impact
Asia’s diverse landscape necessitates a nuanced approach to green AI. China’s centralised governance model contrasts with India’s decentralised ecosystem, requiring tailored policy frameworks and solutions. Culturally specific concerns, such as data privacy in Japan and resource extraction in Indonesia, need to be addressed within local contexts. Sharing best practices across borders and fostering regional collaboration can bridge these gaps and accelerate progress towards shared environmental goals.
Empowering Communities to Takes Center Stage for a Green AI in Asia

Green AI isn’t merely a top-down technological solution; it demands active participation from the communities it impacts. Open-source AI platforms like TensorFlow and PyTorch empower local communities to develop their own solutions and monitor environmental impacts using sensor networks and citizen science initiatives. Imagine farmers in rural Thailand utilising AI-powered soil analysis tools developed by their peers, optimising water usage and crop yields while minimising environmental footprint (FAO). Such grassroots innovations hold immense potential for a sustainable and inclusive AI future.
Data-Driven Insights, Visual Clarity:
To effectively communicate the complexities of AI’s environmental impact and potential, compelling data and visuals are critical. Charts illustrating the projected reduction in carbon footprint from China’s AI policy (NITI Aayog) or images showcasing AI-powered robots cleaning plastic from polluted rivers can make the abstract tangible and impactful. Engaging infographics and data visualisations can further enhance the article’s accessibility and inspire action.
By exploring these additional dimensions, we gain a holistic understanding of the challenges and opportunities shaping AI’s environmental future in Asia.
It’s clear this journey requires not just technological advancements, but also ethical considerations, cross-cultural collaboration, and the active participation of empowered communities.
Only then can we ensure that the path towards a greener future with AI is truly inclusive, sustainable, and bright.
Comment and Share on Green AI in Asia
What role do you think individuals and communities play in promoting sustainable AI practices in Asia? Share your thoughts below and subscribe for updates on AI and AGI developments. Let’s build a greener, more inclusive AI future together.
You may also like:
- Green AI: Sustainable Solutions for Asia’s AI Boom
- Hungry for Power: AI and Crypto Drive Data Center Overload
- Sam Altman: It’ll be powered by Fusion, But Not Quite Skynet
- Europe Powers Up Generative AI Aspirations with Supercomputer Boost for Startups, But Can It Do So Sustainably?
Author
Discover more from AIinASIA
Subscribe to get the latest posts sent to your email.
You may like
-
Revolutionise Your Life: 8 Ways AI Can Boost Your Work-Life Balance
-
The View From Koo: Does Your Business Really Need an AI Strategist? The Surprising Answer
-
6 AI-Powered Paid Search Strategies You Can’t Ignore
-
AI Risk Management: Navigating the Opportunities and Challenges in Asia
-
5 Prompts to Ignite Your Job Search With AI
-
GO DEEPER: AI and the Future of Human Intelligence
Business
Adrian’s Arena: What Is Project Stargate and How Will It Impact Asia’s AI Future?
How Project Stargate’s $500 billion investment may impact Asia’s AI future—opportunities, challenges, and strategies for Asia.
Published
2 hours agoon
January 22, 2025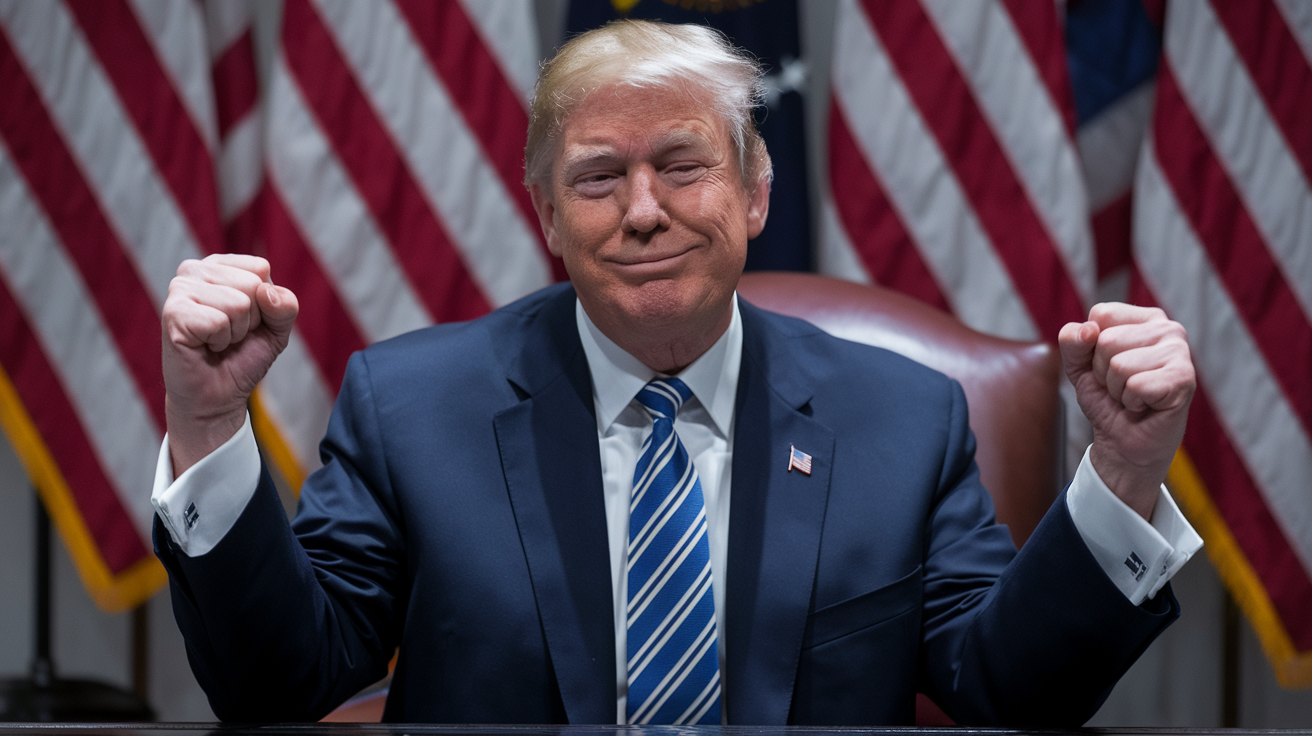
TL;DR
- The $500 billion Project Stargate initiative by the US is set to reshape global AI dynamics, pushing Asia to innovate rapidly.
- Asia faces challenges like brain drain, regulatory pressures, and the need for faster AI adoption across industries.
- Opportunities abound in collaboration, regional specialisation, and industry-specific transformations.
The Stargate Initiative: A Bold Move That’s Got Asia Watching
President Donald Trump has just announced Project Stargate, a groundbreaking AI initiative designed to position the United States as the global AI leader. With an investment of up to $500 billion and the backing of OpenAI, Oracle, and SoftBank, Stargate is set to redefine AI infrastructure and talent development.
But here’s the thing: while this project is US-focused, its ripple effects will be felt far beyond its borders. For Asia, the stakes are high. Can the region capitalise on this moment, or will it find itself playing catch-up?
Let’s explore how Project Stargate is reshaping the game for Asia’s AI ambitions, industry transformation, and policy development.
What is Project Stargate?
Announced on 22 January 2025, Project Stargate is the United States’ most ambitious AI initiative to date, aiming to secure global AI dominance through a $500 billion investment. The programme is a collaborative effort between the US government and key industry players, including OpenAI, Oracle, and SoftBank. Its scope spans three primary pillars:
- AI Infrastructure Development:
- Establishing a nationwide network of AI supercomputers to support cutting-edge research and deployment.
- Building AI-ready data centres optimised for scalability and efficiency.
- Upgrading digital infrastructure in rural and underserved areas to democratise AI access.
- Talent and Workforce Upskilling:
- Creating over 100,000 high-paying AI-related jobs in research, development, and deployment.
- Introducing AI education into primary and secondary schools to nurture talent from an early age.
- Partnering with universities and private institutions to fund scholarships, fellowships, and training programmes.
- Industry-Focused Initiatives:
- Prioritising sectors like healthcare, defence, energy, and finance for AI integration.
- Providing grants and incentives for startups innovating in AI applications, from generative AI tools to advanced robotics.
- Accelerating the development of AI ethics frameworks and ensuring AI systems align with democratic values.
Timeline and Milestones: Project Stargate is designed as a phased initiative with key milestones over the next decade:
- 2025-2027: Laying the Foundation
- Deploy AI-ready infrastructure in priority regions across the US.
- Begin workforce upskilling programmes, with an initial focus on underserved communities.
- 2028-2030: Scaling Innovation
- Launch major AI initiatives in healthcare (e.g., diagnostics powered by large language models) and defence (e.g., predictive analytics for national security).
- Begin exporting advanced AI technologies to allies, fostering collaboration while ensuring US leadership.
- 2031 and Beyond: Global AI Leadership
- Establish the US as the go-to destination for AI talent, innovation, and investment.
- Shape global AI standards and ethics policies through multilateral partnerships.
Technologies at the Core of Project Stargate: The initiative will focus on driving innovation in several cutting-edge areas:
- Generative AI: Developing models with improved creativity, contextual understanding, and multi-modal capabilities.
- Edge AI: Enhancing AI’s performance in IoT devices, enabling real-time decision-making at the source.
- Quantum AI: Investing in quantum computing to push the boundaries of problem-solving and data processing.
- AI Safety: Creating robust, interpretable AI systems that align with ethical standards.
Asia’s AI Competitiveness: Time to Double Down
Stargate has put Asia’s AI strategies under the spotlight. The US’s massive investment is a wake-up call for governments and businesses alike to accelerate their AI initiatives. Here’s how some of Asia’s key players are reacting:
- China: Already a global AI powerhouse, wlth an AI market projected to reach $145 billion by 2030, China’s national AI strategy is likely to ramp up even further. From semiconductor manufacturing to AI-driven tech exports, Beijing will focus on countering Stargate’s competitive edge.
- India: Known for its IT expertise, India could use this moment to enhance its AI ecosystem. Expect initiatives like Digital India and Startup India to evolve with more focus on AI funding and local talent retention.
- Singapore: A regional AI leader, Singapore’s Smart Nation initiatives and plans to grow its AI workforce to 15,000 by 2028 may now need to scale up to stay ahead.
- ASEAN: As a bloc, ASEAN’s Digital Economy Framework Agreement (DEFA) could be pivotal in harmonising AI policies across the region to compete collectively.
Talent Tug-of-War: Will Asia Keep Its Best Minds?
One of the biggest challenges for Asia will be retaining top talent. With Stargate promising over 100,000 new AI jobs in the US, the lure of lucrative salaries and cutting-edge research opportunities will be hard to resist.
How Asia Can Fight Back
- Upskilling at scale: Programmes like AI Singapore and India’s AI-focused education initiatives are critical for building and retaining talent pipelines.
- Competitive incentives: Companies across Asia, from Alibaba to Tata Consultancy Services, are stepping up with attractive packages to retain talent.
- Reverse brain drain: As Asia’s AI ecosystems mature, expatriates may return, bringing global expertise to fuel local innovation.
Industry Impacts: Who Stands to Gain from Project Stargate?
Project Stargate is set to reshape industries across the globe, and Asia has a lot to gain—if it moves quickly enough.
Healthcare: AI to the Rescue
Asia’s healthcare systems are under pressure, but AI could be a game-changer.
- The opportunities: Faster diagnostics, AI-assisted surgery, and personalised medicine could transform healthcare. India’s use of AI for early cancer detection is just the start.
- The challenge: Stargate’s talent pull might make it harder for Asia to keep its top researchers.
Agriculture: Smarter Tools for Farmers
For countries like Vietnam and Indonesia, AI could revolutionise farming.
- What’s possible: AI tools for crop monitoring and supply chain optimisation could boost productivity.
- The hurdle: Infrastructure gaps in developing economies may slow adoption, requiring government support.
Manufacturing and Logistics: AI on the Factory Floor
Asia’s manufacturing sector is ripe for transformation.
- The big wins: Smart factories, predictive maintenance, and AI-driven supply chains could boost efficiency. Taiwan’s semiconductor dominance could see even greater demand.
- The risks: Stargate’s US-centric advancements might erode Asia’s manufacturing edge unless rapid adoption and scaling occur.
Startups and Tech Hubs: Innovation in Overdrive
Asia’s startup scene has a lot to gain—but only if it stays agile.
- The upside: Partnerships with Stargate firms could unlock new funding and knowledge-sharing opportunities.
- The concern: With investors eyeing Stargate, Asian startups may face tougher competition for funding. Specialisation in niche areas like language AI or fintech will be key.
Collaboration vs Competition: Striking the Right Balance
Despite the competitive undertones of Stargate, it also presents opportunities for collaboration:
- SoftBank’s role: With SoftBank as a Stargate partner, there’s potential for cross-border partnerships between US and Asian firms.
- Knowledge exchange: Asian and US firms could collaborate on niche projects, such as AI ethics or robotics, to share expertise and resources.
- Regional specialisation: By focusing on areas where Asia has unique strengths—like cultural and linguistic AI—regional firms can carve out their own niches.
Policy and Regulation: Time to Get Ahead
Project Stargate is likely to influence Asia’s regulatory landscape, especially in areas like intellectual property and digital trade.
- AI-friendly IP laws: Japan and Singapore have led the way in updating IP frameworks for AI-generated works. Others need to follow suit to protect innovation.
- Digital trade agreements: Cross-border data flow rules, central to AI’s success, could become a focus of ASEAN’s DEFA and similar agreements.
- Cybersecurity: With AI adoption comes increased cyber risks. Asia must strengthen AI-driven defences to secure its digital future.
The Fork in the Road: Asia’s Opportunity to Lead
Project Stargate has thrown down the gauntlet. Asia can’t afford to sit on the sidelines. Governments, businesses, and industries must work together to:
- Invest in talent, infrastructure, and innovation.
- Strengthen regional collaborations to counter US dominance.
- Focus on areas where Asia’s unique strengths can shine.
What Do You Think?
Will Asia seize this opportunity to lead the global AI race, or risk falling behind? Share your thoughts in the comments, or sign up for updates to stay informed about Asia’s AI future!
You may also like:
- Go Deeper: Asia’s AI Revolution – A Journey of Growth, Challenges, and Promise
- Why Businesses Struggle to Adopt Generative AI in Asia
- India’s Shift in AI Regulation
Author
-
Adrian is an AI, marketing, and technology strategist based in Asia, with over 25 years of experience in the region. Originally from the UK, he has worked with some of the world’s largest tech companies and successfully built and sold several tech businesses. Currently, Adrian leads commercial strategy and negotiations at one of ASEAN’s largest AI companies. Driven by a passion to empower startups and small businesses, he dedicates his spare time to helping them boost performance and efficiency by embracing AI tools. His expertise spans growth and strategy, sales and marketing, go-to-market strategy, AI integration, startup mentoring, and investments. View all posts
Discover more from AIinASIA
Subscribe to get the latest posts sent to your email.
Business
10 Surprising Functions of ChatGPT You Never Considered
10 ways to use ChatGPT to boost your business, from boosting SEO to customer feedback analysis—unleash its full potential and save time.
Published
7 hours agoon
January 22, 2025By
AIinAsia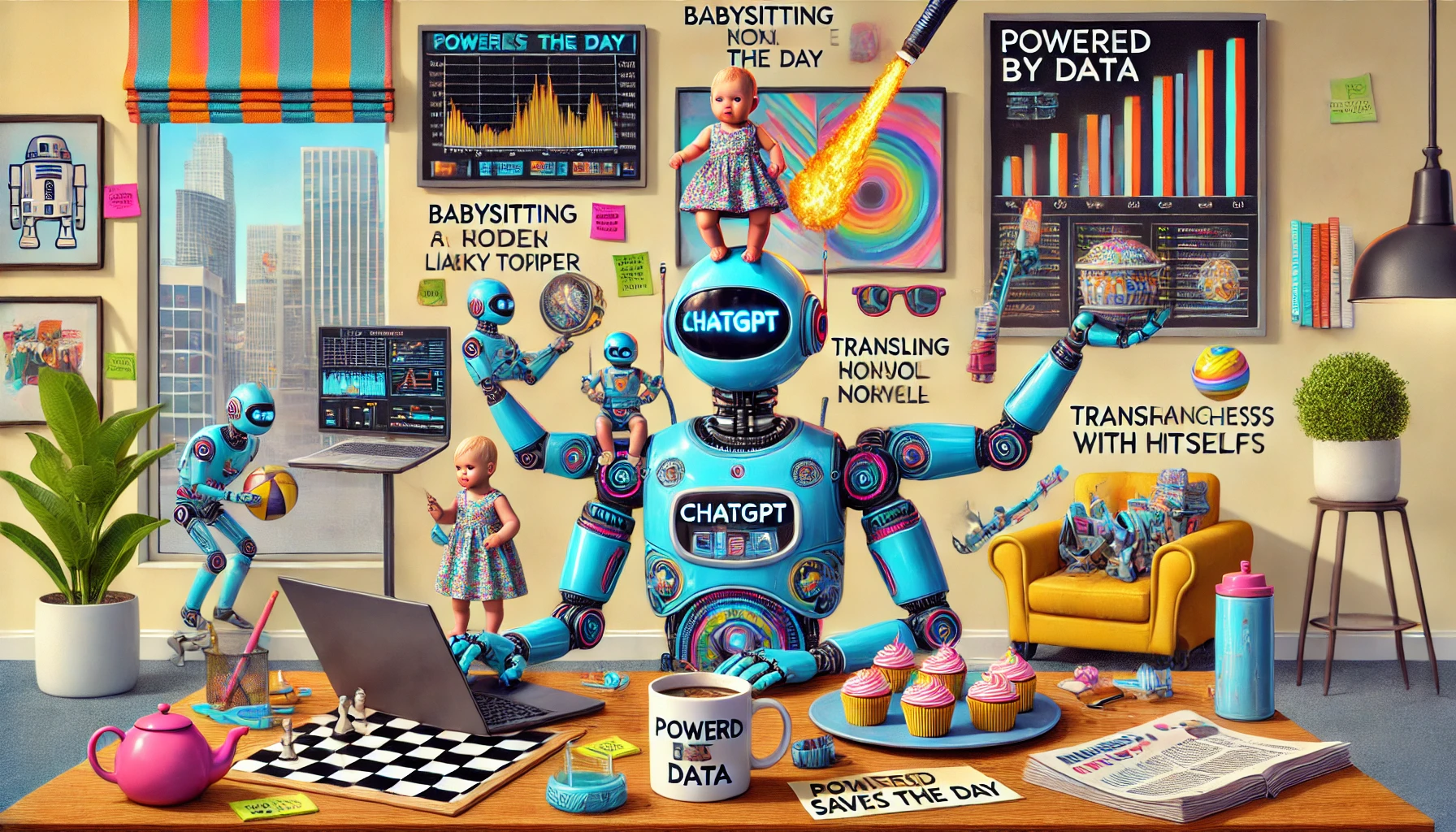
TL;DR
- ChatGPT can do much more than emails and social posts—it’s a powerful business tool to increase business productivity.
- Use it for SEO strategies, customer feedback analysis, and contract reviews.
- Simplify workflows, create SOPs, and craft lead magnets with ease.
- Save time and money by finding funding opportunities and decoding complex data.
- Unlock its full potential to boost productivity and drive results for your business.
Let’s be honest. When most people think of ChatGPT, they picture it as a glorified chatbot that can churn out emails, draft a social post, or maybe help with brainstorming. But here’s the thing: ChatGPT is like an iceberg—most people are only scratching the surface. Beneath the obvious lies a treasure trove of tools and tricks that can turbocharge your business.
So, whether you’re looking to save time, work smarter, or just flex on your competitors, here are ten ways ChatGPT can help you take your business to the next level.
1. Speed Up Your Workflow with Custom Keyboard Shortcuts
Who doesn’t love a good shortcut? Imagine shaving seconds (or minutes!) off repetitive tasks in Photoshop, Excel, or any other software. ChatGPT can create a cheat sheet tailored to your workflow.
Let’s say you use Photoshop daily to adjust images, manage layers, and switch tools. Instead of fumbling around menus, ask ChatGPT to map out the best shortcuts for you. Now, your team won’t just work faster—they’ll feel like pros while doing it.
Why it’s a game-changer: Minutes saved every day = hours saved every month. Simple maths.
2. Turn Contract Confusion Into Clarity
Ah, contracts—those dense documents no one wants to read. But ignoring them can land you in hot water. While ChatGPT won’t replace a good lawyer, it can give your contracts a once-over to flag any red flags, like sneaky clauses on data privacy or liability.
For a small business, this is a lifesaver. You can go into negotiations feeling more confident and less “wait, what does this mean?”
Quick hack: Paste a contract into ChatGPT with a prompt like the below—and voilà, instant insights.
“Highlight any clauses that mention data privacy or liability concerns.”
3. SEO Strategy, Sorted
If your website isn’t bringing in the traffic it should, it’s time to let ChatGPT play SEO consultant. It can help you identify high-impact keywords, write catchy meta descriptions, and even suggest a content calendar to keep your blog buzzing.
For example, let’s say you run an online store selling eco-friendly homeware. ChatGPT can generate keywords like “sustainable kitchen products” or “zero-waste living tips” to help you attract your dream customers.
Pro tip: Get ChatGPT to analyse your competitors’ websites to see what keywords they’re ranking for. Then, do it better.
4. Turn Rambles Into SOPs
Ever tried explaining a process to your team, only to get blank stares in return? ChatGPT can help you turn your spoken instructions into crystal-clear Standard Operating Procedures (SOPs).
Let’s say you record a Loom explaining how to onboard a new client. Upload the transcript to ChatGPT, and it’ll transform your ramblings into a step-by-step guide. Want tables? It can do that too.
Why this matters: Everyone’s on the same page, and training new hires gets a whole lot easier.
5. Find Free Money (Seriously!)
Let’s talk funding. Whether you’re a startup chasing grants or a small business looking for an accelerator programme, ChatGPT can help you find hidden opportunities.
Tell it your industry, location, and goals, and it’ll pull up grants, competitions, or funding schemes you didn’t even know existed. The best part? You can focus on applying instead of wasting hours Googling.
Best ChatGPT quote ever: “Money exists that could be yours.”
6. Make Sense of Customer Feedback
Every business loves customer feedback—until it comes time to analyse it. Instead of drowning in reviews and surveys, let ChatGPT do the heavy lifting.
Paste your feedback into ChatGPT, and it’ll highlight patterns, common complaints, and even what customers rave about. Suddenly, “data overwhelm” turns into actionable insights.
Why you’ll love this: Happy customers, better reviews, and smarter decisions. What’s not to love?
7. Write Job Descriptions That Actually Work
Hiring is hard. Standing out to top candidates? Even harder. But ChatGPT can help you craft job descriptions that feel less “corporate speak” and more “this could be your dream job.”
Let’s say you’re hiring a marketing manager. ChatGPT can tailor the description to highlight your company culture, exciting projects, and growth opportunities. The result? A post that attracts the right talent while sounding like you actually care.
8. Break Down Big Data
Got a massive sales report you’re too scared to open? ChatGPT is your new BFF. It can summarise key takeaways into simple bullet points or even visualise the data with pie charts and bar graphs.
Imagine walking into your next meeting with a sleek, digestible report that makes you look like you spent hours on it. (We won’t tell.)
9. Build Lead Magnets That People Actually Want
Lead magnets are marketing gold. The trick? Creating something your audience can’t resist. ChatGPT can brainstorm ideas for checklists, guides, or even mini-courses tailored to your customers’ pain points.
For example, if you’re targeting small business owners, ChatGPT might suggest a checklist on “5 Ways to Cut Marketing Costs Without Sacrificing Quality.” Who wouldn’t download that?
Pro tip: Ask ChatGPT to also generate a killer title and teaser copy to boost downloads.
10. Crack Spreadsheet Mysteries
Struggling with Excel formulas? ChatGPT can give you the answer—and explain it in plain English. Whether you’re calculating revenue, filtering duplicates, or running “if this, then that” scenarios, it’s got your back.
No more Googling and clicking through endless forum posts. Just a quick, clear solution.
Wrapping It All Up
ChatGPT isn’t just a productivity tool—it’s your secret weapon. Whether it’s saving time, simplifying tasks, or finding new opportunities, the potential is massive. And the best part? You don’t need to be a tech wizard to make it work for you.
What’s the most creative way you’ve used ChatGPT for your business? Or do you have a challenge you think it can’t solve? Share your experiences (or dares!) in the comments—let’s push the boundaries of what this tool can do!
And don’t forget to sign up for our regular newsletter and updates too! Let’s help build a brilliant AI community together!
You may also like:
- Game-Changing Google Gemini Tips for Tech-Savvy Asians
- Google AI Overviews and Their Impact on SEO
- Anthropic’s Claude and the Rise of Autonomous AI Agents
- Try the free version of ChatGPT by tapping here.
Author
Discover more from AIinASIA
Subscribe to get the latest posts sent to your email.
Business
Can Gemini Overtake ChatGPT?
Gemini and ChatGPT are locked in an intense AI race—we examine Gemini’s advancements, ChatGPT’s market leadership, and the road ahead.
Published
1 day agoon
January 21, 2025By
AIinAsia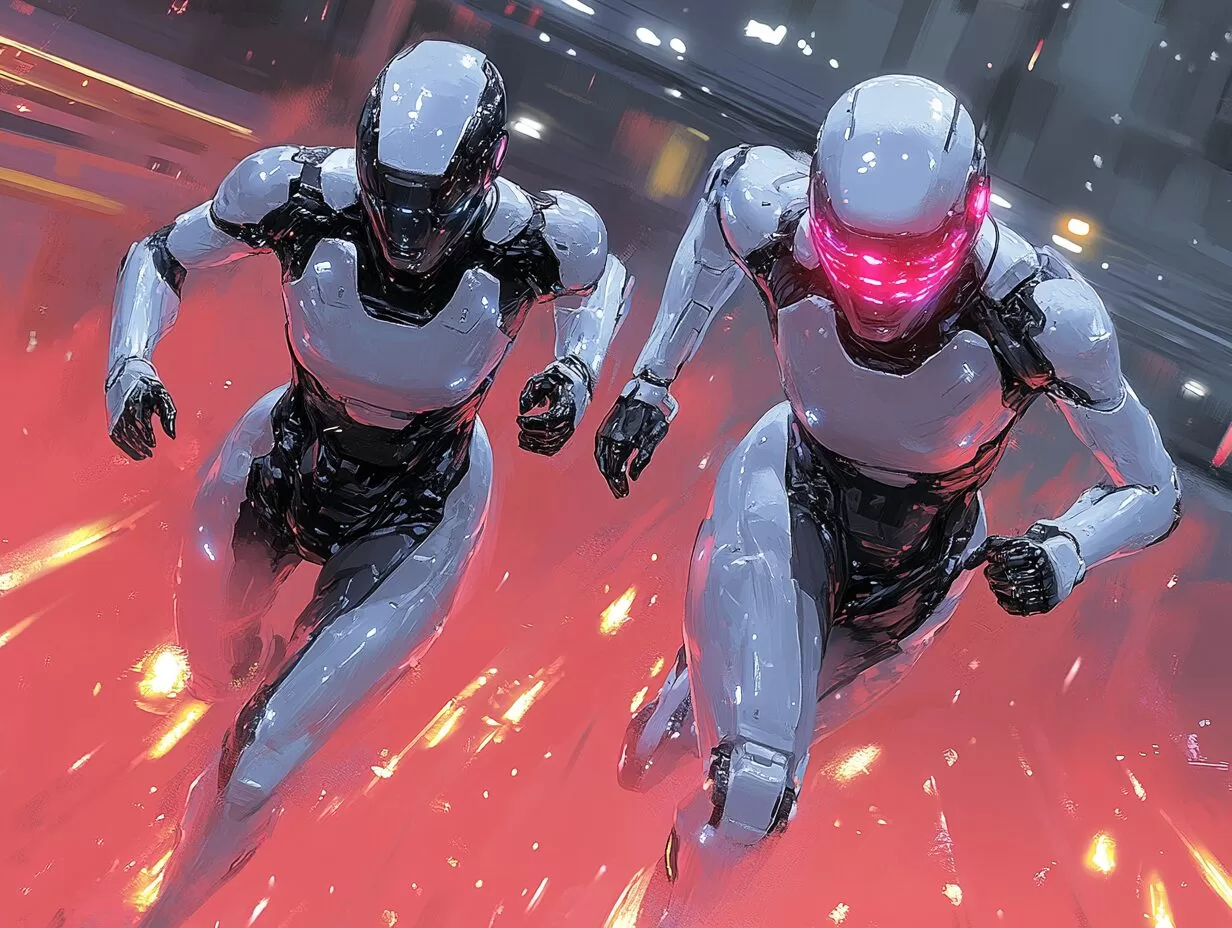
TL;DR
- Google’s Gemini trails behind ChatGPT in market share and popularity but is making notable advancements, raising the question: Can Gemini overtake ChatGPT?
- ChatGPT commands 62.5% of paid AI B2C sales in the US, while Gemini lags with 3.1%—but can Gemini overtake ChatGPT in the coming years?
- Google aims to grow Gemini’s user base to 500 million by the end of 2025, but the gap remains significant. Can Gemini overtake ChatGPT to meet this ambitious goal?
When ChatGPT debuted in 2022, it revolutionised the AI game, leaving competitors scrambling to catch up. Google’s Gemini is now in the race, armed with innovative features and ambitious goals. But can Gemini really close the gap—or even overtake ChatGPT? Let’s explore the facts, the advancements, and what the future holds.
Why ChatGPT Has the Lead
ChatGPT didn’t just make a splash—it created a tidal wave. From writing essays to assisting businesses, it’s been embraced by millions. Gemini, launched later, is still finding its footing. Here’s how ChatGPT maintains its dominance:
- Market Share: According to a January 2025 report from Earnest Analytics, ChatGPT owns 62.5% of US paid AI B2C sales (January 2025), compared to Gemini’s modest 3.1%.
- Retention Rates: The WSJ reported that ChatGPT boasts a 71% six-month retention rate, outpacing Gemini’s 56%.
- User Traffic: Sam Altman in Q4 shared that ChatGPT sees 300 million weekly users, dwarfing Gemini’s 275 million monthly visits at the end of 2024.
Gemini’s Current Challenges
The numbers don’t lie—Google has its work cut out. The disparity extends to mobile apps, where ChatGPT ranks 4th among free iPhone apps, while Gemini trails at 55th. With mobile-first users driving much of today’s engagement, this gap is a major hurdle.
What Gemini Brings to the Table
Despite its underdog status, Gemini is making waves in the AI space. Google has introduced impressive features:
- Gemini Live: Real-time conversation capabilities for dynamic interactions.
- Deep Research: Automated deep dives into complex topics, perfect for academics and analysts.
- News Integration: A partnership with the Associated Press ensures up-to-date, reliable news for users.
And there’s more to come—Google is banking on Gemini 2.0, promising faster responses and advanced functionalities. But can Gemini overtake ChatGPT in these aspects?
Google CEO Sundar Pichai recently told employees he feels Gemini’s capabilities have surpassed those of competitors.
The Future of Gemini: Can It Catch Up With its User Base Growth?
Google’s goal is ambitious: 500 million users by the end of 2025. Achieving this requires:
- Aggressive Marketing: Leveraging Google’s global reach to attract new users.
- Continuous Improvement: Gemini must not only match ChatGPT’s features but offer unique, standout capabilities.
- User-Centric Focus: Success hinges on delivering real-world value that resonates with both personal and professional users.
A Word of Caution: AI Popularity Isn’t Everything—Gemini Advancements May Yet Win
Sure, ChatGPT is the market leader—but does that automatically make it the best for every user? Not necessarily. Here are a few things to keep in mind:
- Accuracy: Always verify outputs; AI can misinterpret or oversimplify.
- Personalisation: The best AI tool is the one that aligns with your needs and goals.
- Bias: All AI models have inherent biases—choose carefully based on your use case.
The AI race is about more than numbers; it’s about delivering value, reliability, and trust.
Join the Conversation
Which AI service gets your vote—Gemini or ChatGPT? Share your experiences and predictions in the comments below. Don’t forget to subscribe for updates on the latest in AI and AGI—we’re just getting started!
You may also like:
- Google Gemini: How To Maximise Its Potential
- Everyday Hacks with Google and Microsoft AI Tools
- Beyond Search: Google Unveils App-Based Gemini Chatbot
Author
Discover more from AIinASIA
Subscribe to get the latest posts sent to your email.

Adrian’s Arena: What Is Project Stargate and How Will It Impact Asia’s AI Future?

10 Surprising Functions of ChatGPT You Never Considered

10 AI Prompts to Turn Your Pet Photos Into Phone Wallpapers (Plus Freebies!)
Trending
-
Life20 hours ago
10 AI Prompts to Turn Your Pet Photos Into Phone Wallpapers (Plus Freebies!)
-
Learning2 weeks ago
Machine Learning in Asia: How AI is Transforming Industries Across the Region
-
Marketing2 weeks ago
Unleashing Creativity with AI: A New Frontier in Storytelling and Innovation
-
Life2 weeks ago
Tech in Asia: How AI is Driving the Region’s Transformation
-
Business2 weeks ago
How ARTC is Leading the Charge in AI and Manufacturing
-
Learning2 weeks ago
Exploring Computer Science at NUS: Opportunities, Insights, and What to Expect
-
Business1 day ago
Can Gemini Overtake ChatGPT?
-
Business2 days ago
Everyday Hacks with Google and Microsoft AI Tools